Deep learning based multi-category object detection in aerial images
Proceedings of SPIE(2017)
摘要
Multi-category object detection in aerial images is an important task for many applications such as surveillance, tracking or search and rescue tasks. In recent years, deep learning approaches using features extracted by convolutional neural networks (CNN) significantly improved the detection accuracy on detection benchmark datasets compared to traditional approaches based on hand-crafted features as used for object detection in aerial images. However, these approaches are not transferable one to one on aerial images as the used network architectures have an insufficient resolution of feature maps for handling small instances. This consequently results in poor localization accuracy or missed detections as the network architectures are explored and optimized for datasets that considerably differ from aerial images in particular in object size and image fraction occupied by an object. In this work, we propose a deep neural network derived from the Faster R-CNN approach for multicategory object detection in aerial images. We show how the detection accuracy can be improved by replacing the network architecture by an architecture especially designed for handling small object sizes. Furthermore, we investigate the impact of different parameters of the detection framework on the detection accuracy for small objects. Finally, we demonstrate the suitability of our network for object detection in aerial images by comparing our network to traditional baseline approaches and deep learning based approaches on the publicly available DLR 3K Munich Vehicle Aerial Image Dataset that comprises multiple object classes such as car, van, truck, bus and camper.
更多查看译文
关键词
deep learning,multi-category object detection,aerial imagery
AI 理解论文
溯源树
样例
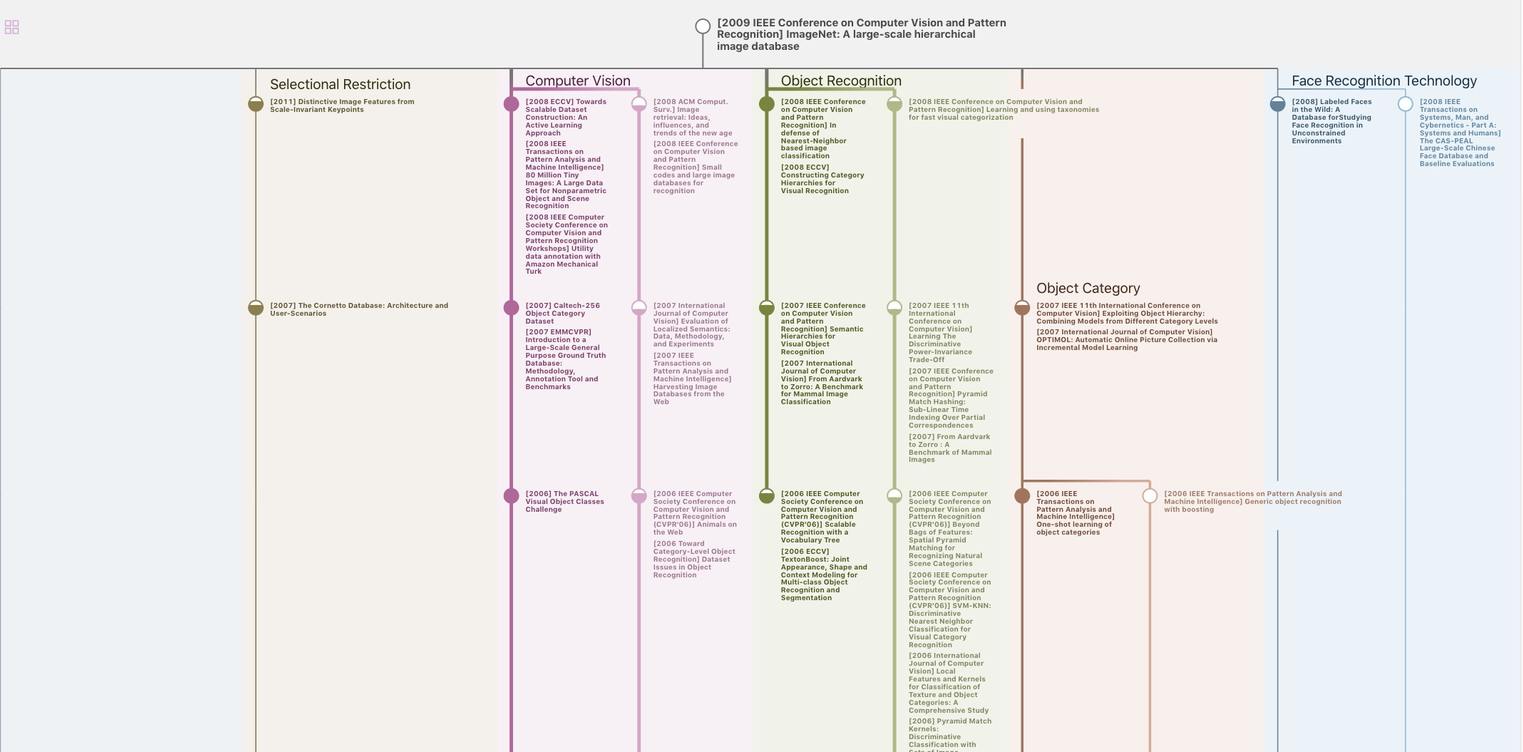
生成溯源树,研究论文发展脉络
Chat Paper
正在生成论文摘要