Poor textural image tie point matching via graph theory
ISPRS Journal of Photogrammetry and Remote Sensing(2017)
摘要
Feature matching aims to find corresponding points to serve as tie points between images. Robust matching is still a challenging task when input images are characterized by low contrast or contain repetitive patterns, occlusions, or homogeneous textures. In this paper, a novel feature matching algorithm based on graph theory is proposed. This algorithm integrates both geometric and radiometric constraints into an edge-weighted (EW) affinity tensor. Tie points are then obtained by high-order graph matching. Four pairs of poor textural images covering forests, deserts, bare lands, and urban areas are tested. For comparison, three state-of-the-art matching techniques, namely, scale-invariant feature transform (SIFT), speeded up robust features (SURF), and features from accelerated segment test (FAST), are also used. The experimental results show that the matching recall obtained by SIFT, SURF, and FAST varies from 0 to 35% in different types of poor textures. However, through the integration of both geometry and radiometry and the EW strategy, the recall obtained by the proposed algorithm is better than 50% in all four image pairs. The better matching recall improves the number of correct matches, dispersion, and positional accuracy.
更多查看译文
关键词
Tie point matching,Poor textural image,Affinity tensor,Edge-weighted,High-order graph matching
AI 理解论文
溯源树
样例
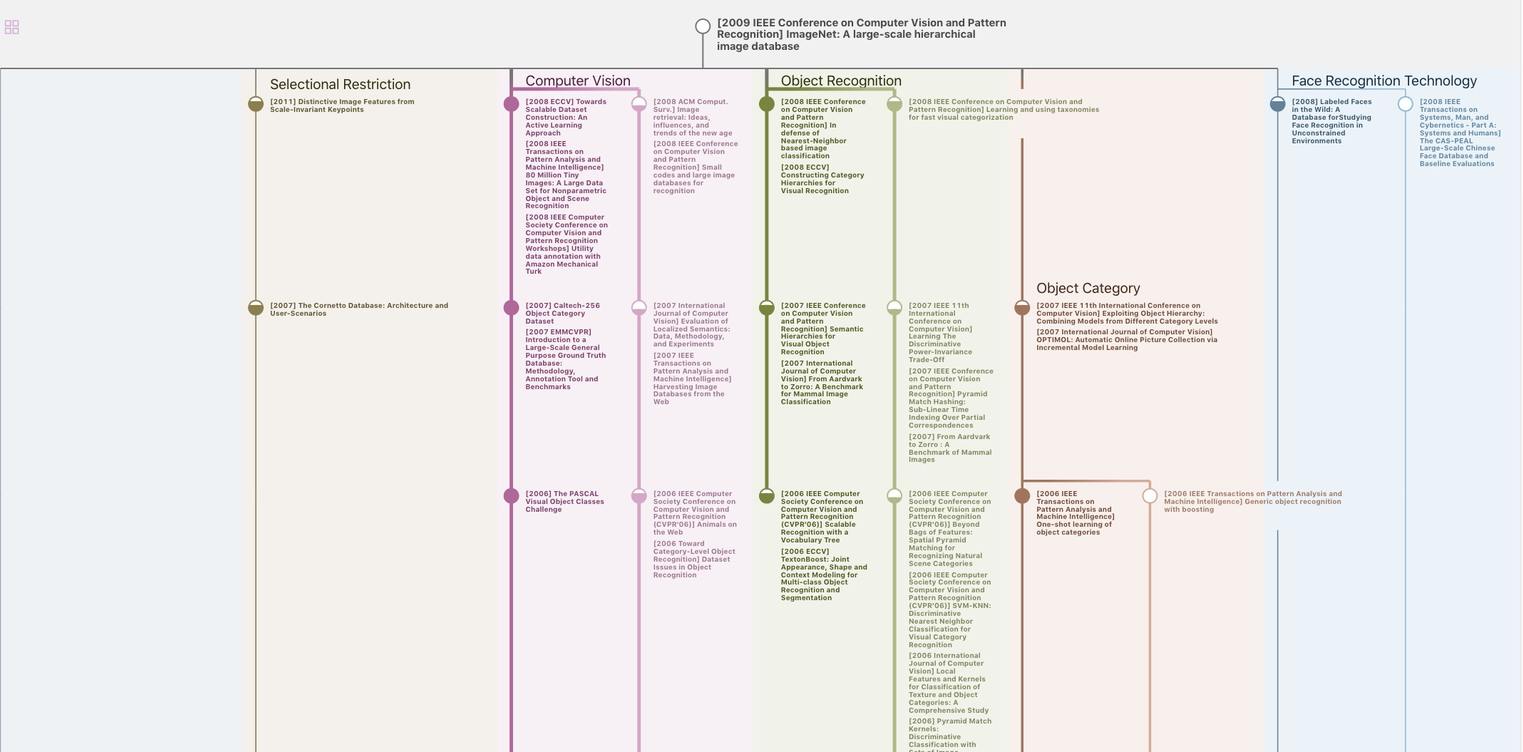
生成溯源树,研究论文发展脉络
Chat Paper
正在生成论文摘要