Improving Detection of Call Clusters through Surveillance of Poison Center Data
CLINICAL TOXICOLOGY(2017)
摘要
Objective Our objective was to compare the effectiveness of applying the historical limits method (HLM) to poison center (PC) call volumes with vs without stratifying by exposure type. Introduction The Centers for Disease Control and Prevention (CDC) uses the National Poison Data System (NPDS) to conduct surveillance of calls to United States PCs. PCs provide triage and treatment advice for hazardous exposures through a free national hotline. Information on demographics, health effects, implicated substance(s), medical outcome of the patient, and other variables are collected. CDC uses automated algorithms to identify anomalies in both pure call volume and specific clinical effect volume, and to identify calls reporting exposure to high priority agents. Pure and clinical effect volume anomalies are identified when an hourly call count exceeds a threshold based on historical data using HLM. 1 Clinical toxicologists and epidemiologists at the American Association of Poison Control Centers and CDC apply standardized criteria to determine if the anomaly identifies a potential incident of public health significance (IPHS) and to notify the respective health departments and local PCs as needed. Discussions with NPDS users and analysis of IPHS showed that alerting based on pure call volume yielded excessive false positives. A study using a 5-year NPDS call dataset assessed the positive predictive value (PPV) of the call volume-based approach. This study showed that less than 4% of anomalies were IPHS. 2 A low PPV can cause unnecessary waste of staff time and resources analyzing false positive anomalies. As an alternative to pure call volume-based detection where all calls to each PC are aggregated for anomaly detection, we considered separating calls by toxicologically-relevant exposure categories for more targeted anomaly detection. We hypothesized that this stratified approach would reduce the number of false positives. Methods We derived our exposure categories based on the criteria that the categories must: 1) relate to hazardous exposures of public health importance, 2) reflect categories based on clinical effects and treatment modalities, 3) avoid high priority exposures that may be triggered by single calls, 4) be compatible with exposure substance identification codes currently used by PCs and NPDS, and 5) include enough calls for meaningful tracking. We queried all calls reporting exposures to the proposed categories between January 1, 2009 and July 31, 2015 for ten PCs. We applied the HLM method after stratifying by exposure category and tabulated the number of alerts triggered for each category during the study period. We then applied the HLM method for the ten PCs on all combined exposure calls to represent the traditional non-stratified approach. We compared the combined alert burden generated by stratifying by exposure category with the alert burden for the non-stratified approach for varying time windows (1-, 2-, 4-, 8- and 24-hours). We conducted analysis in R. Results We derived a total of 20 exposure categories, including chemicals (n=4), drugs of abuse (n=6), pesticides (n=3), gas/fume/vapors (n=2), contaminated food/water (n=1), and others (n=4). Call counts during 2015 for these categories ranged from approximately 5,000 to 90,000. Table 1 shows the total number of alerts triggered for each method by time windows. There was a marked reduction of alert burden when first stratifying by exposure category for time windows shorter than eight hours compared to the alert burden for the non-stratified approach. Conclusions Stratification of call volume by exposure category and time window suggests potential improvement over traditional non- stratified approach by having a lower alert burden. Further work should focus on refining the exposure categories, refining the time window for surveillance, and assessing other detection performance metrics, such as sensitivity. Table 1: Alert burden comparison for the non-stratified vs stratified approach
更多查看译文
关键词
Public health,surveillance,poison center
AI 理解论文
溯源树
样例
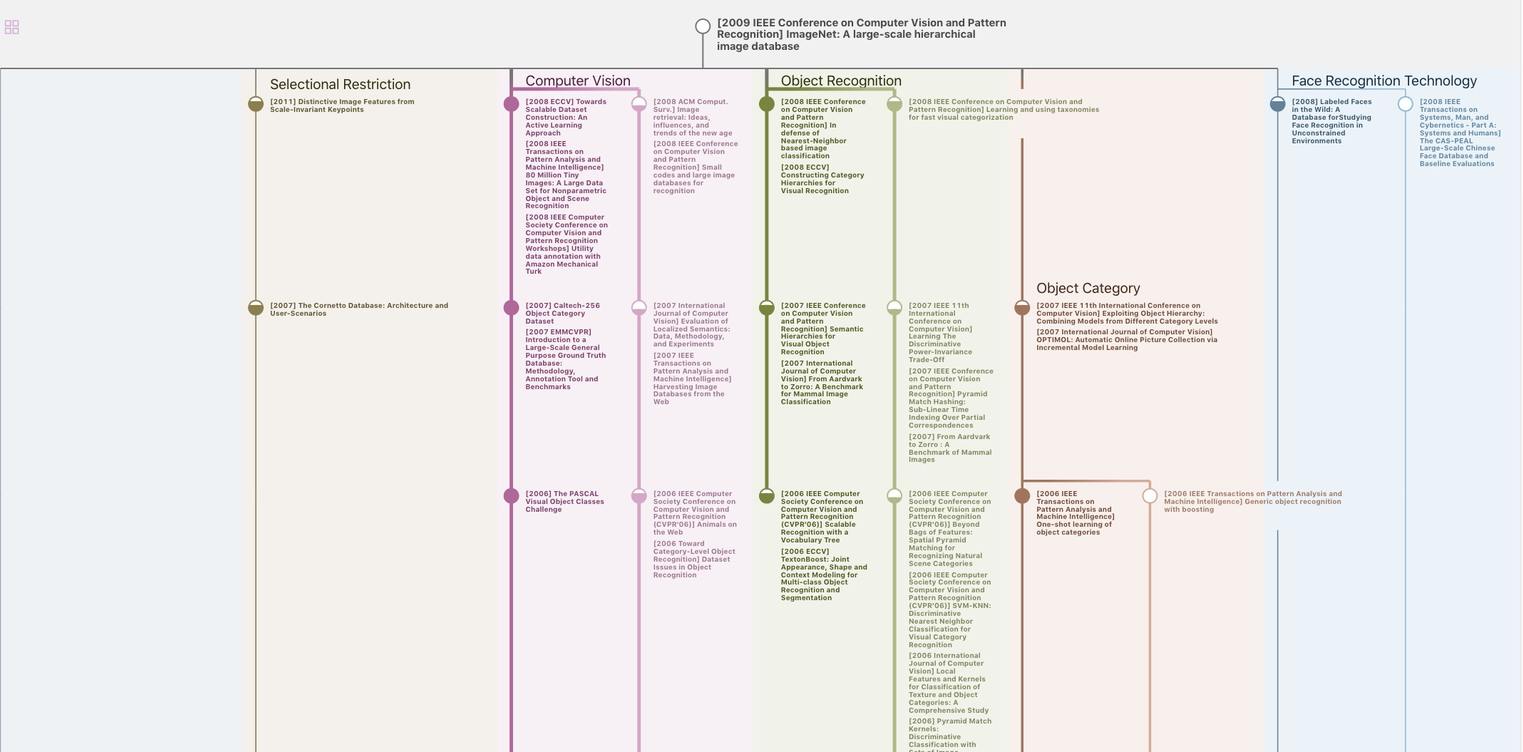
生成溯源树,研究论文发展脉络
Chat Paper
正在生成论文摘要