Convolutional Networks For Voting-Based Anomaly Classification In Metal Surface Inspection
2017 IEEE INTERNATIONAL CONFERENCE ON INDUSTRIAL TECHNOLOGY (ICIT)(2017)
摘要
Automated Visual Inspection (AVI) systems for metal surface inspection is increasingly used in industries to aid human visual inspectors for classification of possible anomalies. For classification, the challenge lies in having a small and specific dataset that may easily result in over-fitting. As a solution, we propose to use deep Convolutional Neural Networks (ConvNets) learnt from the large ImageNet dataset [9] for image representations via transfer learning. Since a small dataset cannot be used to fine-tune a ConvNet due to overfitting, we also propose a Majority Voting Mechanism (MVM), which fuses the features extracted from the last three layers of ConvNets using Support Vector Machine (SVM) classifiers. This classification framework is effective where no prior knowledge of the best performing ConvNet layers is needed. This also allows flexibility in the choice of ConvNet used for feature extraction. The proposed method not only outperforms state-of-the-art traditional hand-crafted features in terms of classification but also obtains good results compared to other deep ConvNet features extracted from a pre-selected best layer on several anomaly and texture datasets.
更多查看译文
关键词
anomaly classification,convolutional neural network,transfer learning,voting,automated visual inspection,metal surface
AI 理解论文
溯源树
样例
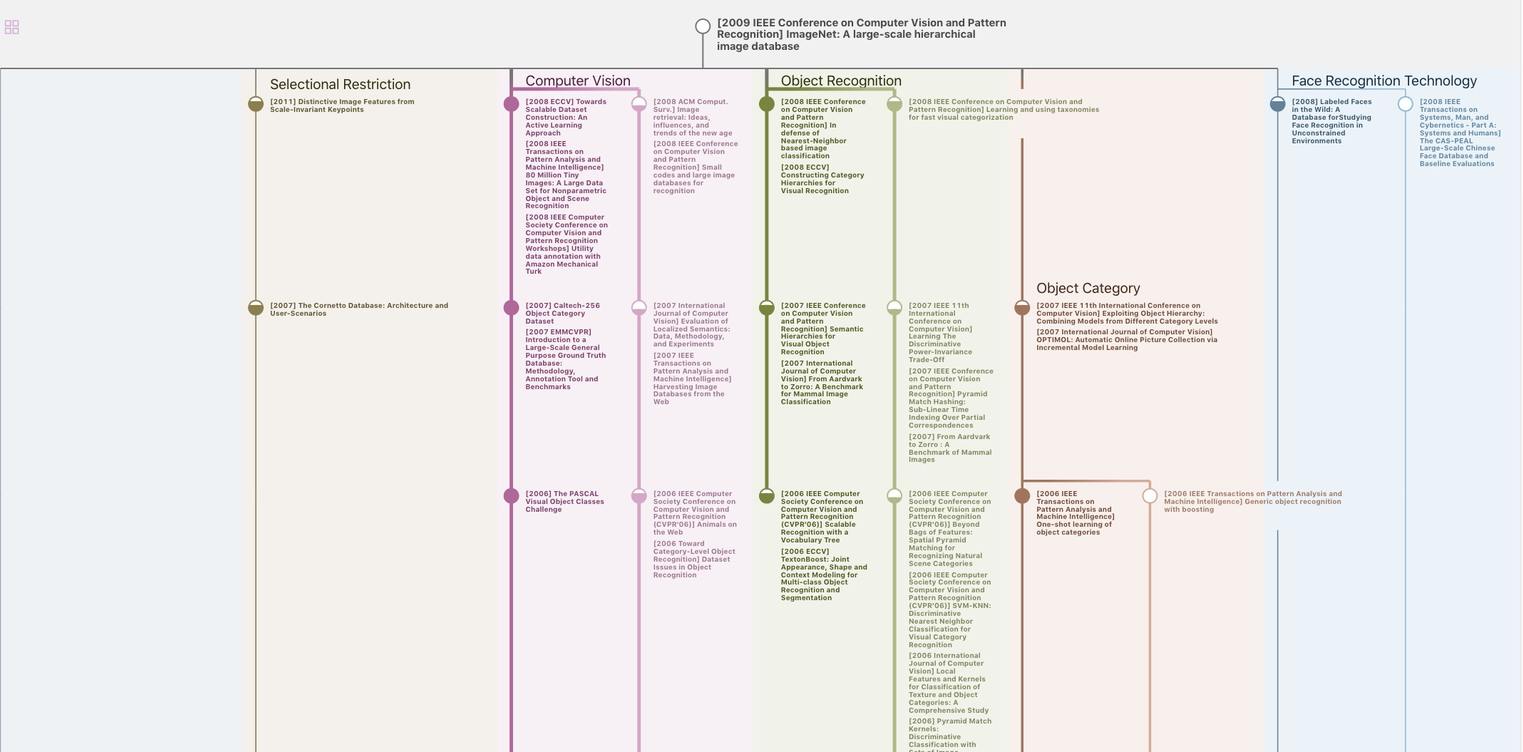
生成溯源树,研究论文发展脉络
Chat Paper
正在生成论文摘要