The Algorithmic Neuroanatomy Of Action-Outcome Learning
bioRxiv(2017)
摘要
Although it is well known that animals can encode the consequences of their actions and can use this information to control action selection and evaluation, it is not known what learning rules control action-outcome (AO) learning. Here we trained participants to encode specific AO associations whilst undergoing functional imaging (fMRI) and used computational modelling to evaluate competing models. This analysis revealed that a Kalman filter, which learned the unique causal effect of each action, best characterized AO learning and found the medial prefrontal cortex differentiated the unique effect of actions from background effects. We subsequently extended these findings to show that mPFC participated in a circuit with parietal cortex and caudate nucleus to segregate distinct contributions to AO learning. The results extend our understanding of goal-directed learning and demonstrate that sensitivity to the causal relationship between actions and outcomes guides goal-directed learning rather than contiguous state-action relations.
更多查看译文
关键词
reinforcement learning,causal,computational neuroscience,fMRI
AI 理解论文
溯源树
样例
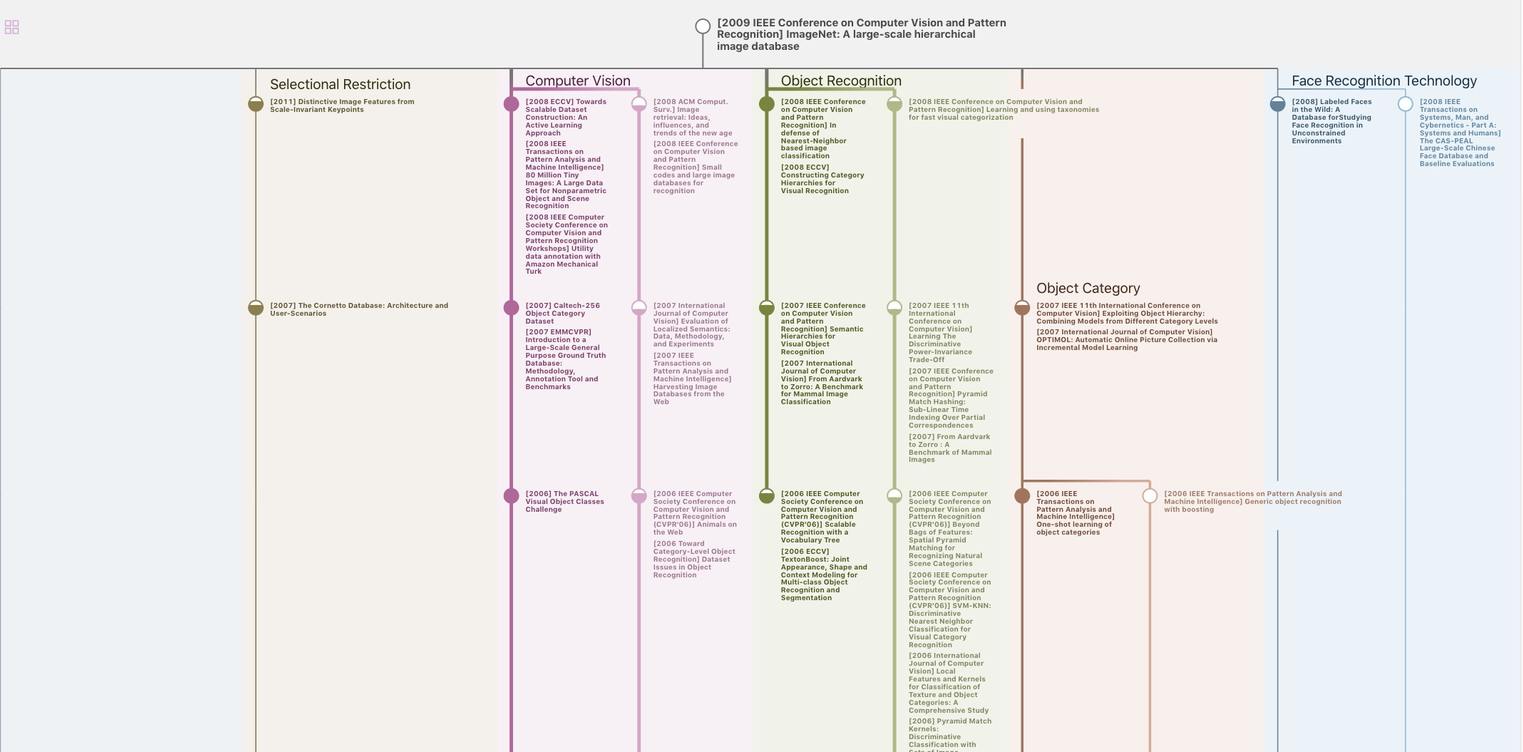
生成溯源树,研究论文发展脉络
Chat Paper
正在生成论文摘要