Unsupervised Source Selection for Domain Adaptation
Photogrammetric engineering and remote sensing(2018)
摘要
The creation of training sets for supervised machine learning often incurs unsustainable manual costs. Transfer learning (TL) techniques have been proposed as a way to solve this issue by adapting training data from different, but related (source) datasets to the test (target) dataset. A problem in TL is how to quantify the relatedness of a source quickly and robustly. In this work, we present a fast domain similarity measure that captures the relatedness between datasets purely based on unlabeled data. Our method transfers knowledge from multiple sources by generating a weighted combination of domains. We show for multiple datasets that learning on such sources achieves an average overall accuracy closer than 2.5 percent to the results of the target classifier for semantic segmentation tasks. We further apply our method to the task of choosing informative patches from unlabeled datasets. Only labeling these patches enables a reduction in manual work of up to 85 percent.
更多查看译文
关键词
Transfer Learning,Unsupervised Learning,Semi-Supervised Learning,Meta-Learning,Optimization
AI 理解论文
溯源树
样例
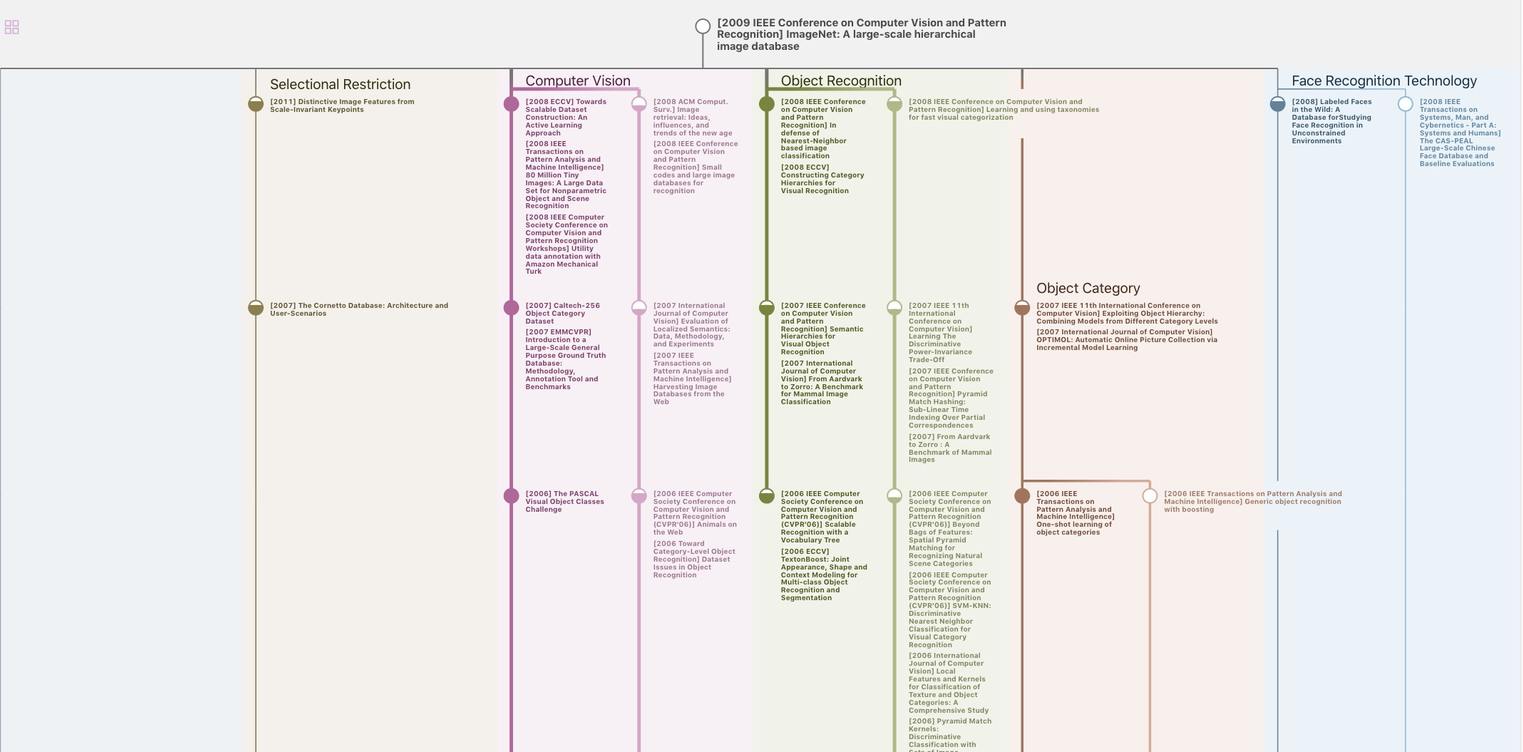
生成溯源树,研究论文发展脉络
Chat Paper
正在生成论文摘要