Neuromorphic Technologies for Next-Generation Cognitive Computing
2017 IEEE International Memory Workshop (IMW)(2017)
摘要
With the end of Dennard scaling and the consequent slow-down of Moore's law, researchers are looking to exploit new device, circuit and architectural concepts to build future systems that are exponentially more capable than the systems of today. One promising approach is Neuromorphic computing based on Non-Von Neumann architectures, that could achieve orders of magnitude performance improvements over conventional systems on certain neural network training tasks. In this paper we review our recent work on implementing such a neuromorphic system on arrays of Phase- Change-Memory (PCM) devices. We present both experimental and simulation results that explore the impact of device non-idealities such as non-linearity, asymmetry, drift and variability on neural network performance. We also highlight circuit design approaches to enable speedup vs. conventional systems.
更多查看译文
关键词
circuit design approaches,device nonidealities,PCM devices,phase-change-memory devices,neuromorphic system,neural network training tasks,nonVon Neumann architectures,neuromorphic computing,future systems,next-generation cognitive computing,neuromorphic technologies
AI 理解论文
溯源树
样例
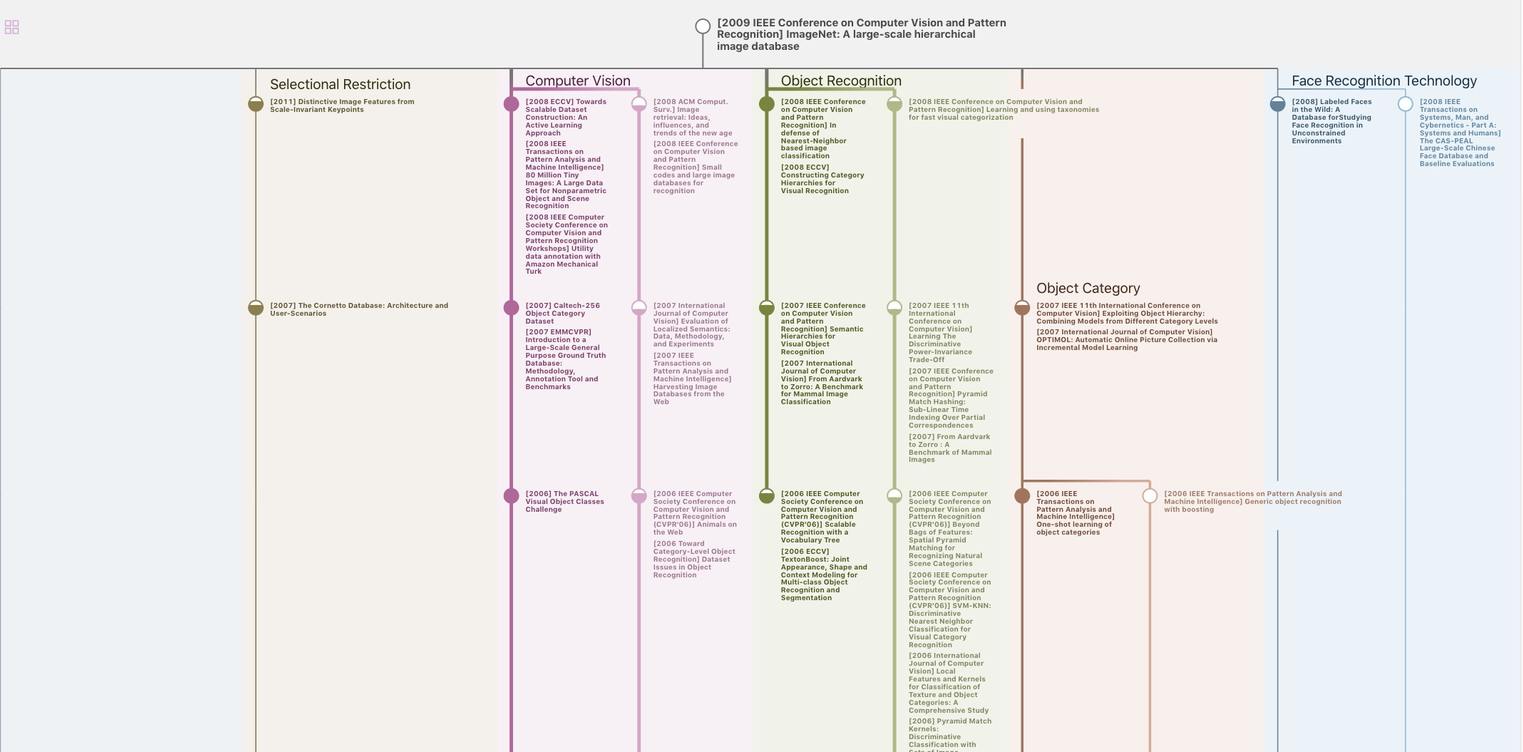
生成溯源树,研究论文发展脉络
Chat Paper
正在生成论文摘要