Spatiotemporal predictions of soil properties and states in variably saturated landscapes
JOURNAL OF GEOPHYSICAL RESEARCH-BIOGEOSCIENCES(2017)
摘要
Understanding greenhouse gas (GHG) fluxes from landscapes with variably saturated soil conditions is challenging given the highly dynamic nature of GHG fluxes in both space and time, dubbed hot spots, and hot moments. On one hand, our ability to directly monitor these processes is limited by sparse in situ and surface chamber observational networks. On the other hand, remote sensing approaches provide spatial data sets but are limited by infrequent imaging over time. We use a robust statistical framework to merge sparse sensor network observations with reconnaissance style hydrogeophysical mapping at a well-characterized site in Ohio. We find that combining time-lapse electromagnetic induction surveys with empirical orthogonal functions provides additional environmental covariates related to soil properties and states at high spatial resolutions (similar to 5m). A cross-validation experiment using eight different spatial interpolation methods versus 120 in situ soil cores indicated an similar to 30% reduction in root-mean-square error for soil properties (clay weight percent and total soil carbon weight percent) using hydrogeophysical derived environmental covariates with regression kriging. In addition, the hydrogeophysical derived environmental covariates were found to be good predictors of soil states (soil temperature, soil water content, and soil oxygen). The presented framework allows for temporal gap filling of individual sensor data sets as well as provides flexible geometric interpolation to complex areas/volumes. We anticipate that the framework, with its flexible temporal and spatial monitoring options, will be useful in designing future monitoring networks as well as support the next generation of hyper-resolution hydrologic and biogeochemical models. Plain Language Summary Understanding greenhouse gas emissions from landscapes with variable saturated soil conditions is challenging given the highly dynamic nature of greenhouse gases (GHGs) in both space and time, dubbed hot spots, and hot moments. On one hand, our ability to directly monitor these processes is limited by sparse in situ and surface observational networks. On the other hand, proximal and remote sensing approaches provide spatial data sets but are limited by indirect observations and infrequent mapping. In this work, we use a statistical framework to merge sparse sensor network observations with reconnaissance mapping. The framework addresses a critical gap that exists in many long-term monitoring networks, specifically how to combine sparse in situ monitoring networks with proximal sensing. We found that combining time-lapse electromagnetic induction surveys with landscape features was able to generate statistical models of (1) soil inventories (i.e. clay percent, total soil carbon, and soil nitrogen) across the study site and (2) spatiotemporal models of key abiotic factors (i.e. soil temperature, soil water content, and soil oxygen) that may affect GHGs. The developed framework allows for temporal gap filling of individual sensors as well provides flexible geometric interpolation making it well suited for the use in the next generation of hydrologic and biogeochemical models.
更多查看译文
关键词
hydrogeophysics,empirical orthogonal functions,scaling,distributed sensors
AI 理解论文
溯源树
样例
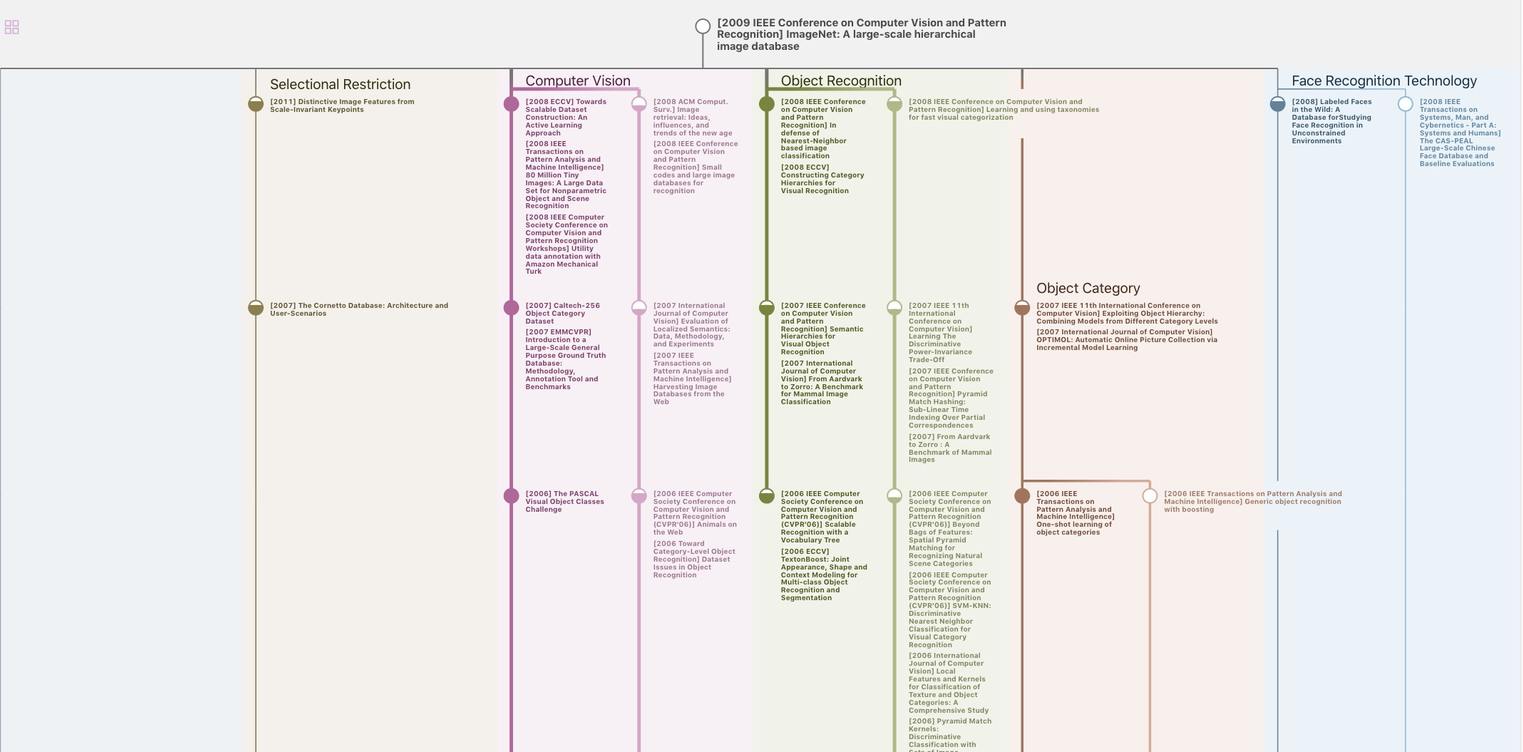
生成溯源树,研究论文发展脉络
Chat Paper
正在生成论文摘要