Active learning framework of informative p53 cancer rescue mutants
2017 Third International Conference on Advances in Electrical, Electronics, Information, Communication and Bio-Informatics (AEEICB)(2017)
摘要
P53 mutants are closely related to humor tumors. Unfortunately, in vitro testing of all possible mutation combinations to determine their cancer rescue effects is infeasible due to time and expense. Therefore, it would be very desirable to have a computer model to run in silico experiments. In this paper, we propose a framework for active learning that can be used in any membership model active learning which does not consider predicted class as a criterion. Because the number of positive instances is much more than the number of negative instances. An active learning strategy is proposed to dynamically balance the number of positive and negative instances. As a result, we get a relatively balanced training set relative to both positive and negative instances, which leads to results showing good performance relative to high precision as well as high recall.
更多查看译文
关键词
dynamically balance,machine learning,negative instances,P53,positive instances
AI 理解论文
溯源树
样例
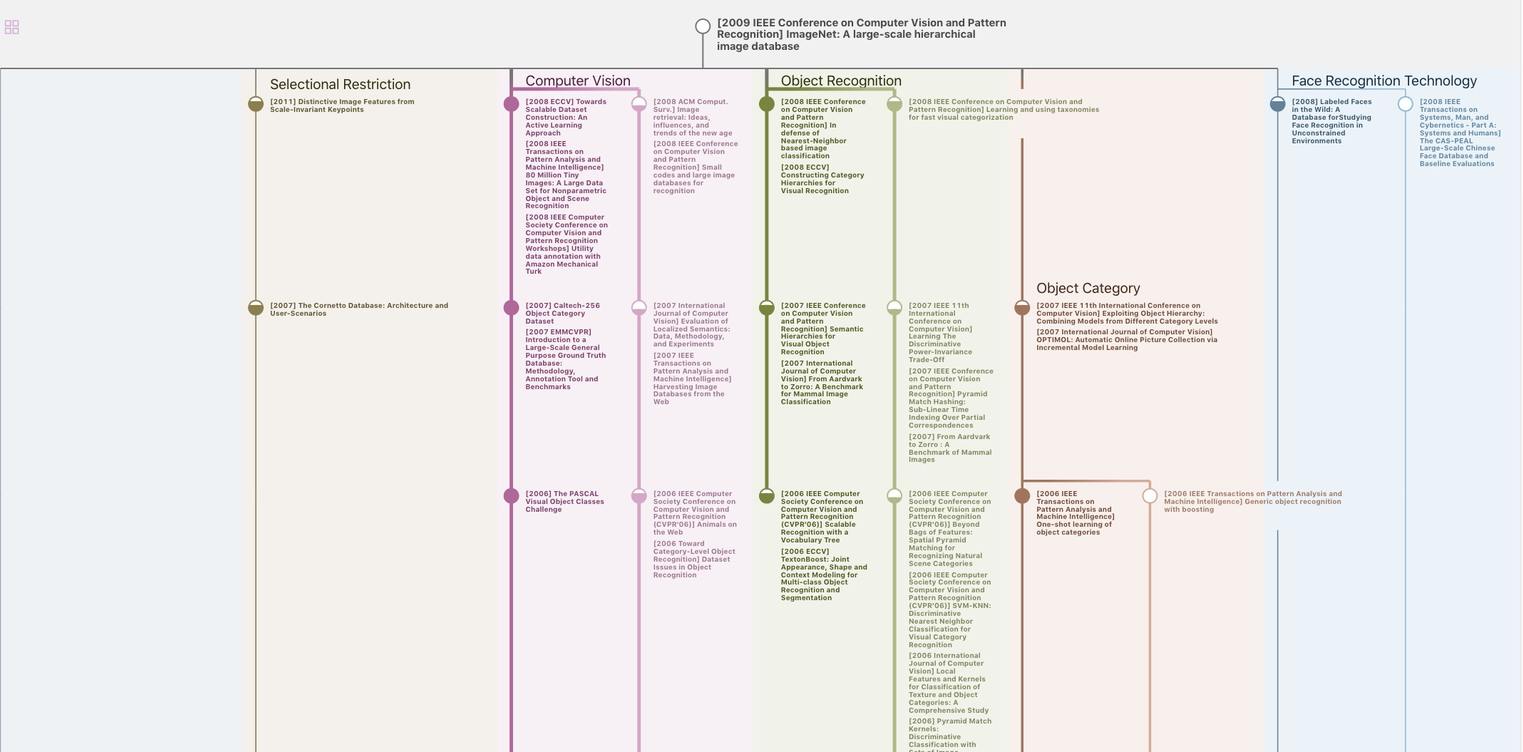
生成溯源树,研究论文发展脉络
Chat Paper
正在生成论文摘要