A weighted Gaussian process regression for multivariate modelling
2017 6th International Symposium on Advanced Control of Industrial Processes (AdCONIP)(2017)
摘要
This paper develops three weighted Gaussian process regression (GPR) approaches for multivariate modelling. Taking into account weighted strategy in the traditional univariate GPR, the heteroscedastic noise problem has been solved. The present paper extends the univariate weighted GPR algorithm to the multivariate case. Considering the correlation and weight between data, as well as the correlation between outputs, the covariance functions of the proposed approaches are formulated. By formulating different process noise mechanisms, the proposed methods can solve different multivariate modelling problems. The effectiveness of the proposed algorithm is demonstrated by a numerical example as well as a six-level drawing of a Carbon fiber example.
更多查看译文
关键词
weighted Gaussian process regression,multivariate modelling,GPR approaches,heteroscedastic noise problem,univariate weighted GPR algorithm,covariance functions,process noise mechanisms,industrial processes
AI 理解论文
溯源树
样例
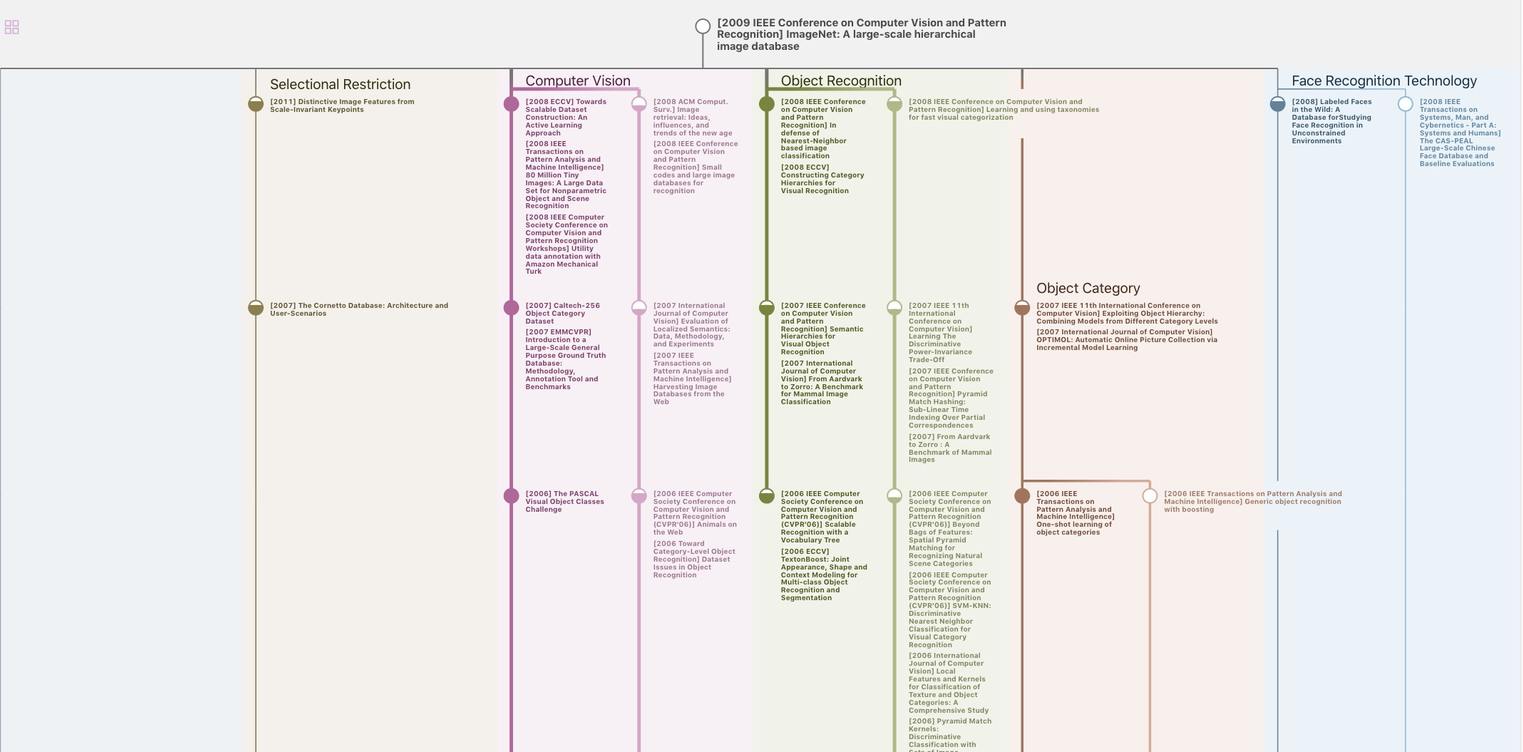
生成溯源树,研究论文发展脉络
Chat Paper
正在生成论文摘要