Integrating Source-Side Semantic Roles Into A Phrase-Based Statistical Machine Translation
2017 25TH IRANIAN CONFERENCE ON ELECTRICAL ENGINEERING (ICEE)(2017)
摘要
Most of the statistical machine translation (SMT) systems suffer from lack of proper understanding about the meaning of input sentence, confusion of semantic roles or semantic ambiguities. In this paper, we propose semantic features for phrase-based SMT (PB-SMT) using source-side semantic roles. The objective of proposed features is to employ predicate-argument information in an English-Persian SMT, which is a sample of low-resource language pairs. Then these features serve to re-rank list of n-best translations produced by the translation system. The first proposed feature tries to preserve the correlation between predicate-argument structures (PAS) among two languages, while the second one aims to preserve the internal cohesion of semantic roles. Both of these features use direct annotation projection to transfer semantic roles from the source to the target language. For extracting the last feature, the Persian Semantic Role Labeling (SRL) is used which is trained on Persian semantic annotated data. We create the training data by automatically projecting the annotation from English to Persian using in-house parallel data, filtering out uncertain labels and also by bootstrapping. To our knowledge, this is the largest Persian corpus annotated with the semantic roles. Evaluations show that these features cause an improvement of 0.65 BLEU score over the baseline. Human evaluation of the translation results also shows the positive influence of these features on translation quality. Preliminary results from experiments demonstrate the importance of predicate-argument semantics in machine translation.
更多查看译文
关键词
Statistical machine translation, Predicate-argument structure, Semantic role labeling, N-best re-ranking
AI 理解论文
溯源树
样例
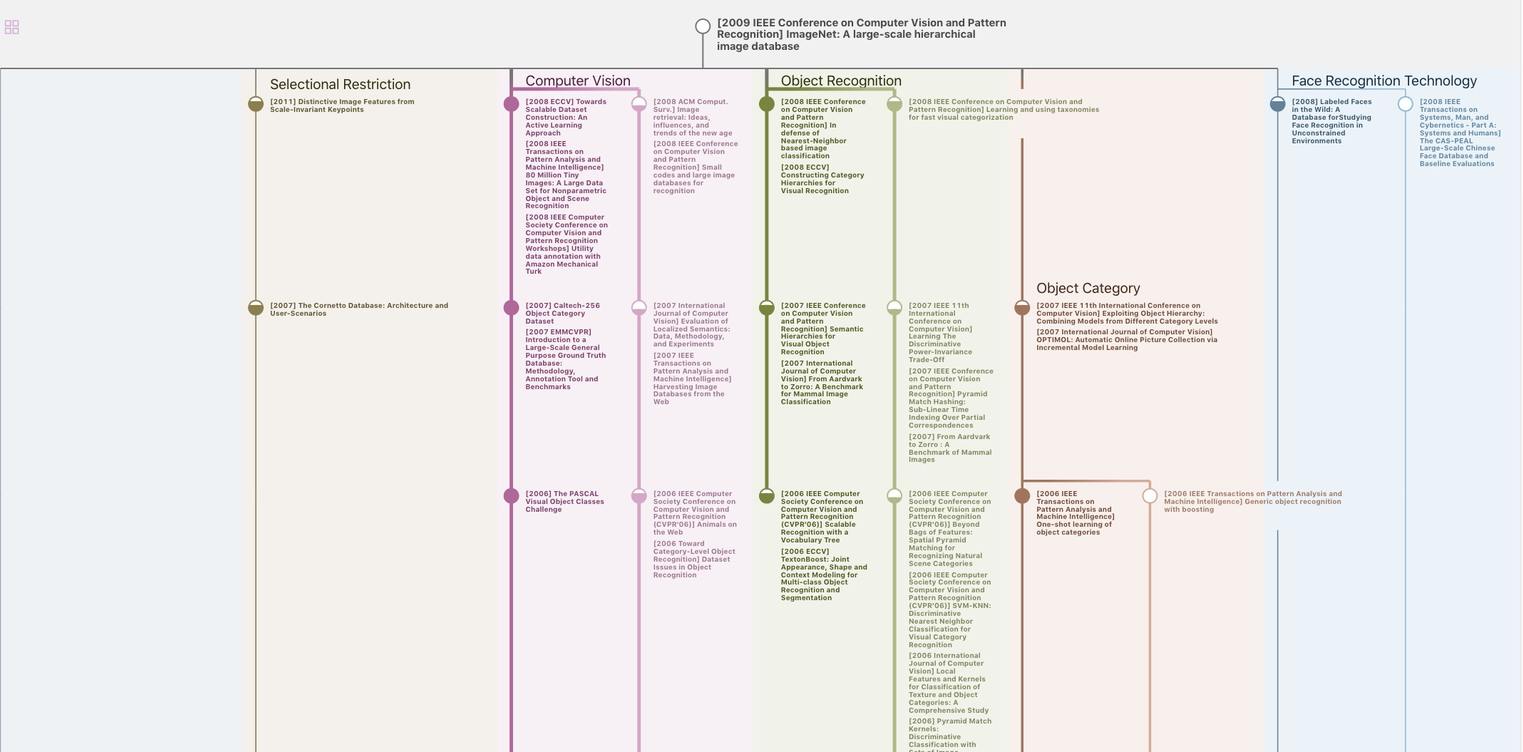
生成溯源树,研究论文发展脉络
Chat Paper
正在生成论文摘要