Discovery Of Patterns In Spatio-Temporal Data Using Clustering Techniques
2017 2ND INTERNATIONAL CONFERENCE ON IMAGE, VISION AND COMPUTING (ICIVC 2017)(2017)
摘要
Spatial-temporal clustering is very useful unsupervised learning technique and can be used to identify interesting distribution patterns from geo-Iocated data. It is one of the most commonly used data mining techniques in many application domains, e.g. geographic information science, health science, and environmental science. In this paper, we propose a density-based spatial-temporal clustering algorithm for geo-Iocated data points, based on an extension of the SNN (Shared Nearest Neighbor) clustering. The proposed algorithm allows the integration of location, time and other semantic attributes in the clustering process. This algorithm can find clusters of different sizes, shapes, and densities in noisy data. We evaluate the effectiveness of our algorithm through a case study involving a New York City taxi cab pickup data and Maryland crime data. The experimental results show that the proposed algorithm can discover interesting patterns and useful information from spatial-temporal data.
更多查看译文
关键词
clustering, spatial-temporal clustering, spatial-temporal patterns, shared nearest neighbor clustering
AI 理解论文
溯源树
样例
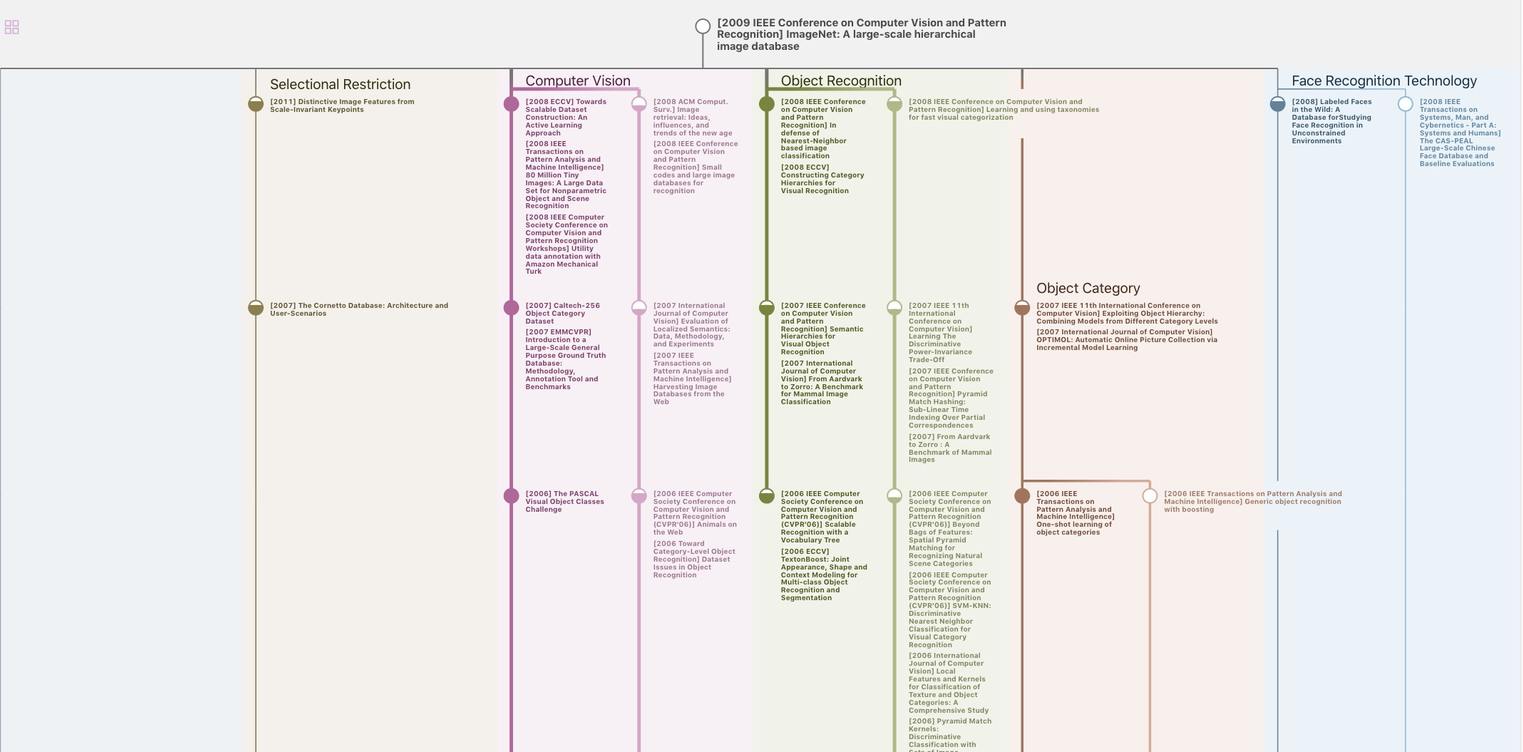
生成溯源树,研究论文发展脉络
Chat Paper
正在生成论文摘要