Automating Deconvolution Of Heterogeneous Bulk Tumor Genomic Data
CANCER RESEARCH(2017)
摘要
Tumor heterogeneity has been linked to many measures of poor patient outcome. Despite numerous recent methodological advances, though, reliable estimates of clonal and subclonal architecture remain elusive. We present advances in computational deconvolution of tumor heterogeneity, aimed at better handling complex substructure, more automated inference, and increased flexibility to heterogeneous genomic data sources. Our methods make use of geometric models of mixture substructure, a specialized form of a strategy called manifold learning, to better resolve clonal substructure shared across genomic samples. We validate the methods on breast tumor dataset from the Cancer Genome Atlas (TCGA). We find statistically significant Spearman correlation between inferred mixture models and independently derived clinical subtype across DNA, RNA, and combined datasets. DAVID tissue term enrichment, applied to inferred amplified gene sets, further yields significant correlation (p Citation Format: Theodore Roman, Brenda Xiao, Russell Schwartz. Automating deconvolution of heterogeneous bulk tumor genomic data [abstract]. In: Proceedings of the American Association for Cancer Research Annual Meeting 2017; 2017 Apr 1-5; Washington, DC. Philadelphia (PA): AACR; Cancer Res 2017;77(13 Suppl):Abstract nr 974. doi:10.1158/1538-7445.AM2017-974
更多查看译文
AI 理解论文
溯源树
样例
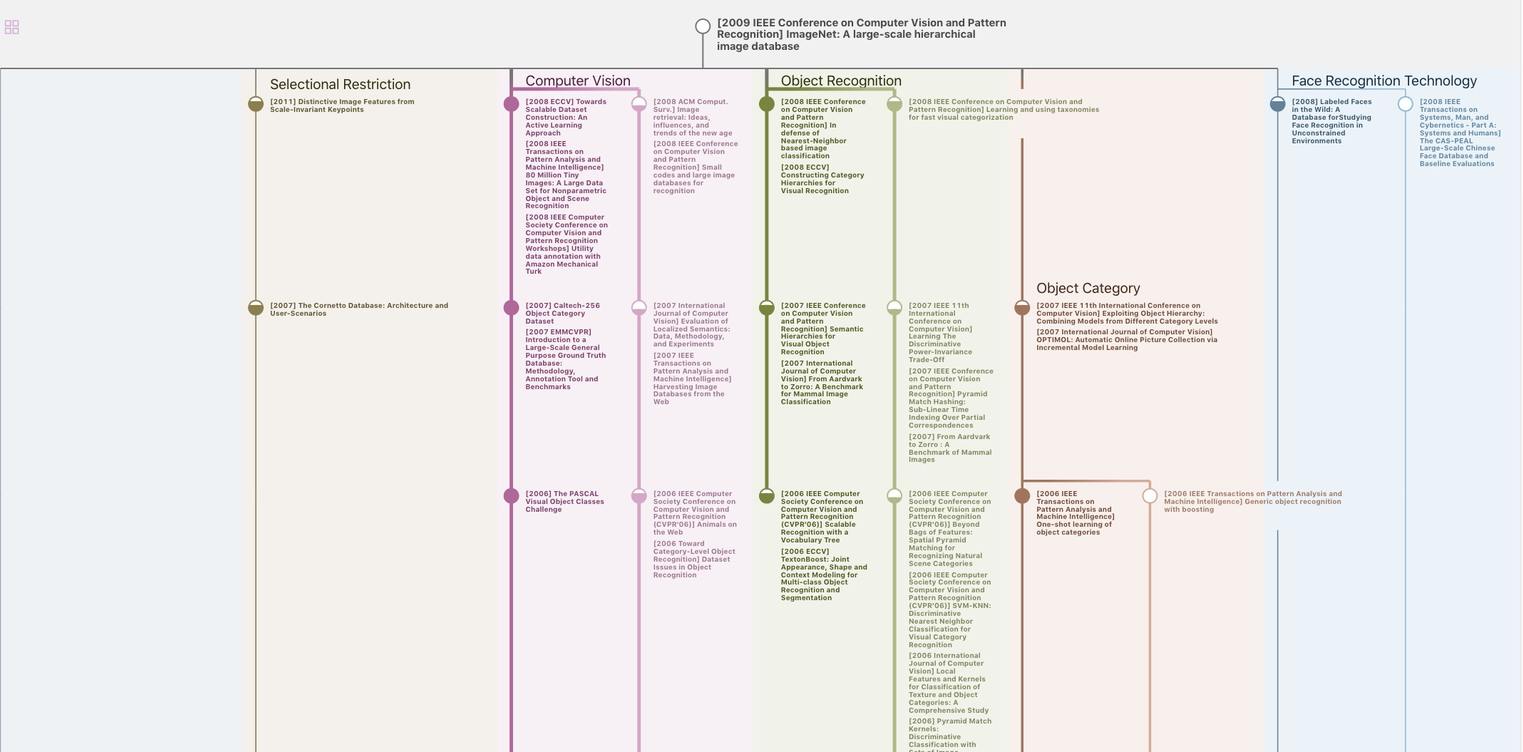
生成溯源树,研究论文发展脉络
Chat Paper
正在生成论文摘要