Symmetric rank covariances: a generalized framework for nonparametric measures of dependence
BIOMETRIKA(2018)
摘要
The need to test whether two random vectors are independent has spawned many competing measures of dependence. We focus on nonparametric measures that are invariant under strictly increasing transformations, such as Kendall's tau, Hoeffding's D, and the Bergsma-Dassios sign covariance. Each exhibits symmetries that are not readily apparent from their definitions. Making these symmetries explicit, we define a new class of multivariate nonparametric measures of dependence that we call symmetric rank covariances. This new class generalizes the above measures and leads naturally to multivariate extensions of the Bergsma-Dassios sign covariance. Symmetric rank covariances may be estimated unbiasedly using U-statistics, for which we prove results on computational efficiency and large-sample behaviour. The algorithms we develop for their computation include, to the best of our knowledge, the first efficient algorithms for Hoeffding's D statistic in the multivariate setting.
更多查看译文
关键词
Dependence,Hoeffding's D,Independence testing,Kendall's tau,U-statistic
AI 理解论文
溯源树
样例
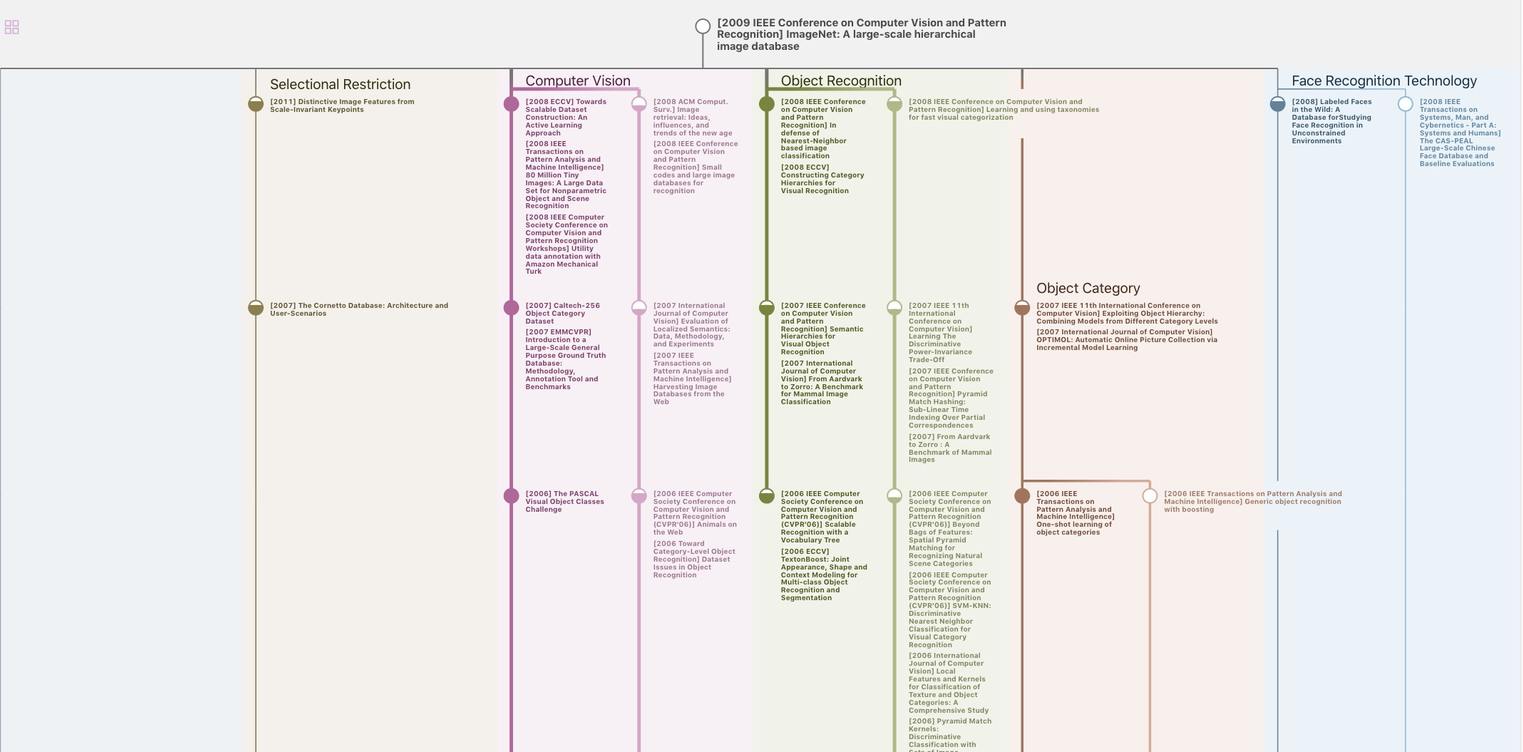
生成溯源树,研究论文发展脉络
Chat Paper
正在生成论文摘要