Combining classification and regression for improving permeability estimations from 1H NMR relaxation data
Journal of Applied Geophysics(2017)
摘要
Permeability is a key property to state if producing fluids from a hydrocarbon reservoir will be feasible or not. Nevertheless, the accurate estimation of permeability in carbonate rock reservoirs is considered a challenging task. This is due to two main reasons: (i) the extreme heterogeneity typically contained in these kinds of rocks; (ii) the fact that the permeability of carbonate rocks can vary enormously (from 0.01mD to more than 1000mD). In this paper, we explore a methodology to estimate permeability, from nuclear magnetic resonance (NMR) data, based on the combination of classification and regression techniques. This proposal works in two steps. First, data preprocessing and classification algorithms are applied to the original target dataset in order to build a classification model that is able to identify the permeability class zone – among four possible ranges – of a new rock sample. In the second step, according to the predicted permeability class zone, a specialized regression model is selected and will be responsible for determining the final permeability continuous value of the new rock sample. We evaluated this approach over a dataset formed by 1H NMR relaxation responses of 78 rock samples and their respective laboratory-measured permeability values. The obtained results revealed that the proposed approach, which combines classification and regression, led to more uniform and accurate predictions (RMSE=0.413 and r2=0.888) compared with the use of regression in a stand-alone manner (RMSE=0.641 and r2=0.735).
更多查看译文
关键词
Nuclear magnetic resonance,Permeability,Regression,Classification,Data mining
AI 理解论文
溯源树
样例
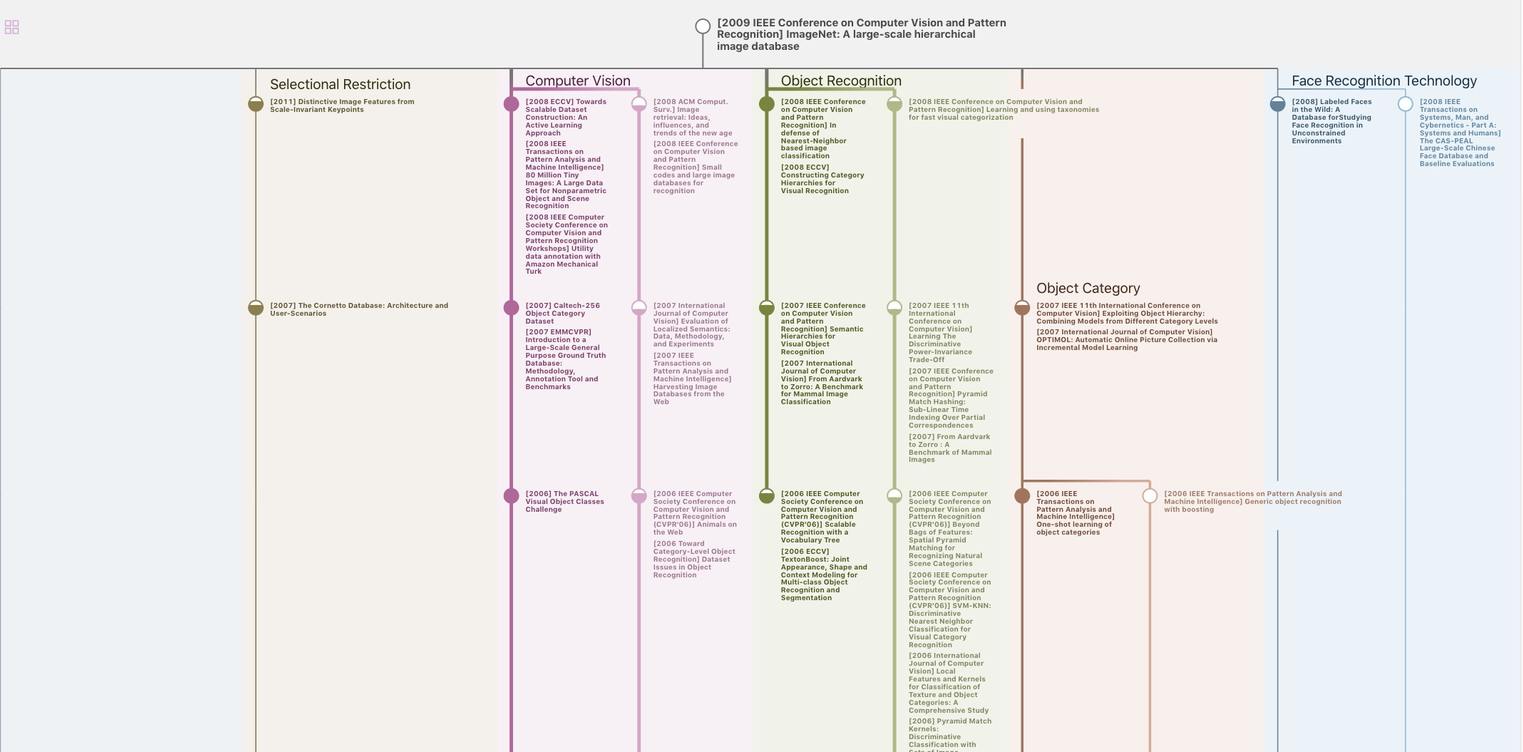
生成溯源树,研究论文发展脉络
Chat Paper
正在生成论文摘要