Post-modified non-negative matrix factorization for deconvoluting the gene expression profiles of specific cell types from heterogeneous clinical samples based on RNA-sequencing data
JOURNAL OF CHEMOMETRICS(2018)
摘要
The application of supervised algorithms in clinical practice has been limited by the lack of information on pure cell types. Several supervised algorithms have been proposed to estimate the gene expression patterns of specific cell types from heterogeneous samples. Post-modified non-negative matrix factorization (NMF), the unsupervised algorithm we proposed here, is capable of estimating the gene expression profiles and contents of the major cell types in cancer samples without any prior reference knowledge. Post-modified NMF was first evaluated using simulation data sets and then applied to deconvolution of the gene expression profiles of cancer samples. It exhibited satisfactory performance with both the validation and application data. For application in 3 types of cancer, the differentially expressed genes (DEGs) identified from the deconvoluted gene expression profiles of tumor cells were highly associated with the cancer-related gene sets. Moreover, the estimated proportions of tumor cells showed significant difference between the 2 compared patient groups in clinical endpoints. Our results indicated that the post-modified NMF can efficiently extract the gene expression patterns of specific cell types from heterogeneous samples for subsequent analysis and prediction, which will greatly benefit clinical prognosis.
更多查看译文
关键词
cancer,deconvolution,non-negative matrix factorization,RNA sequencing,prognosis
AI 理解论文
溯源树
样例
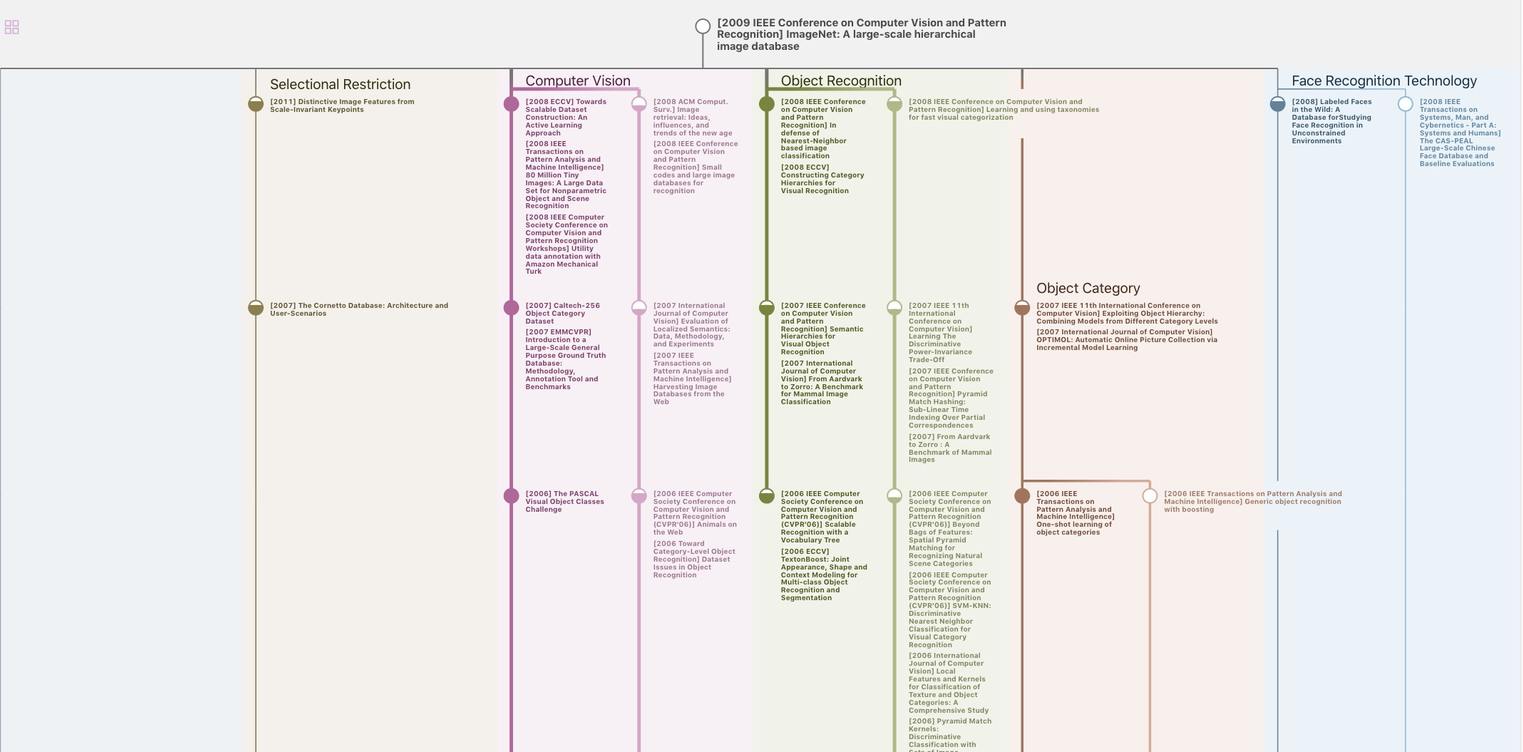
生成溯源树,研究论文发展脉络
Chat Paper
正在生成论文摘要