Recycling for Fairness: Learning with Conditional Distribution Matching Constraints
neural information processing systems(2017)
摘要
Equipping machine learning models with ethical and legal constraints is a serious issue; without this, the future of machine learning is at risk. This paper takes a step forward in this direction and focuses on ensuring machine learning models deliver fair decisions. In the legal scholarships, the notion of fairness itself is evolving and multi-faceted. We set an overarching goal to develop a unified machine learning framework that is able to handle any definitions of fairness, their combinations, and also new definitions that might be stipulated in the future. To achive our goal, we recycle two well-established machine learning techniques, privileged learning and distribution matching, and harmonize them for satisfying multi-faceted fairness definitions. We consider protected characteristics such as race and gender as privileged information; this accelerates model training and delivers fairness through unawareness. Further, we cast demographic parity, equalized odds, and equal opportunity as a classical two-sample problem of conditional distributions, which can be solved in a general form by using distance measures in Hilbert Space. Finally, we show several existing models are special cases of ours.
更多查看译文
AI 理解论文
溯源树
样例
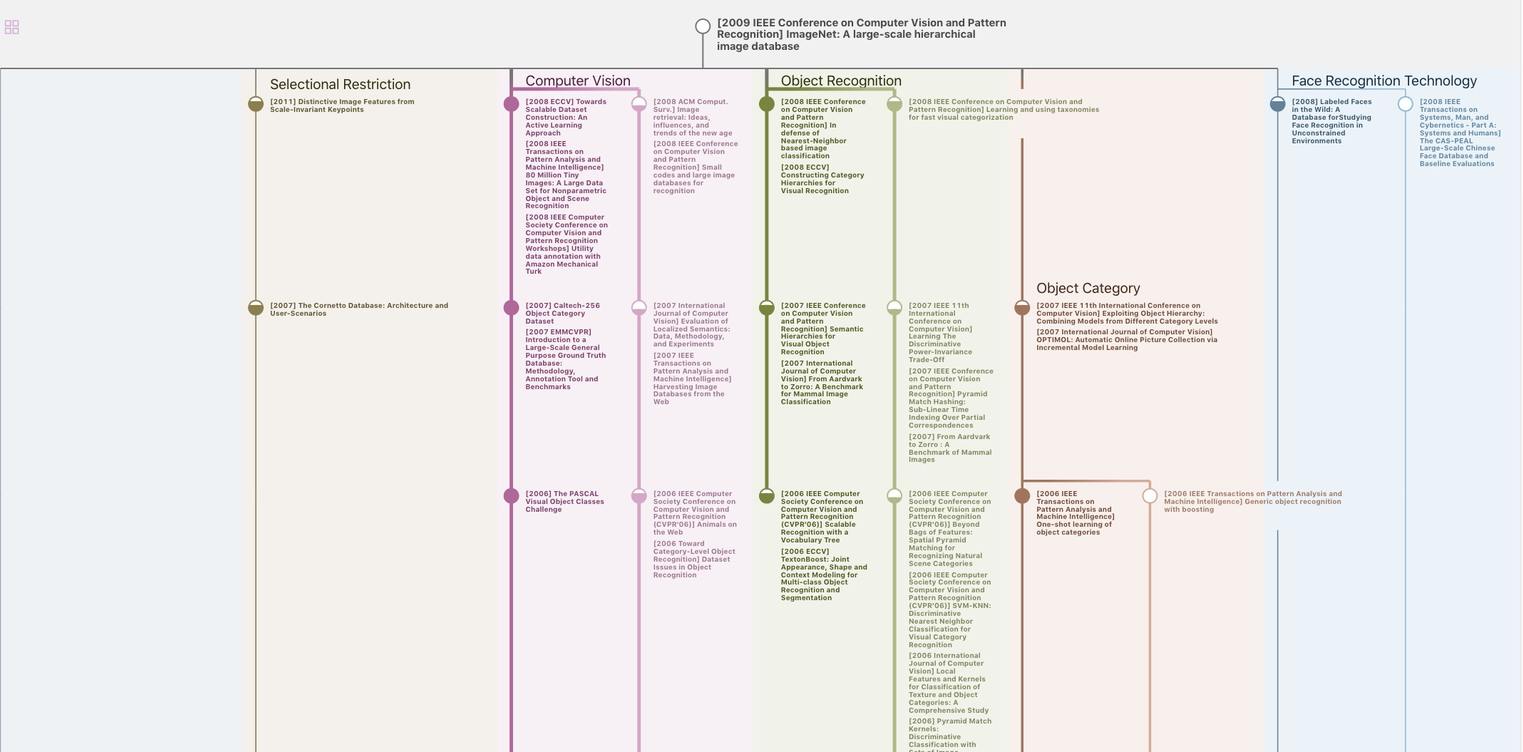
生成溯源树,研究论文发展脉络
Chat Paper
正在生成论文摘要