Deep learning methods for on-line flexibility prediction and optimal resource allocation in smart buildings
power and energy society general meeting(2017)
摘要
Unprecedented high volume of data is available with the upward growth of the advanced metering infrastructure. Because the built environment is the largest user of electricity, a deeper look at building energy consumption holds promise for helping to achieve overall optimization of the energy system. Yet, a knowledge transfer from the fusion of extensive data is under development. To overcome this limitation, in the big data era, more and more machine learning methods appear to be suitable to automatically extract, predict and optimized building electrical patterns by performing successive transformation of the data. More recently, there has been a revival of interest in deep learning methods as the most advance on-line solutions for large-scale and real databases. Enabling real-time applications from the high level of aggregation in the smart grid will put end-users in position to change their consumption patterns, offering useful benefits for the system as a whole.
更多查看译文
AI 理解论文
溯源树
样例
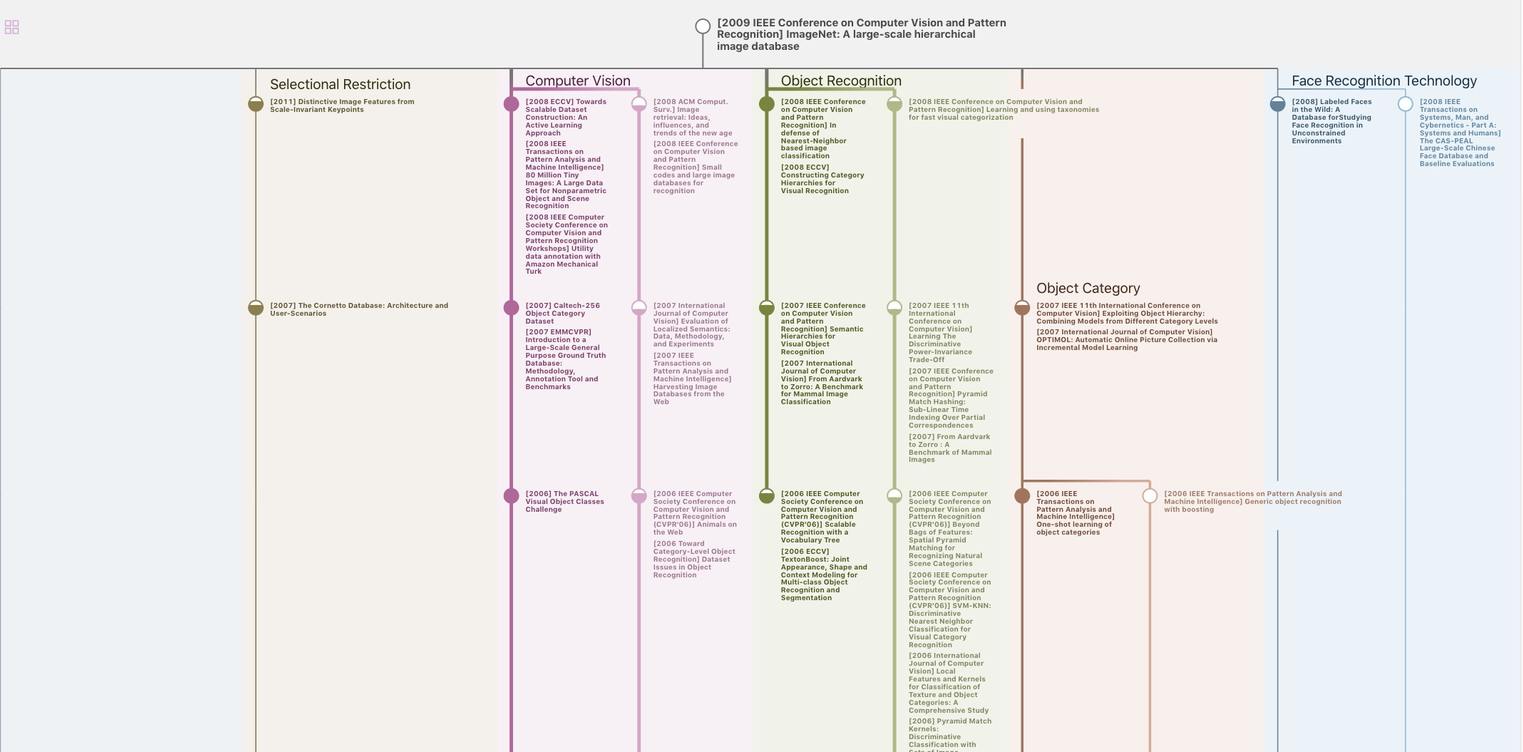
生成溯源树,研究论文发展脉络
Chat Paper
正在生成论文摘要