On Input/Output Architectures for Convolutional Neural Network-Based Cross-View Gait Recognition
IEEE Transactions on Circuits and Systems for Video Technology(2019)
摘要
In this paper, we discuss input/output architectures for convolutional neural network (CNN)- based cross-view gait recognition. For this purpose, we consider two aspects: verification versus identifi- cation, and the trade-off between spatial displacement caused by subject difference and view difference. More specifically, we use the Siamese network with a pair of inputs and contrastive loss for verification, and a triplet network with a triplet of inputs and triplet ranking loss for identification. The aforementioned CNN architec- tures are insensitive to spatial displacement because the difference between a matching pair is calculated at the last layer after passing through the convolution and max pooling layers; hence, they are expected to work relatively well under large view differences. By contrast, because it is better to use the spatial displace- ment to its best advantage because of the subject dif- ference under small view differences, we also use CNN architectures where the difference between a matching pair is calculated at the input level to make them more sensitive to spatial displacement. We conducted experiments for cross-view gait recognition and con- firmed that the proposed architectures outperformed the state-of-the-art benchmarks in accordance with their suitable situations of verification/identification tasks and view differences.
更多查看译文
关键词
Gait recognition,Probes,Network architecture,Robustness,Performance evaluation,Neural networks
AI 理解论文
溯源树
样例
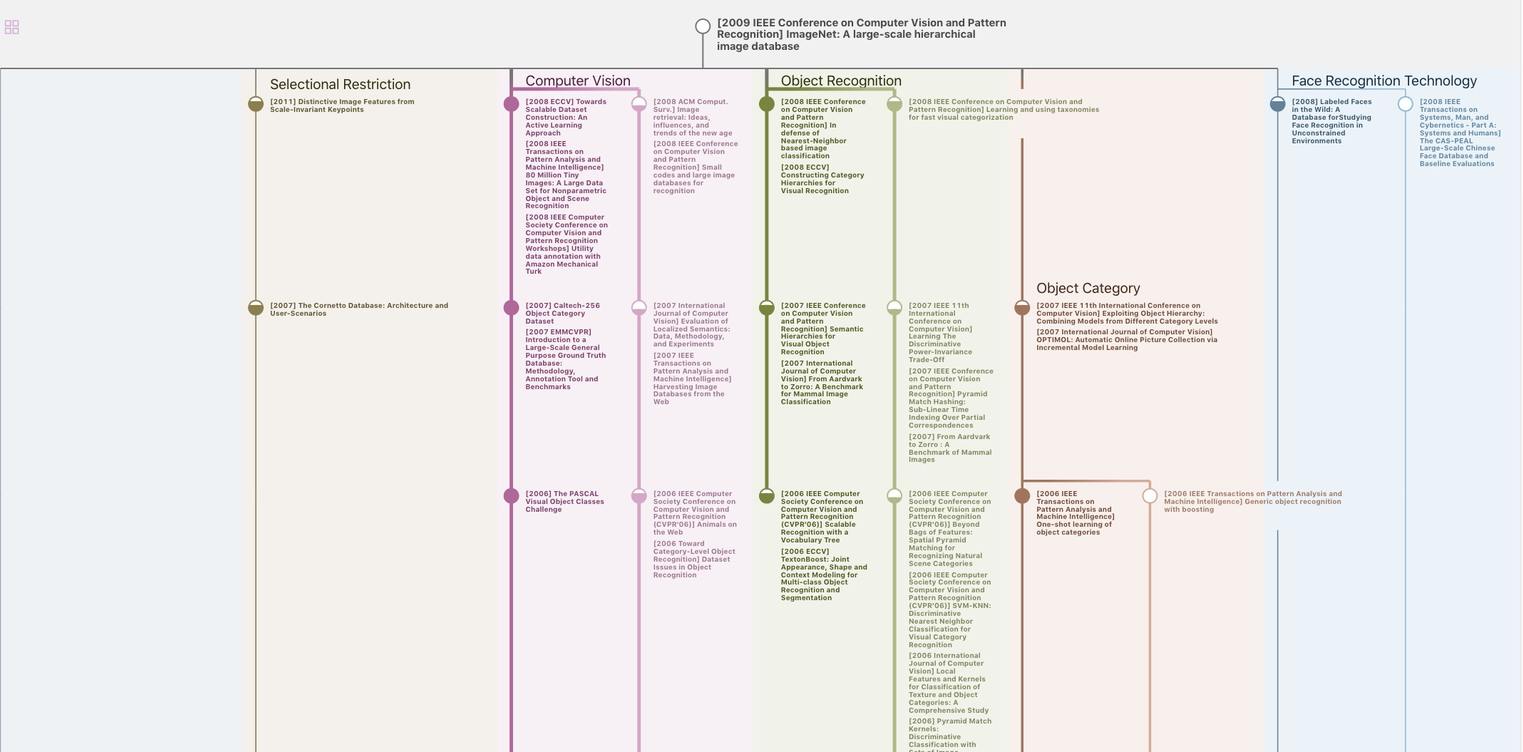
生成溯源树,研究论文发展脉络
Chat Paper
正在生成论文摘要