Human Emotion Recognition with Electroencephalographic Multidimensional Features by Hybrid Deep Neural Networks
APPLIED SCIENCES-BASEL(2017)
摘要
The aim of this study is to recognize human emotions by electroencephalographic (EEG) signals. The innovation of our research methods involves two aspects: First, we integrate the spatial characteristics, frequency domain, and temporal characteristics of the EEG signals, and map them to a two-dimensional image. With these images, we build a series of EEG Multidimensional Feature Image (EEG MFI) sequences to represent the emotion variation with EEG signals. Second, we construct a hybrid deep neural network to deal with the EEG MFI sequences to recognize human emotional states where the hybrid deep neural network combined the Convolution Neural Networks (CNN) and Long Short-Term-Memory (LSTM) Recurrent Neural Networks (RNN). Empirical research is carried out with the open-source dataset DEAP (a Dataset for Emotion Analysis using EEG, Physiological, and video signals) using our method, and the results demonstrate the significant improvements over current state-of-the-art approaches in this field. The average emotion classification accuracy of each subject with CLRNN (the hybrid neural networks that we proposed in this study) is 75.21%.
更多查看译文
关键词
emotion recognition,EEG signal,multidimensional features,hybrid neural networks,CNN,LSTM RNN
AI 理解论文
溯源树
样例
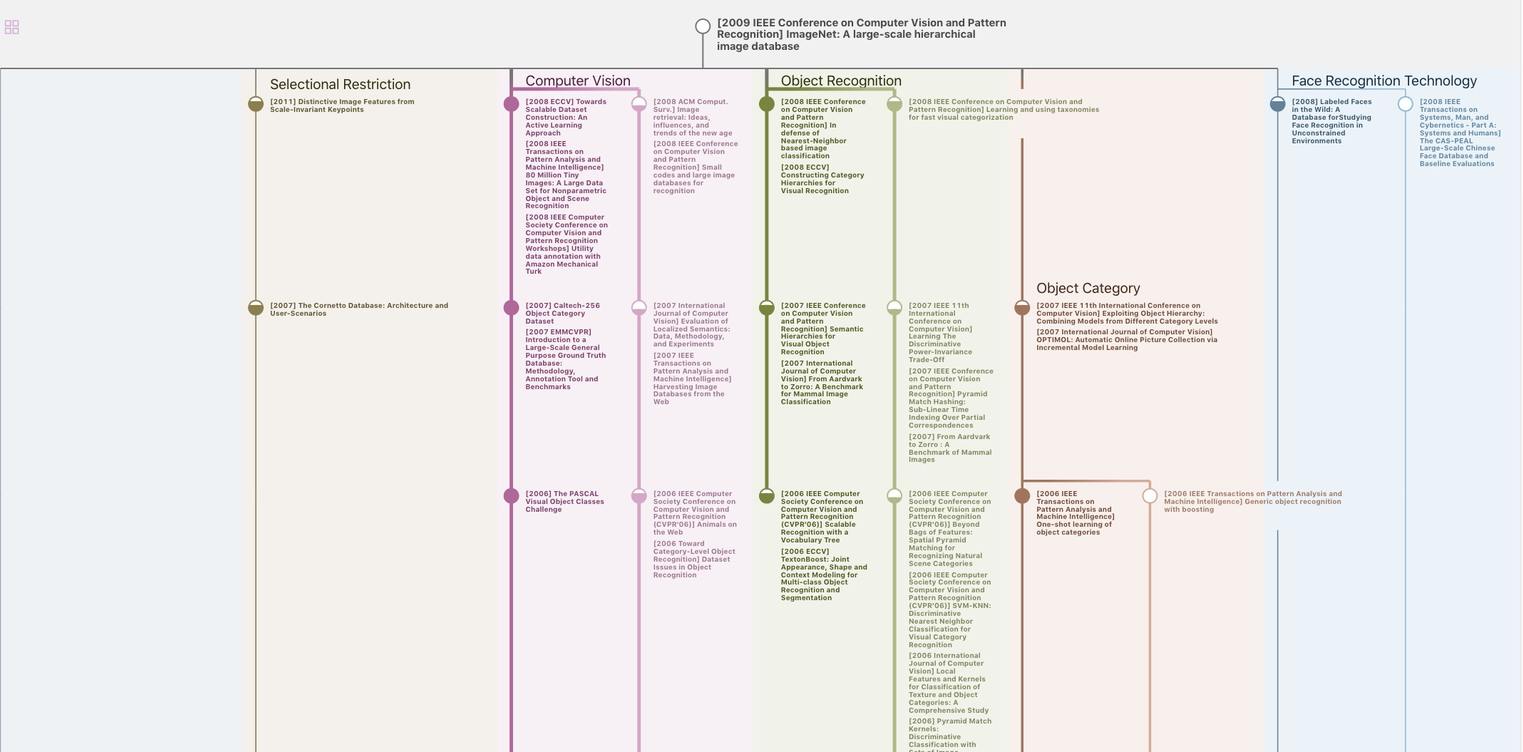
生成溯源树,研究论文发展脉络
Chat Paper
正在生成论文摘要