Trajectory-pooled Spatial-temporal Architecture of Deep Convolutional Neural Networks for Video Event Detection
IEEE Transactions on Circuits and Systems for Video Technology(2019)
摘要
Nowadays Content-based video event detection faces great challenges due to complex scenes and blurred actions in surveillance videos. To alleviate these challenges, we propose a novel spatial-temporal architecture of deep Convolutional Neural Networks for this task. By taking advantage of spatial-temporal information, we fine-tune two-stream networks, and then fuse spatial and temporal features at convolution layers using a 2D pooling fusion method to enforce the consistence of spatial-temporal information. Based on the two-stream networks and spatial-temporal layer, a triple-channel model is obtained. Furthermore, we implement trajectory-constrained pooling to deep features and hand-crafted features to combine their merits. A fusion method on triple-channel yields the final detection result. The experiments on two benchmark surveillance video datasets including VIRAT 1.0 and VIRAT 2.0, which involve a suit of challenging events, such as person loading an object to a vehicle or person opening a vehicle trunk, manifest that the proposed method can achieve superior performance compared with the state-of-the-art methods on these event benchmarks.
更多查看译文
关键词
Trajectory,Event detection,Feature extraction,Computer vision,Computer architecture,Image motion analysis,Fuses
AI 理解论文
溯源树
样例
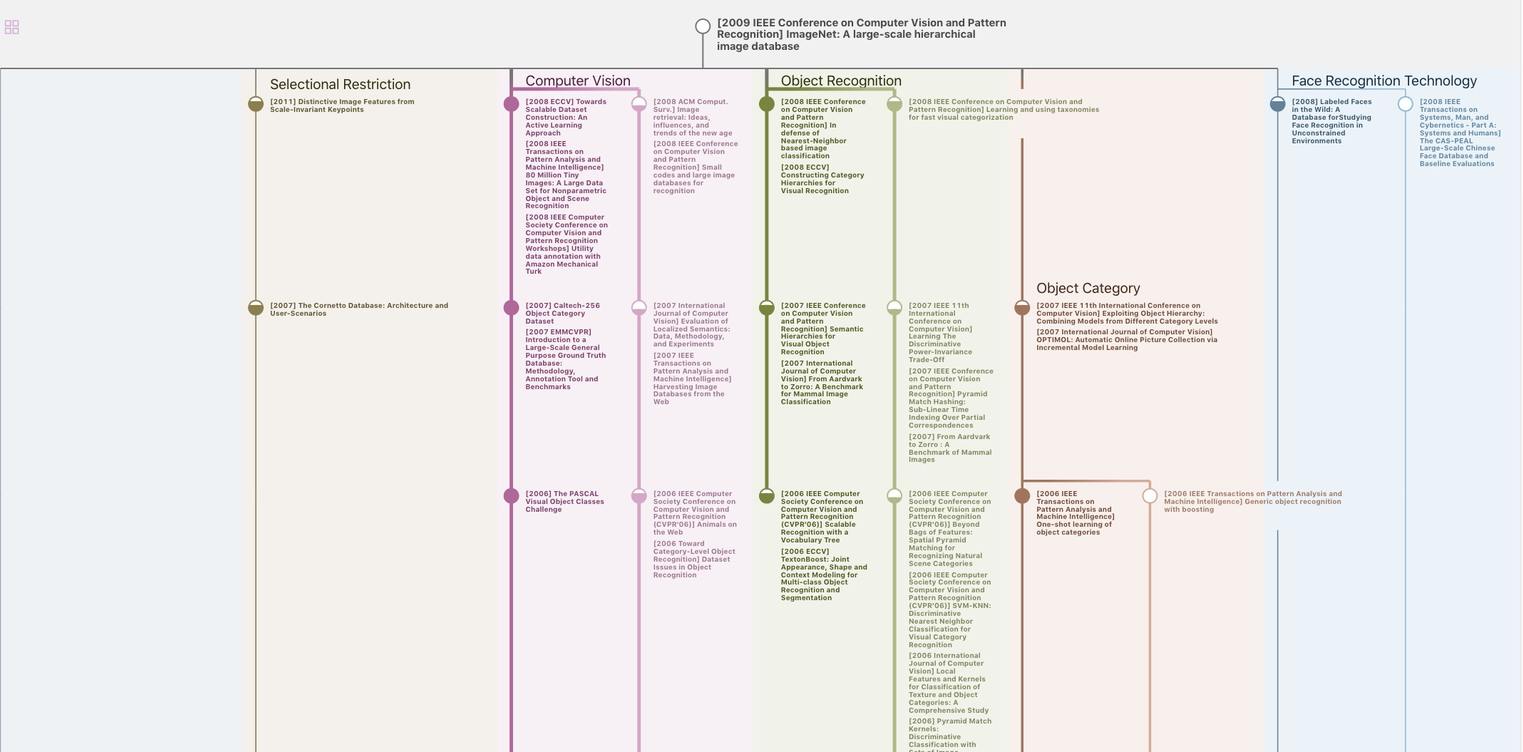
生成溯源树,研究论文发展脉络
Chat Paper
正在生成论文摘要