Variational Autoencoder: An Unsupervised Model for Modeling and Decoding fMRI Activity in Visual Cortex
bioRxiv(2018)
摘要
Goal-driven and feedforward-only convolutional neural networks (CNN) have been shown to be able to predict and decode cortical responses to natural images or videos. Here, we explored an alternative deep neural network, variational auto-encoder (VAE), as a computational model of the visual cortex. We trained a VAE with a five-layer encoder and a five-layer decoder to learn visual representations from a diverse set of unlabeled images. Inspired by the free-energy principle in neuroscience, we modeled the brain9s bottom-up and top-down pathways using the VAE9s encoder and decoder, respectively. Following such conceptual relationships, we used VAE to predict or decode cortical activity observed with functional magnetic resonance imaging (fMRI) from three human subjects passively watching natural videos. Compared to CNN, VAE resulted in relatively lower accuracies for predicting the fMRI responses to the video stimuli, especially for higher-order ventral visual areas. However, VAE offered a more convenient strategy for decoding the fMRI activity to reconstruct the video input, by first converting the fMRI activity to the VAE9s latent variables, and then converting the latent variables to the reconstructed video frames through the VAE9s decoder. This strategy was more advantageous than alternative decoding methods, e.g. partial least square regression, by reconstructing both the spatial structure and color of the visual input. Findings from this study support the notion that the brain, at least in part, bears a generative model of the visual world.
更多查看译文
关键词
neural coding,variational autoencoder,Bayesian brain,visual reconstruction
AI 理解论文
溯源树
样例
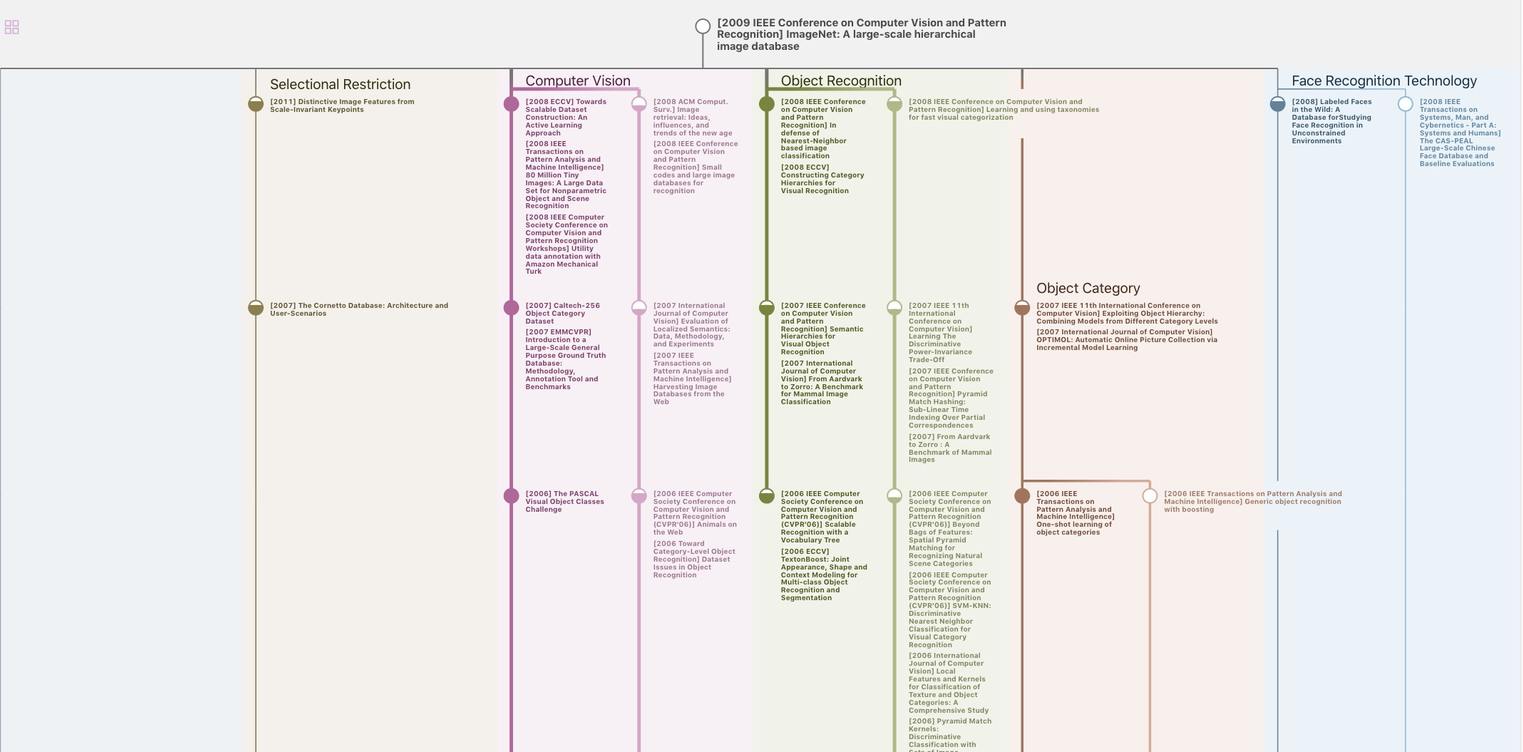
生成溯源树,研究论文发展脉络
Chat Paper
正在生成论文摘要