An ensemble learning-based fault diagnosis method for rotating machinery
2017 Prognostics and System Health Management Conference (PHM-Harbin)(2017)
摘要
Fault diagnosis is a major concern of the prognostics and health management of rotating machinery. Current practice in fault diagnosis is often challenged by the non-normality, multimodality, and nonlinearity of machinery health monitoring signals and their extracted features. A single classifier used in fault diagnosis fails when all these challenges exist. Thus, in this paper a hybrid ensemble learning method is developed to combine the capability of different classifiers to address the challenges. Diversity among classifiers is desired because diversified classifiers lead to uncorrelated classifications, which improve classification accuracy. In this paper two methods are used to increase the diversity. First, different algorithms compatible with rotating machinery data are included in the decision ensemble to get the diversity among algorithms. Second, multiple bootstrap samples are generated to increase the diversity among training data. Each algorithm is trained by multiple bootstrap samples to get multiple classifiers. At the end, classifiers are trained from different combinations of algorithms and bootstrap samples. A final classification result is obtained from the majority voting of the classifiers. The method was evaluated by the classification of simulated data and through the fault diagnosis of experimental data of bearings. Results show the method works when the challenges exist and the performance of the method is better than that of individual classifiers.
更多查看译文
关键词
classification,ensemble learning,fault diagnosis,rotating machinery
AI 理解论文
溯源树
样例
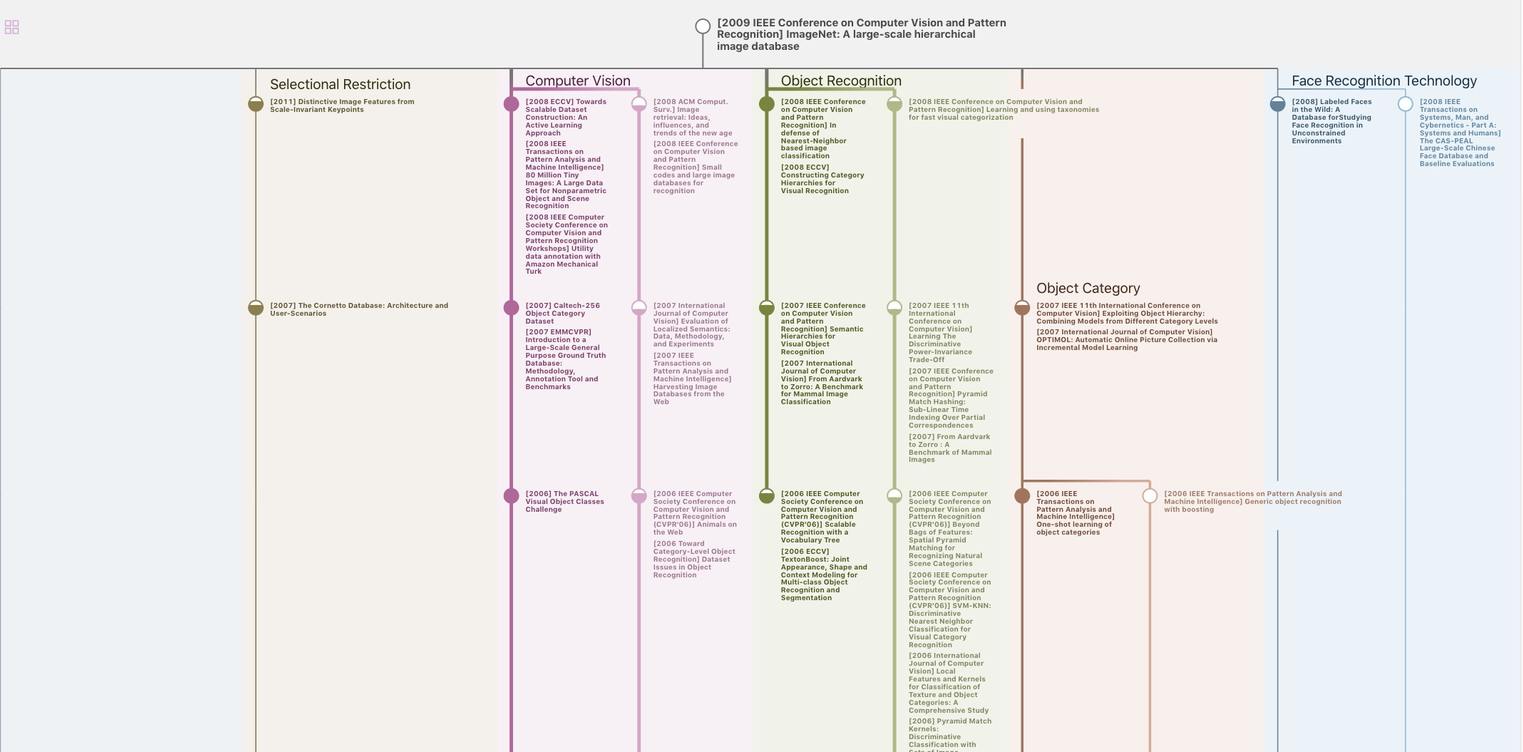
生成溯源树,研究论文发展脉络
Chat Paper
正在生成论文摘要