Dynamic Text Categorization of Search Results for Medical Class Recognition in Real World Evidence Studies in the Chinese Language
international conference on bioinformatics(2017)
摘要
Classifying clinical terms from electronic medical record (EMR) systems is critical for real world evidence (RWE) research. Yet the task is challenging, especially in languages other than English. Clinical research institutes require a cost-effective method to address this challenge. We proposed a software pipeline with two components: a feature generator that gathers descriptive words of the terms by text-segmenting the search results from two search engines and a learning mechanism that utilizes machine learning algorithms for classification. Models are trained with training sets of different sizes to determine effectiveness. Models were compared using 10-fold cross validation or another supplied testing set. We applied our pipeline to a Chinese medication term set extracted from a clinical system, and also to a data set of standard medications names. A term-vs.-word frequency matrix was generated based on the Google search results of the term sets. Most models tasked with classifying whether a medication belonged to Western or Chinese medicine achieved high accuracy, especially with radial basis functions (RBF) network. The performance of models trained with training sets of different sizes was not significantly different. When the same approach was applied to the information gathered from another Chinese language search engine (Baidu), better performance was achieved. The results of the other experiments conducted on the medication name set also demonstrates a significant improvement from baseline. Dynamic text categorization with machine learning can be applied to classify clinical terms based on information retrieved from search engines in RWE studies.
更多查看译文
AI 理解论文
溯源树
样例
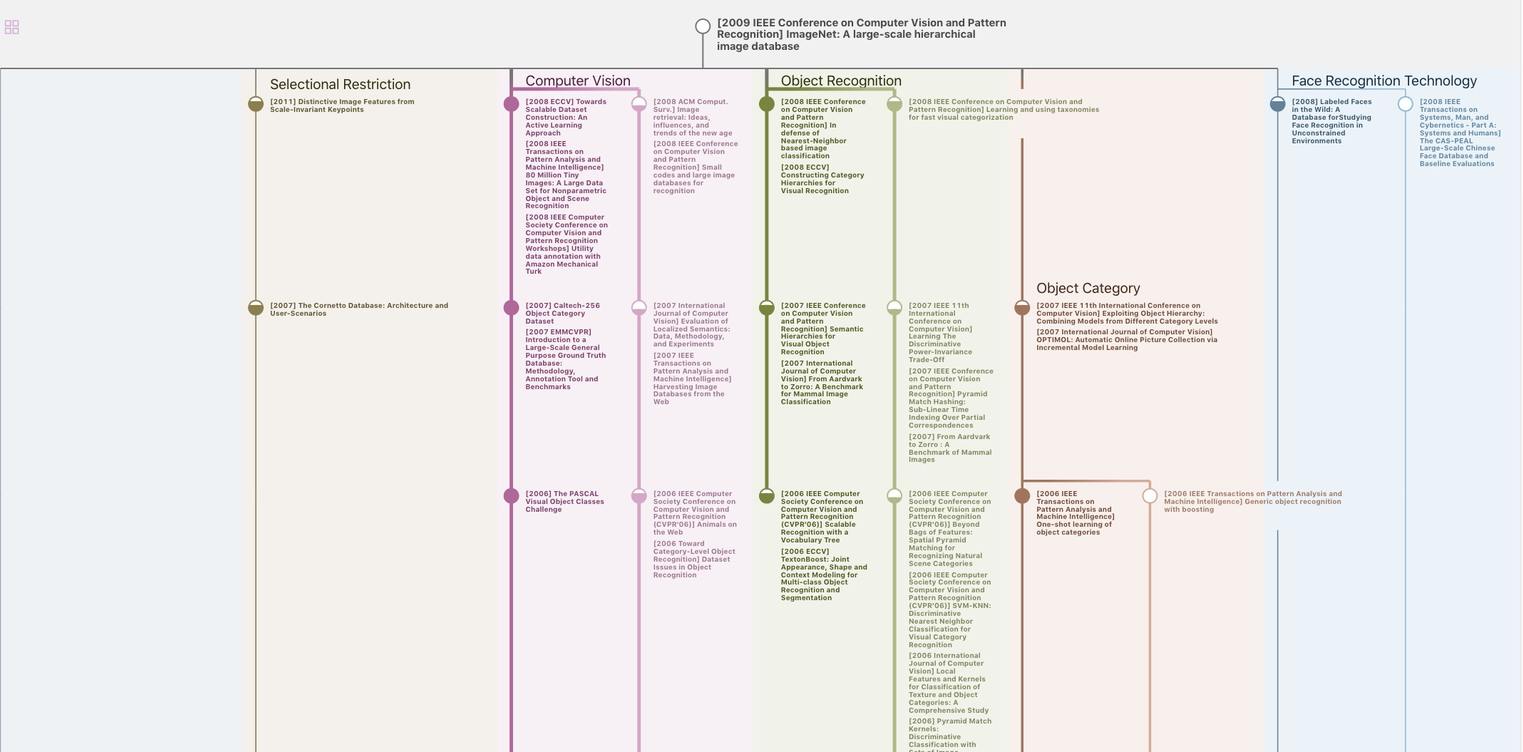
生成溯源树,研究论文发展脉络
Chat Paper
正在生成论文摘要