Scalable Neural Network Decoders for Higher Dimensional Quantum Codes
QUANTUM(2018)
Abstract
Machine learning has the potential to become an important tool in quantum error correction as it allows the decoder to adapt to the error distribution of a quantum chip. An additional motivation for using neural networks is the fact that they can be evaluated by dedicated hardware which is very fast and consumes little power. Machine learning has been previously applied to decode the surface code. However, these approaches are not scalable as the training has to be redone for every system size which becomes increasingly difficult. In this work the existence of local decoders for higher dimensional codes leads us to use a low-depth convolutional neural network to locally assign a likelihood of error on each qubit. For noiseless syndrome measurements, numerical simulations show that the decoder has a threshold of around 7.1% when applied to the 4D toric code. When the syndrome measurements are noisy, the decoder performs better for larger code sizes when the error probability is low. We also give theoretical and numerical analysis to show how a convolutional neural network is different from the 1-nearest neighbor algorithm, which is a baseline machine learning method.
MoreTranslated text
Key words
Fault-tolerant Quantum Computation,Quantum Error Correction,Quantum Machine Learning,Quantum Simulation,Quantum Computation
AI Read Science
Must-Reading Tree
Example
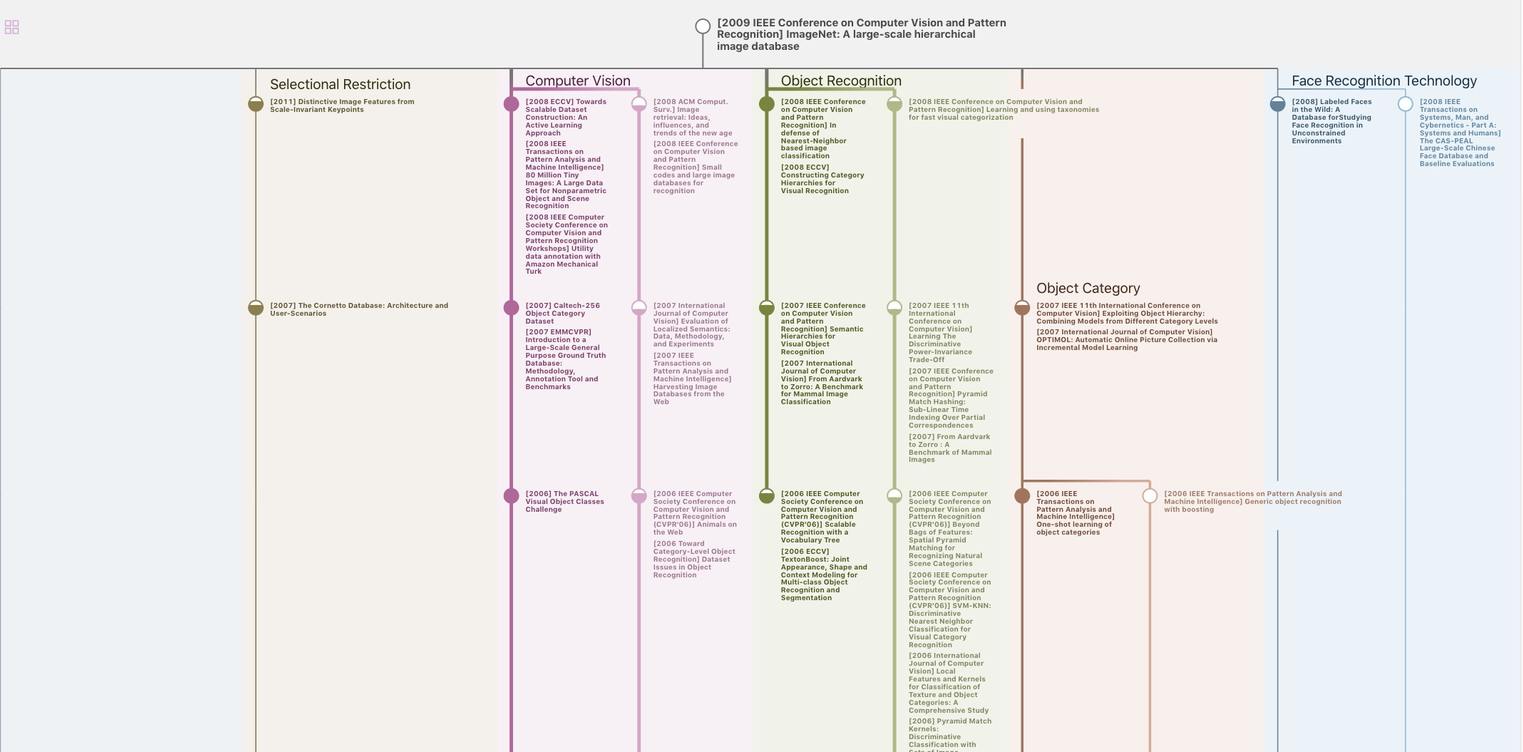
Generate MRT to find the research sequence of this paper
Chat Paper
Summary is being generated by the instructions you defined