Pattern-Growth based Mining Mouse-Interaction Behavior for an Active User Authentication System
IEEE Transactions on Dependable and Secure Computing(2020)
摘要
Analyzing mouse-interaction behaviors for implicitly identifying computer users has received growing interest from security and biometric researchers. This study presents a simple but efficient active user authentication system by modeling mouse-interaction behavior, which is accurate and competent for future deployments. A pattern-growth-based mining method is proposed to extract frequent behavior segments, in obtaining a stable and discriminative representation of mouse-interaction behavior. Then procedural features are extracted to provide an accurate and fine-grained characterization of the behavior segments. A SVM-based decision procedure using one-class learning techniques is applied to the feature space for performing authentication. Analyses are conducted using data from around 1,526,400 mouse operations of 159 participants, and the authentication performance is evaluated across various application scenarios and tasks. Our experimental results show that characteristics from frequent behavior segments are more stable and discriminative than those from holistic behavior, and the system achieves a practically useful level of performance with FAR of 0.09 percent and FRR of 1 percent. Additional experiments on usability to sample length, reliability to application task, scalability to user size, robustness to mimic attack, and response to behavior change are provided to further explore the applicability. We also compare the proposed approach with the state-of-the-art approaches for the collected data.
更多查看译文
关键词
Mice,Authentication,Feature extraction,Monitoring
AI 理解论文
溯源树
样例
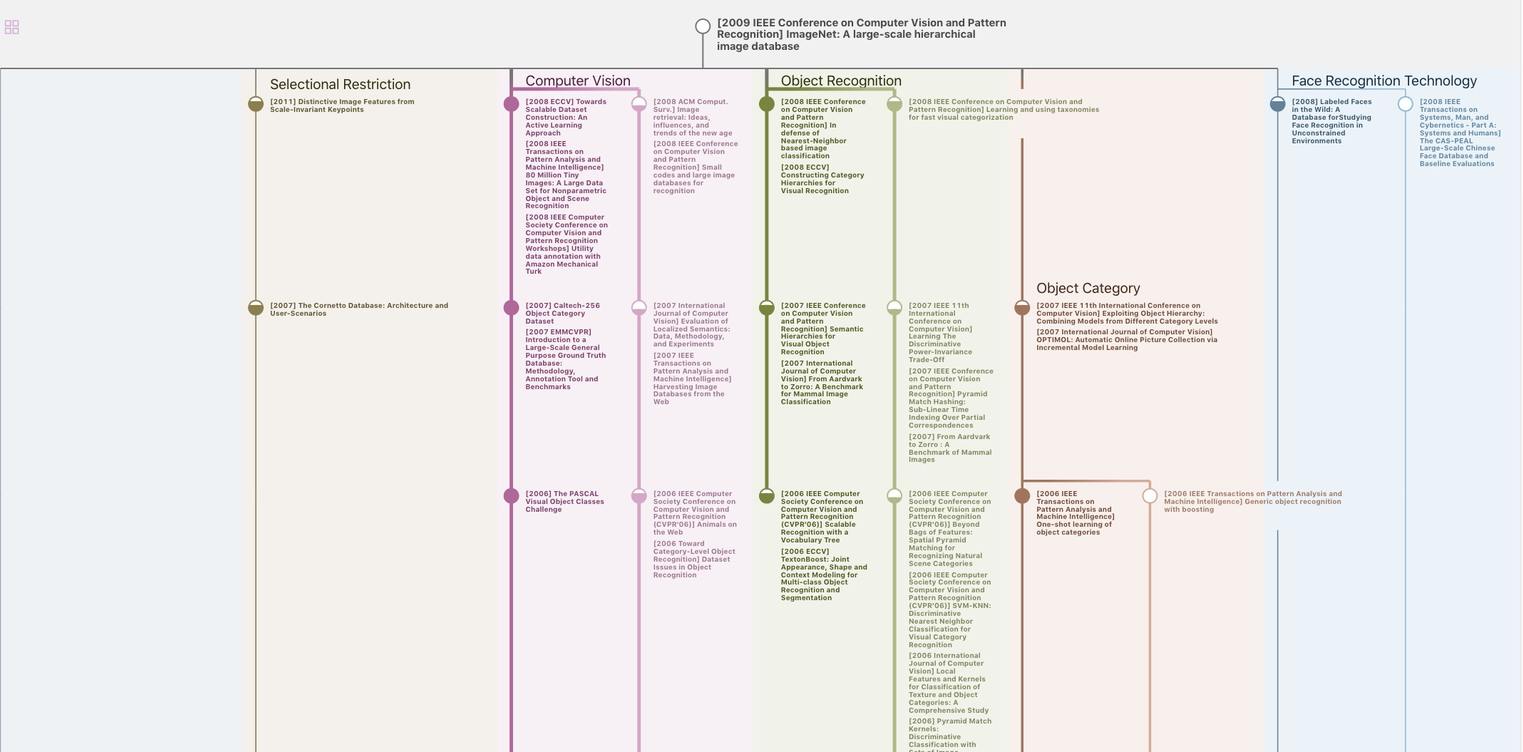
生成溯源树,研究论文发展脉络
Chat Paper
正在生成论文摘要