Low-rank based infrared spectral feature extraction framework for quantitative analysis
Optik(2018)
摘要
Feature extraction is a key problem in spectral analysis. Spectrum collected with spectrometer have latent low-rank component. If spectrum can be represented as a superposition of low-rank component and an approximation term, the spectrum feature is obtained. In this paper, a novel low-rank based infrared spectral feature extraction method is proposed. Employing a slide window to convert a single spectrum into a matrix, which can be decomposed as the superposition of a low-rank component and feature. In machine learning, nuclear norm is employed to approximate to low-rank minimization. Thus, the model can be written as a combination of the nuclear norm and an approximation term. We have proposed an efficient algorithm with singular value decomposition to the model. Solving the model, we obtain the latent low-rank component in spectrum. The feature is obtained via derivative of original and low-rank approximation. Then the quantitative analysis model is directly built with the feature. The advantage of proposed method is that extraction procedure of one spectrum is not affected by other spectrum. Extensive experiments are conducted with four public data sets and experimental results demonstrate that our proposed feature extraction method can lead to accuracy improvements over state-of-the-art methods.
更多查看译文
关键词
Low-rank,Nuclear norm,Feature extraction,Quantitative analysis
AI 理解论文
溯源树
样例
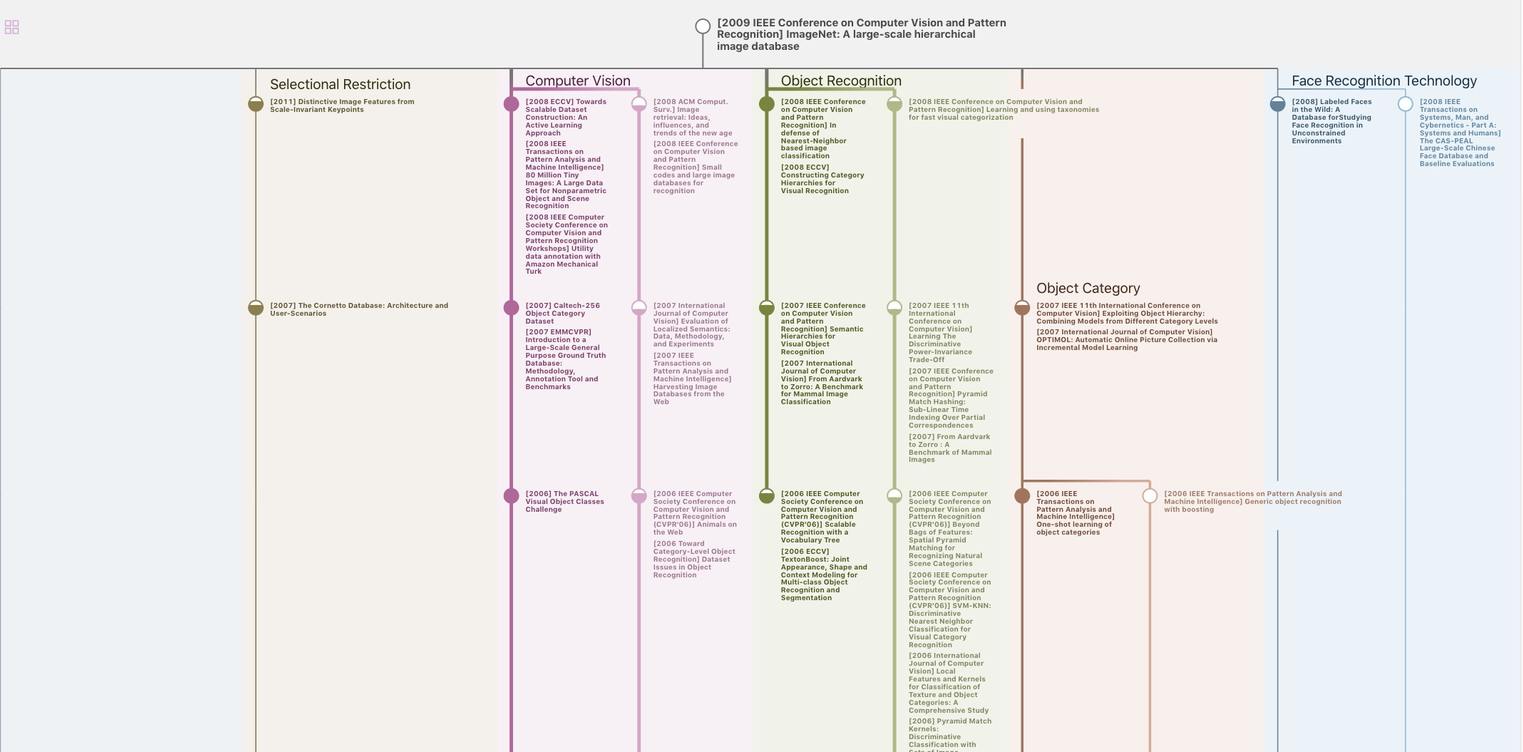
生成溯源树,研究论文发展脉络
Chat Paper
正在生成论文摘要