Prediction of Protein S-Sulfenylation Sites Using a Deep Belief Network
CURRENT BIOINFORMATICS(2018)
摘要
Background: Protein S-Sulfenylation, the reversible oxidative modification of cysteine thiol groups to cysteine S-Sulfenic acids, is a post-translational modification (PTM) that plays a critical role in regulating protein function and signal transduction. The identification of specific protein S-sulfenylation sites is crucial to understand the underlying molecular mechanisms. Objective: We sought to develop a computational method that can effectively predict S-sulfenylation sites by using optimally extracted properties. Method: We propose DBN-Sulf, which uses a Deep Belief Network (DBN) with Restricted Boltzmann Machines (RBMs) to reduce the feature dimensions from a combination of heterogeneous information, including amino acid related features, evolutionary features, and structure-based features. Then a support vector machine (SVM) based predictor is built with the optimal features. Results: We evaluate the DBN-Sulf classifier using a training dataset including 1007 positive sites and 7837 negative sites with 5-fold cross validation, and get an AUC score of 0.80, an ACC of 0.85 and a MCC of 0.53, which are significantly better than that of the existing methods. We further validate our method on the independent test set and obtain promising results. Conclusion: The superior performance over existing S-sulfenylation site prediction approaches indicates the importance of the deep belief network-based feature extracting procedure.
更多查看译文
关键词
Deep belief network,support vector machine,S-sulfenylation sites,restricted boltzmann machines
AI 理解论文
溯源树
样例
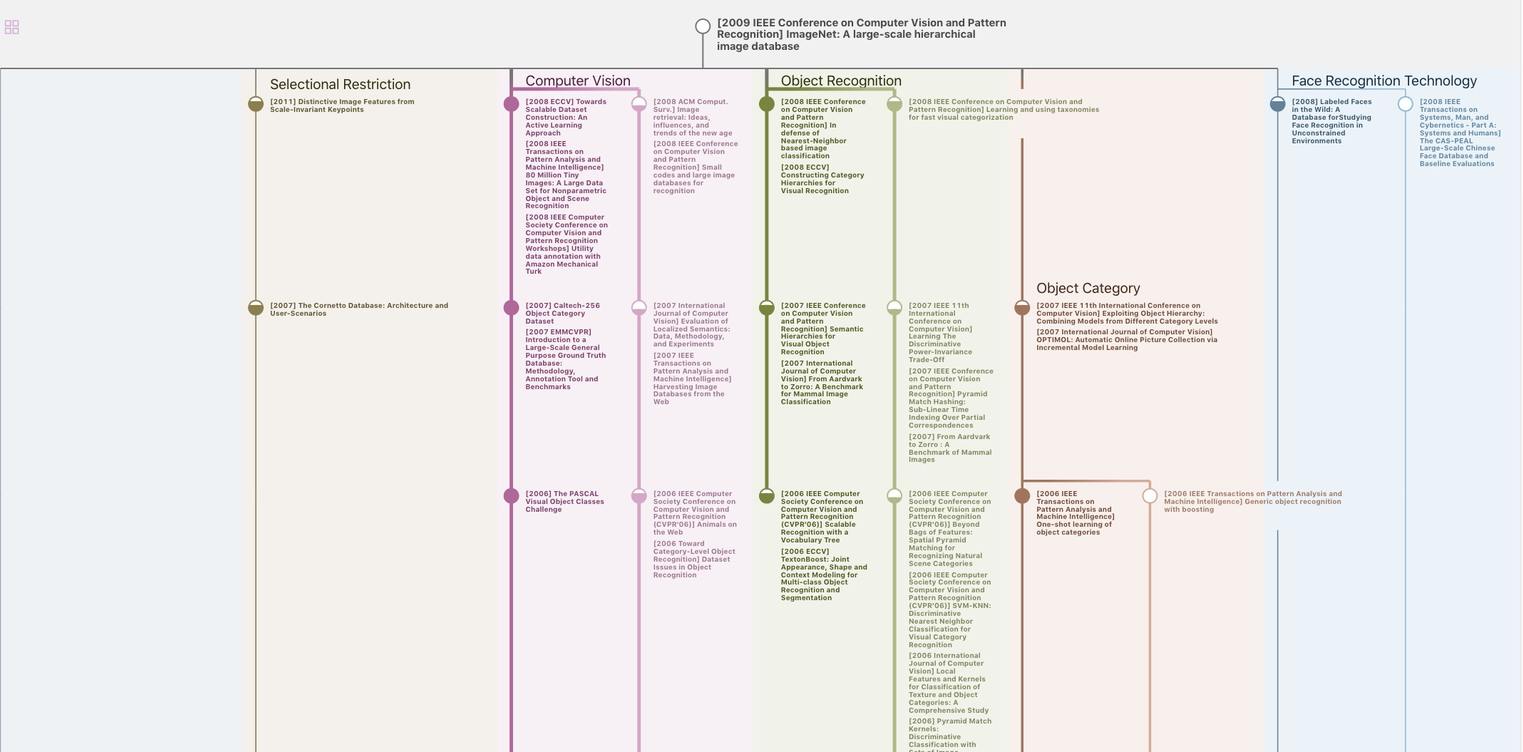
生成溯源树,研究论文发展脉络
Chat Paper
正在生成论文摘要