Sample average approximation with heavier tails II: localization in stochastic convex optimization and persistence results for the Lasso
arXiv: Optimization and Control(2023)
摘要
“Localization” has proven to be a valuable tool in the Statistical Learning literature as it allows sharp risk bounds in terms of the problem geometry. Localized bounds seem to be much less exploited in the stochastic optimization literature. In addition, there is an obvious interest in both communities in obtaining risk bounds that require weak moment assumptions or “heavier-tails”. In this work we use a localization toolbox to derive risk bounds in two specific applications. The first is in portfolio risk minimization with conditional value-at-risk constraints. We consider a setting where, among all assets with high returns, there is a portion of dimension g , unknown to the investor, that has significant less risk than the other remaining portion. Our rates for the SAA problem show that “risk inflation”, caused by a multiplicative factor, affects the statistical rate only via a term proportional to g . As the “normalized risk” increases, the contribution in the rate from the extrinsic dimension diminishes while the dependence on g is kept fixed. Localization is a key tool to show this property. As a second application of our localization toolbox, we obtain sharp oracle inequalities for least-squares estimators with a Lasso-type constraint under weak moment assumptions. One main consequence of these inequalities is to obtain persistence , as posed by Greenshtein and Ritov, with covariates having heavier tails. This gives improvements in prior work of Bartlett, Mendelson and Neeman.
更多查看译文
关键词
90C15,90C31,60E15,60F10
AI 理解论文
溯源树
样例
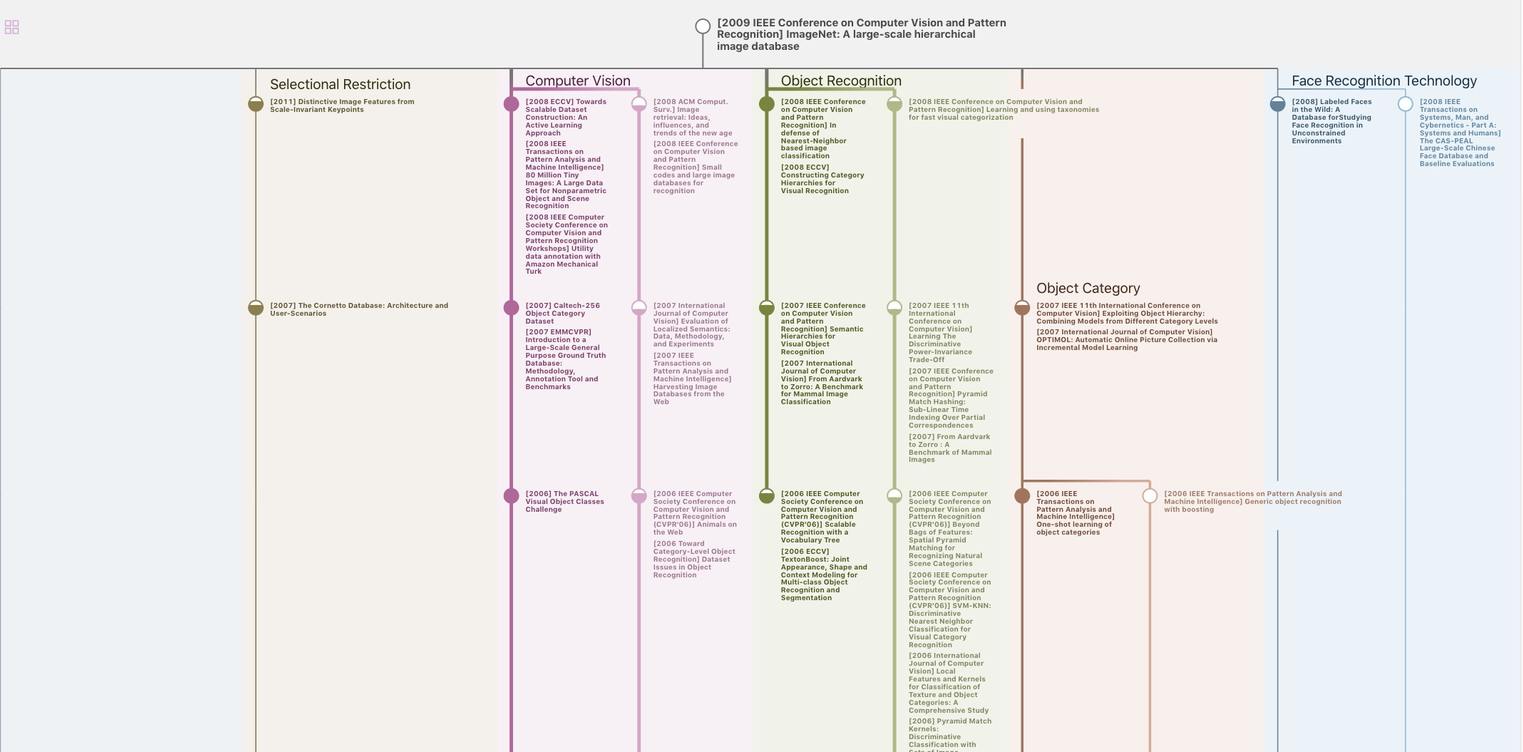
生成溯源树,研究论文发展脉络
Chat Paper
正在生成论文摘要