Deeply fusing multimodal features in hypergraph
Multimedia Tools and Applications(2020)
摘要
Utilizing multimodal features to describe multimedia data is a natural way to improve recognition accuracy. However, how to optimally cluster the raw features into different modalities in order to alleviate curse of dimension and how to exploit relationships between and within the feature modalities are still two tough issues. In this paper, we propose a new deep feature fusion framework: hypergraph feature fusion (HFF), to handle these two issues. First, we extract a collection of deep features from multiple images, then HFF constructs a features’ relationships hypergraph (FRH) to reveal relationships among raw features. Then HFF conducts generalized community learning by graph approximation (GCLGA) in FRH to cluster the raw features into k modalities and obtain the inter and intra modalities’ structure matrices. These matrices reveal relationships of inter and intra modalities and can help to build graph kernels in order to optimize kernel based classification. Finally, HFF applies a two level classifier to classify the fused feature vectors. Dimension of each level classifier’s input feature vector is much lower than raw feature vector. We conduct the kernel based classification on two experiments: 1) Using kernel SVM to classify ETH-80 image dataset by fusing 2 kinds of raw image features. 2) Using features extracted from kernel LDA on speech emotion recognition by fusing 6 kinds of raw speech features. The experimental result shows HFF can effectively solve these two issues and improve class-prediction accuracy over state-of-art feature fusion techniques.
更多查看译文
关键词
Deeply fusing, Multimodal, Hypergraph
AI 理解论文
溯源树
样例
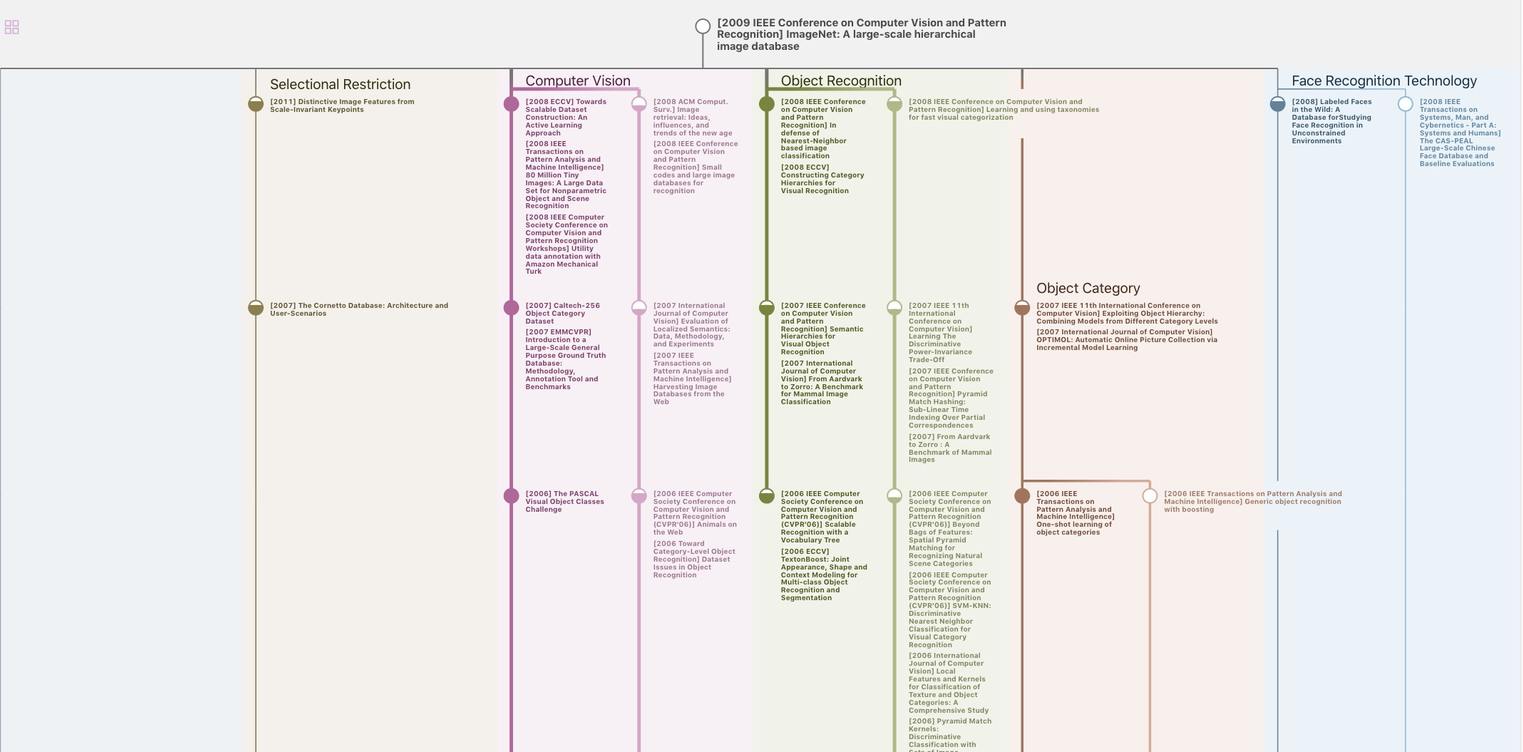
生成溯源树,研究论文发展脉络
Chat Paper
正在生成论文摘要