Unsupervised On-Line Selection of Training Features for a robust classification with drifting and faulty gas sensors
SENSORS AND ACTUATORS B-CHEMICAL(2018)
摘要
Inconstancy and scarce reproducibility limit the online application of chemical sensor arrays. Most of the attempts to counteract these issues are based on adaptive classifiers, namely models that also change to keep pace with fluctuations in the information content. In this paper, we introduce a decision making process to remove ambiguous components from the input data. This approach provides robustness towards fault and drift and, at the same time, maintains the use of standard classifiers. The heart of the method is characterized by an online feature selection for single test measurements. With the selected features, a completely new model is trained to classify the sample. After the class assignment, the dataset used for the model training is updated exploiting the most recent measurements. The algorithm of feature selection has been applied to a number of standard classifiers such as k-NN, PLS-DA and LDA. Synthetic and real sensors dataset have been used to validate the method. In particular synthetic data have been used to test the algorithm in complex drifting sensors scenario and real data provided the basis to study the performance of feature selection procedure. Fault sensors events were included in each dataset. Results confirm an excellent capability to counteract drift and to identify and exclude those features that are either temporarily or permanently faulting. (C) 2017 Elsevier B.V. All rights reserved.
更多查看译文
关键词
On-line feature selection,Adaptive classifier,Drift counteraction,Fault tolerance,Gas sensors array
AI 理解论文
溯源树
样例
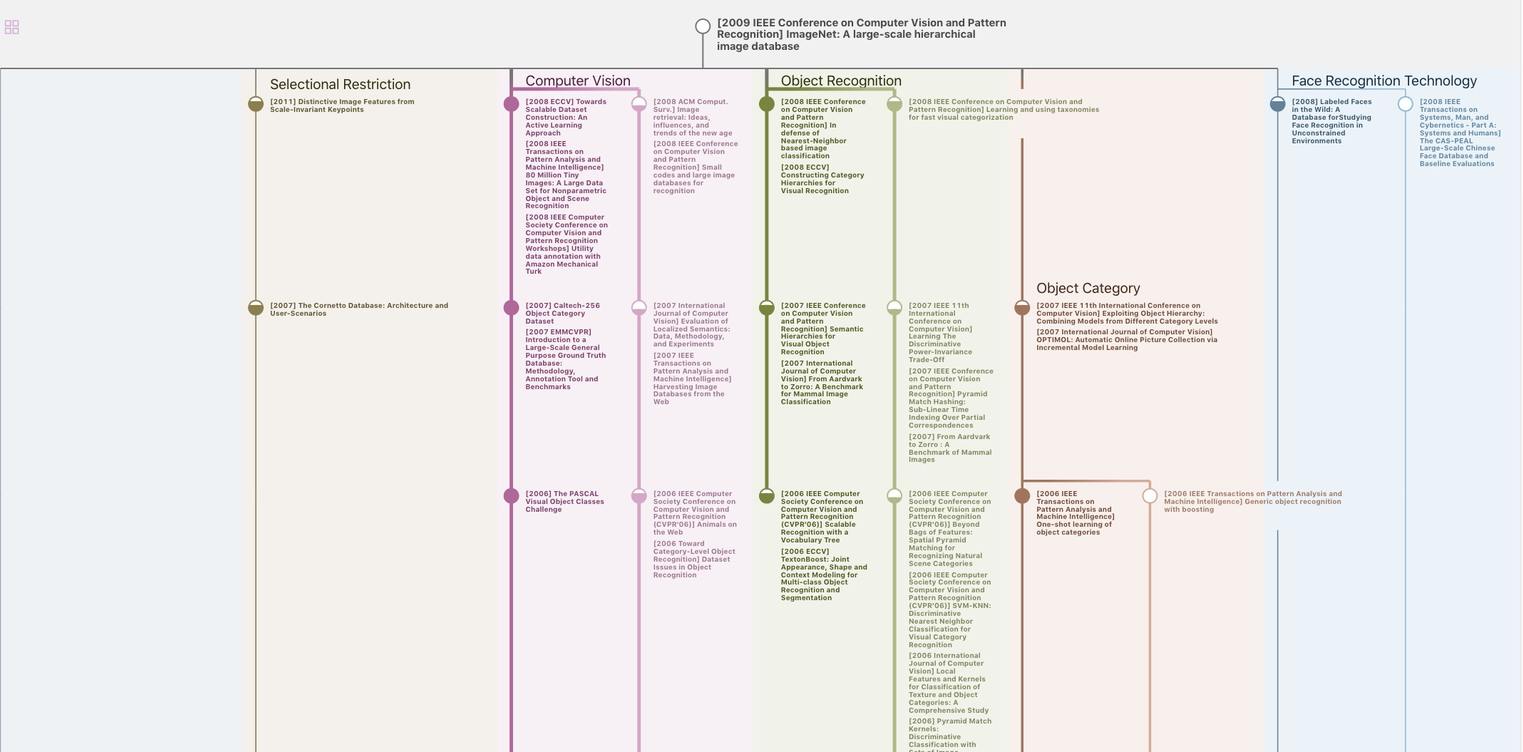
生成溯源树,研究论文发展脉络
Chat Paper
正在生成论文摘要