Ensemble candidate classification for the LOTAAS pulsar survey
MONTHLY NOTICES OF THE ROYAL ASTRONOMICAL SOCIETY(2018)
摘要
One of the biggest challenges arising from modern large-scale pulsar surveys is the number of candidates generated. Here, we implemented several improvements to the machine learning (ML) classifier previously used by the LOFAR Tied-Array All-Sky Survey (LOTAAS) to look for new pulsars via filtering the candidates obtained during periodicity searches. To assist the ML algorithm, we have introduced new features which capture the frequency and time evolution of the signal and improved the signal-to-noise calculation accounting for broad profiles. We enhanced the ML classifier by including a third class characterizing RFI instances, allowing candidates arising from RFI to be isolated, reducing the false positive return rate. We also introduced a new training data set used by the ML algorithm that includes a large sample of pulsars misclassified by the previous classifier. Lastly, we developed an ensemble classifier comprised of five different Decision Trees. Taken together these updates improve the pulsar recall rate by 2.5 per cent, while also improving the ability to identify pulsars with wide pulse profiles, often misclassified by the previous classifier. The new ensemble classifier is also able to reduce the percentage of false positive candidates identified from each LOTAAS pointing from 2.5 per cent (similar to 500 candidates) to 1.1 per cent (similar to 220 candidates).
更多查看译文
关键词
pulsars: general,methods: data analysis,methods: statistical
AI 理解论文
溯源树
样例
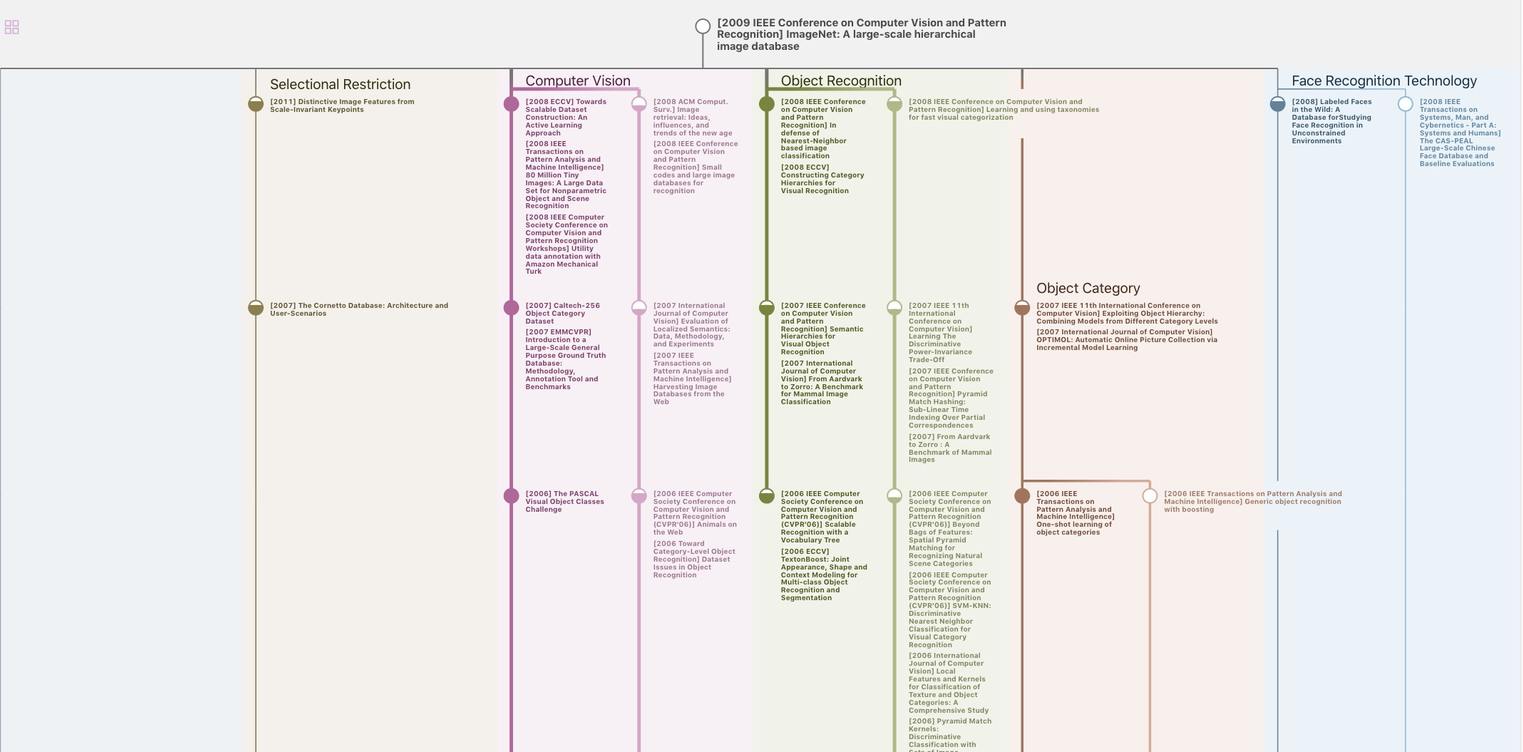
生成溯源树,研究论文发展脉络
Chat Paper
正在生成论文摘要