Late Breaking Abstract - Functional Respiratory Imaging (Fri) And Machine Learning To Predict Organ Rejection Shortly After Lung Transplantation
EUROPEAN RESPIRATORY JOURNAL(2017)
摘要
Introduction: Chronic rejection after lung transplant remains a challenge due to the lack of sensitive diagnostics. Aims and objectives: To assess combination of FRI and machine learning to predict the probability of Bronchiolitis Obliterans Syndrome (BOS). Methods: In this study 41 transplant patients were studied. 15 patients developed BOS while 26 patients remained BOS free. FRI and machine learning was used to find predictors of BOS in the first inspiratory/expiratory HRCT scans after transplant at a time where no clinical signs of BOS were present. Results: BOS is characterized by a regional manifestation of the disease, highlighting the need for a diagnostic that yields regional information on lung structure and function. Patients with smaller lung volumes (pu003c0.001), smaller airway volumes (p=0.01) and surface (p=0.04) and higher airway resistance (p=0.03) had more chance of developing BOS. A combination of lobar resistance, surface area of the central airways and regional lobe volumes could predict chronic rejection with an accuracy of 85% as determined by Support Vector Machines (Figure 1). Figure 1: Functional Residual Imaging parameters that could predict onset of BOS with accuracy of 85% Conclusion: FRI and machine learning provides a sensitive diagnostic tool with a high potential to optimize the treatment paradigm post lung transplantation.
更多查看译文
AI 理解论文
溯源树
样例
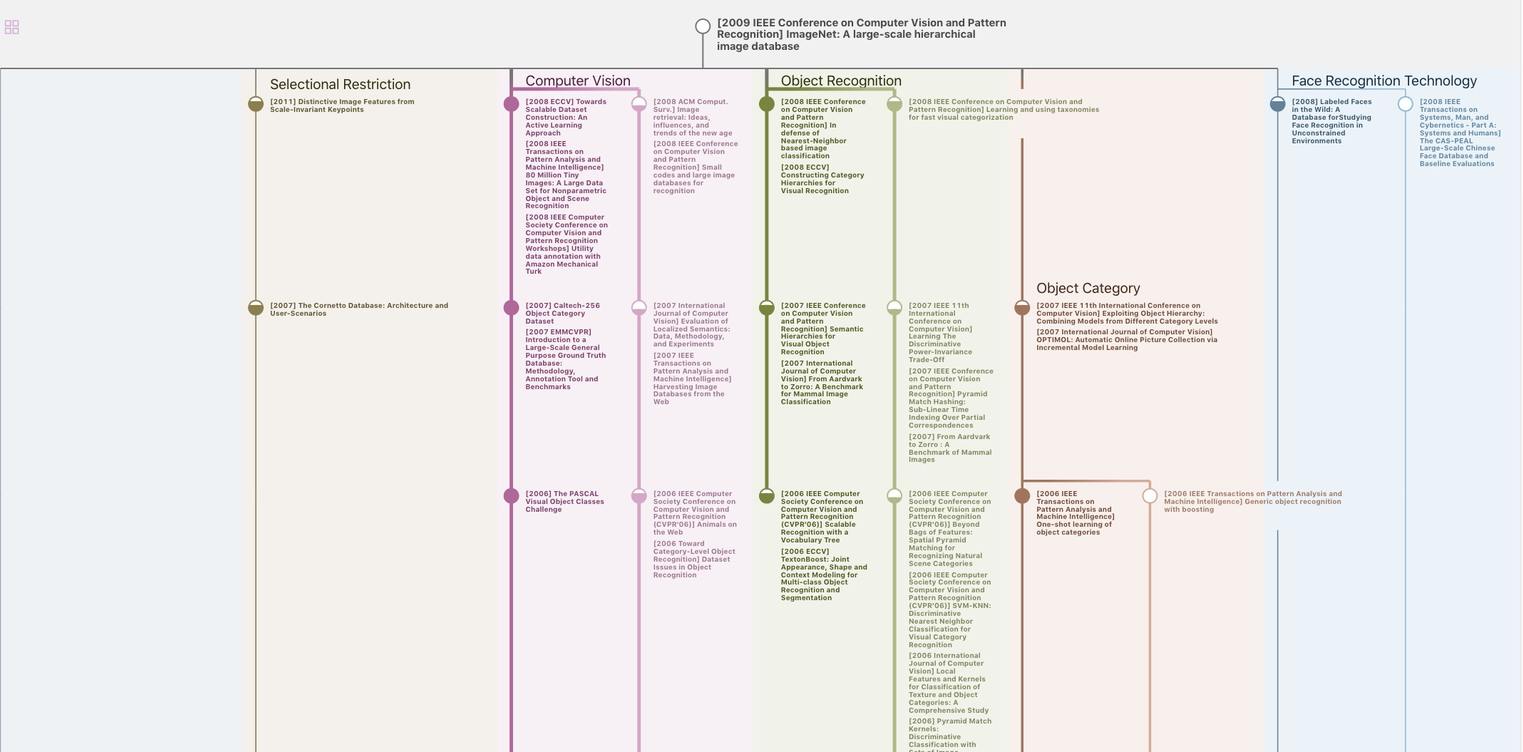
生成溯源树,研究论文发展脉络
Chat Paper
正在生成论文摘要