Parallel multi-stage features fusion of deep convolutional neural networks for aerial scene classification
REMOTE SENSING LETTERS(2018)
摘要
Aerial scene classification is a challenging task in the remote sensing image processing field. Owing to some similar scene, there are only differences in density. To challenge this problem, this paper proposes a novel parallel multi-stage (PMS) architecture formed by a low, middle, and high deep convolutional neural network (DCNN) sub-model. PMS model automatically learns representative and discriminative hierarchical features, which include three 512 dimension vectors, respectively, and the final representative feature created by linear connection. PMS model describes a robust feature of aerial image through three stages feature. Unlike previous methods, we only use transfer learning and deep learning methods to obtain more discriminative features from scene images while improving performance. Experimental results demonstrate that the proposed PMS model has a more superior performance than the state-of-the-art methods, obtaining average classification accuracies of 98.81% and 95.56%, respectively, on UC Merced (UCM) and aerial image dataset (AID) benchmark datasets.
更多查看译文
关键词
deep convolutional neural networks,convolutional neural networks,parallel,features,multi-stage
AI 理解论文
溯源树
样例
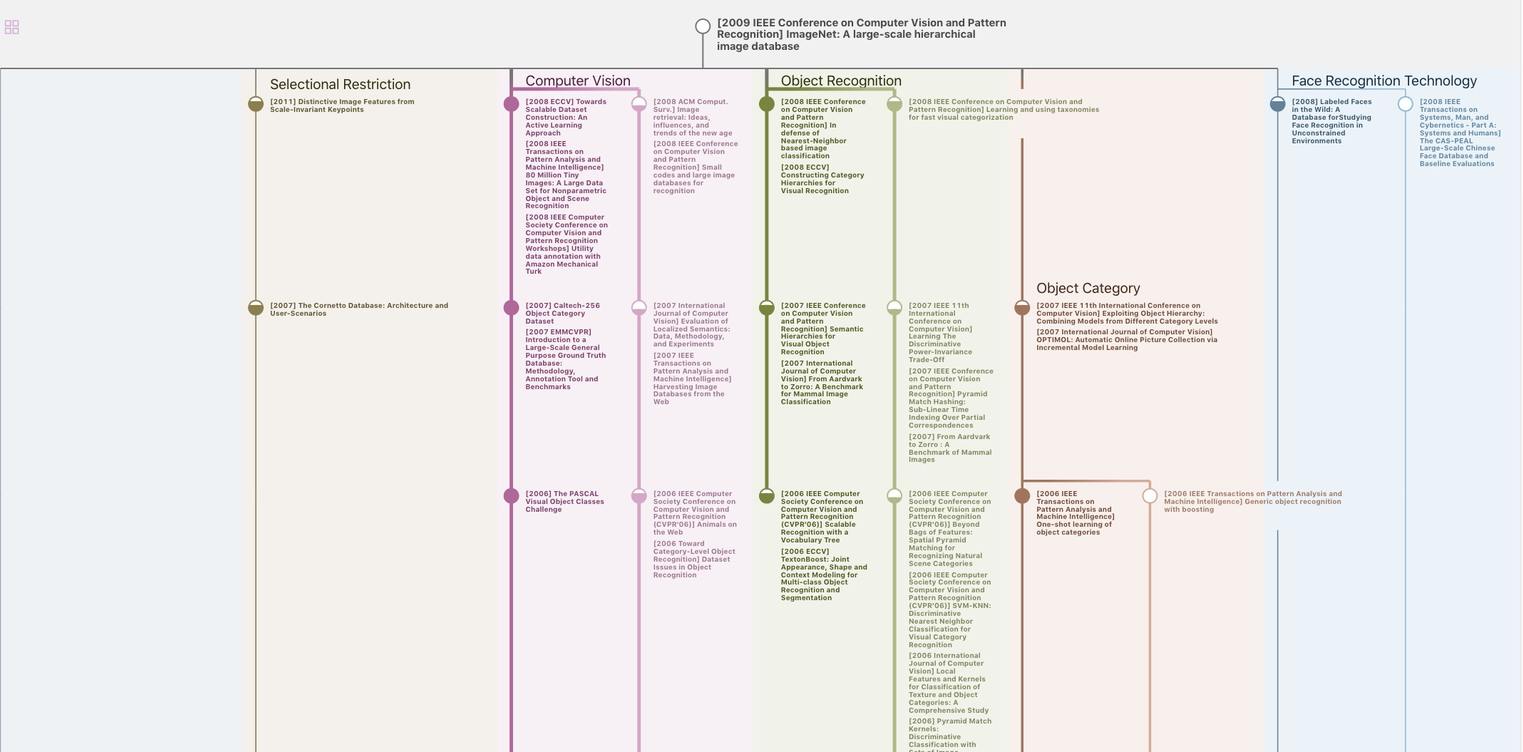
生成溯源树,研究论文发展脉络
Chat Paper
正在生成论文摘要