Punctuation prediction using a bidirectional recurrent neural network with part-of-speech tagging
TENCON IEEE Region 10 Conference Proceedings(2017)
摘要
Most automatic speech recognition (ASR) systems are incapable of generating punctuation, making it difficult to read the transcribed output and less appropriate for tasks such as dictation. This paper introduces a procedure to automatically insert punctuation into unpunctuated sentences by using a bidirectional recurrent neural network with attention mechanism and Part-of-Speech (POS) Tags. Using the WikiText Long Term Dependency Language Modelling Dataset and handling 11 different punctuation symbols, the model managed to achieve a punctuation error rate of 31.4% and an F1 score of 785%. When the system was trained on consecutive sentences and a smaller dataset using the Europarl v7 corpus, the model still managed to achieve a punctuation error rate of 48.1% and an F1 score of 64.7%. In both cases, our proposed system outperforms previous state-of-the-art systems trained on the same datasets, showing the advantage of using POS tags information and an encoder decoder network.
更多查看译文
关键词
deep neural networks,punctuation prediction,sequence-to-sequence approach
AI 理解论文
溯源树
样例
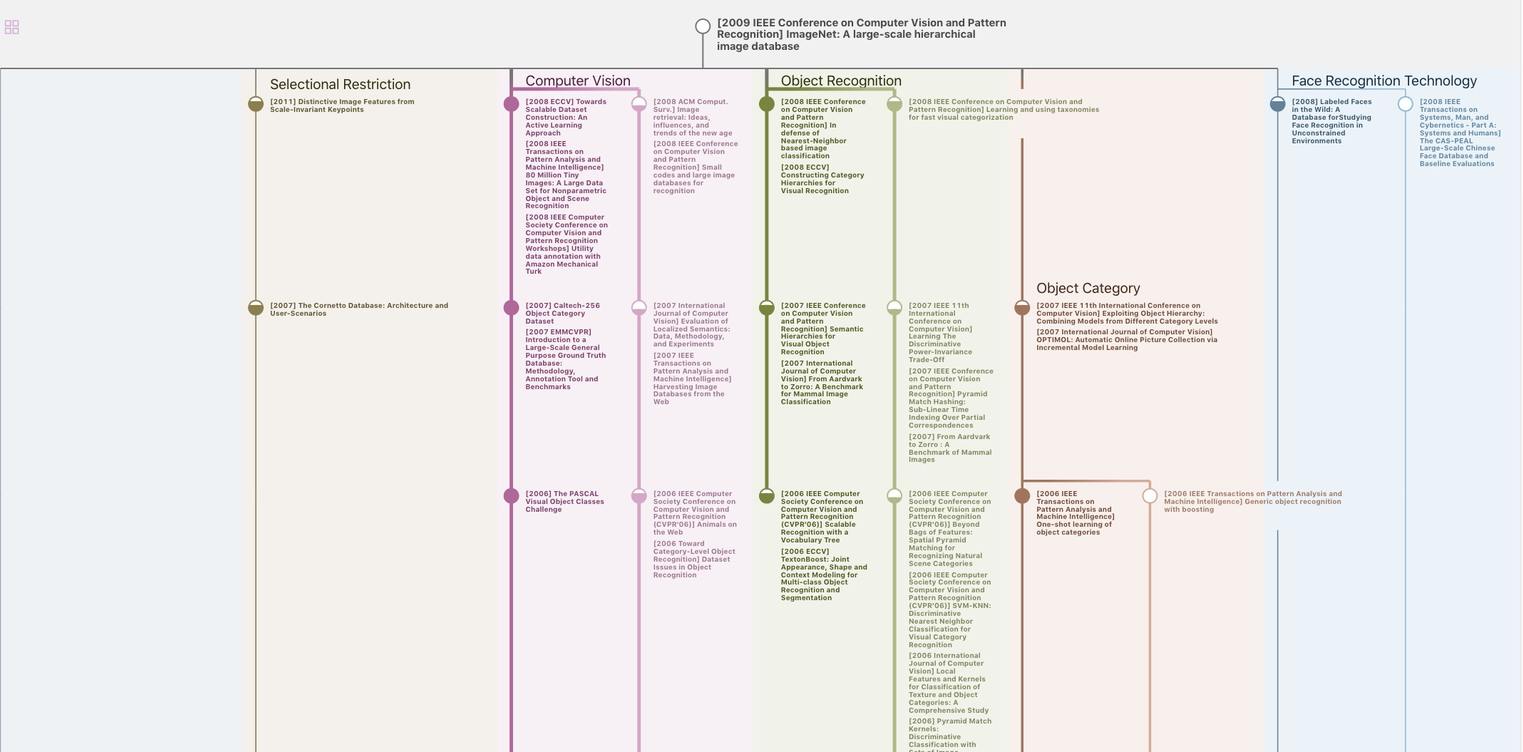
生成溯源树,研究论文发展脉络
Chat Paper
正在生成论文摘要