Short-term traffic flow prediction using a methodology based on ARIMA and RBF-ANN
2017 CHINESE AUTOMATION CONGRESS (CAC)(2017)
摘要
The accurate short-term traffic flow forecasting is fundamental to both theoretical and empirical aspects of intelligent transportation systems deployment. In order to play the ARIMA model with good linear fitting ability and artificial neural network model with strong nonlinear relation mapping ability, this study aimed to develop a simple and effective hybrid model for forecasting traffic volume that combines the AutoRegressive Integrated Moving Average (ARIMA) and the Radial Basis Function Artificial Neural Networks (RBF-ANN) models. By combining different models, different aspects of the underlying patterns of traffic flow could be captured. The ARIMA model was used to model the linear component of the traffic flow time series. Then the RBF-ANN model was applied to capture the nonlinear component by modelling the residuals from the ARIMA model. The hybrid models were fitted for five minutes time-aggregations. The validations of the proposed hybrid methodology were performed by using traffic data from Shinan Avenue in Nansha District, Guangzhou, China. The results indicated that the hybrid models had better predictive performance than utilizing only ARIMA model as well as RBF-ANN model. The combination method played the advantages of the two models is an effective method for short-term traffic flow forecasting.
更多查看译文
关键词
short-term traffic flow forecasting, ARIMA model, artificial neural network, RBF, hybrid model
AI 理解论文
溯源树
样例
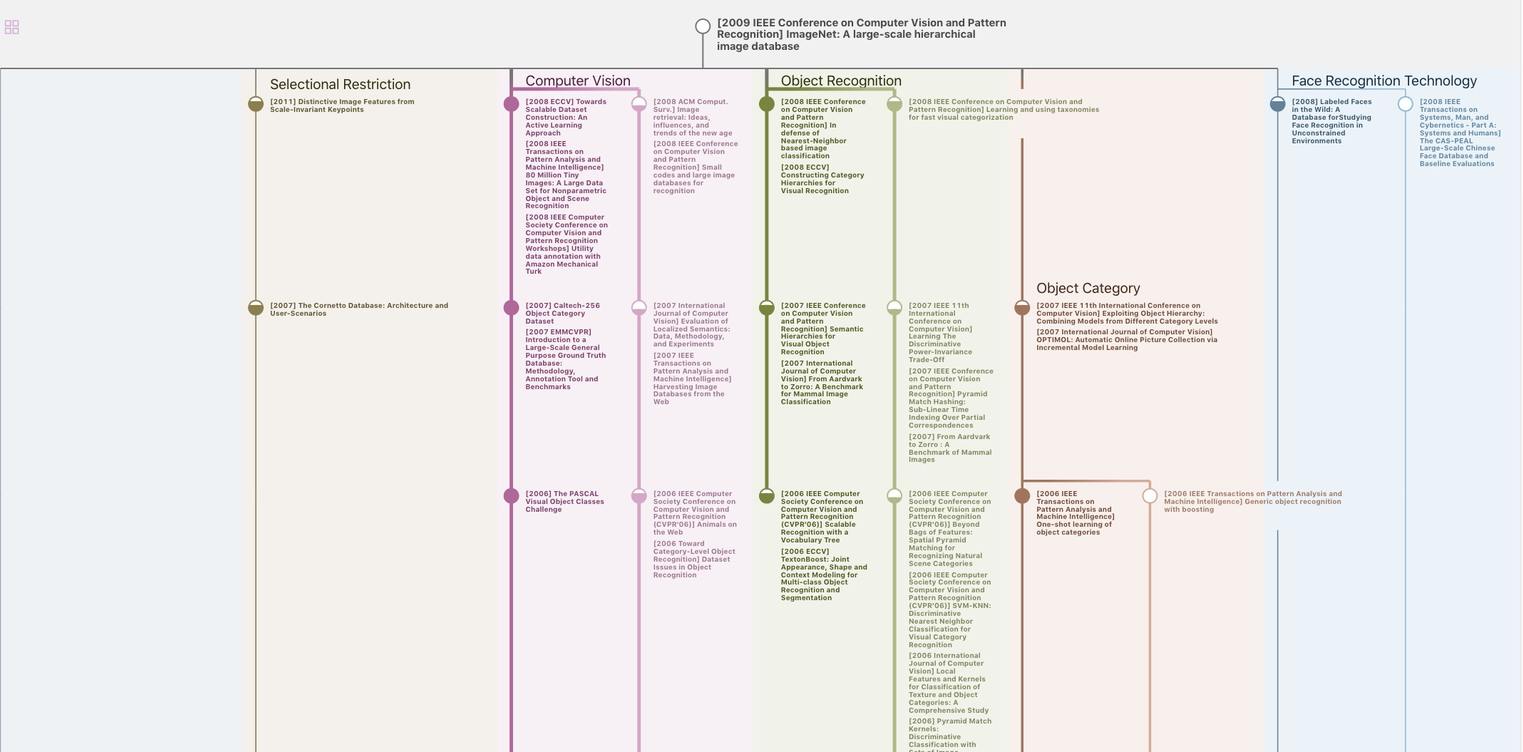
生成溯源树,研究论文发展脉络
Chat Paper
正在生成论文摘要