Cellular automata rule characterization and classification using texture descriptors
Physica A: Statistical Mechanics and its Applications(2018)
摘要
The cellular automata (CA) spatio-temporal patterns have attracted the attention from many researchers since it can provide emergent behavior resulting from the dynamics of each individual cell. In this manuscript, we propose an approach of texture image analysis to characterize and classify CA rules. The proposed method converts the CA spatio-temporal patterns into a gray-scale image. The gray-scale is obtained by creating a binary number based on the 8-connected neighborhood of each dot of the CA spatio-temporal pattern. We demonstrate that this technique enhances the CA rule characterization and allow to use different texture image analysis algorithms. Thus, various texture descriptors were evaluated in a supervised training approach aiming to characterize the CA’s global evolution. Our results show the efficiency of the proposed method for the classification of the elementary CA (ECAs), reaching a maximum of 99.57% of accuracy rate according to the Li–Packard scheme (6 classes) and 94.36% for the classification of the 88 rules scheme. Moreover, within the image analysis context, we found a better performance of the method by means of a transformation of the binary states to a gray-scale.
更多查看译文
关键词
Elementary cellular automata,Texture analysis
AI 理解论文
溯源树
样例
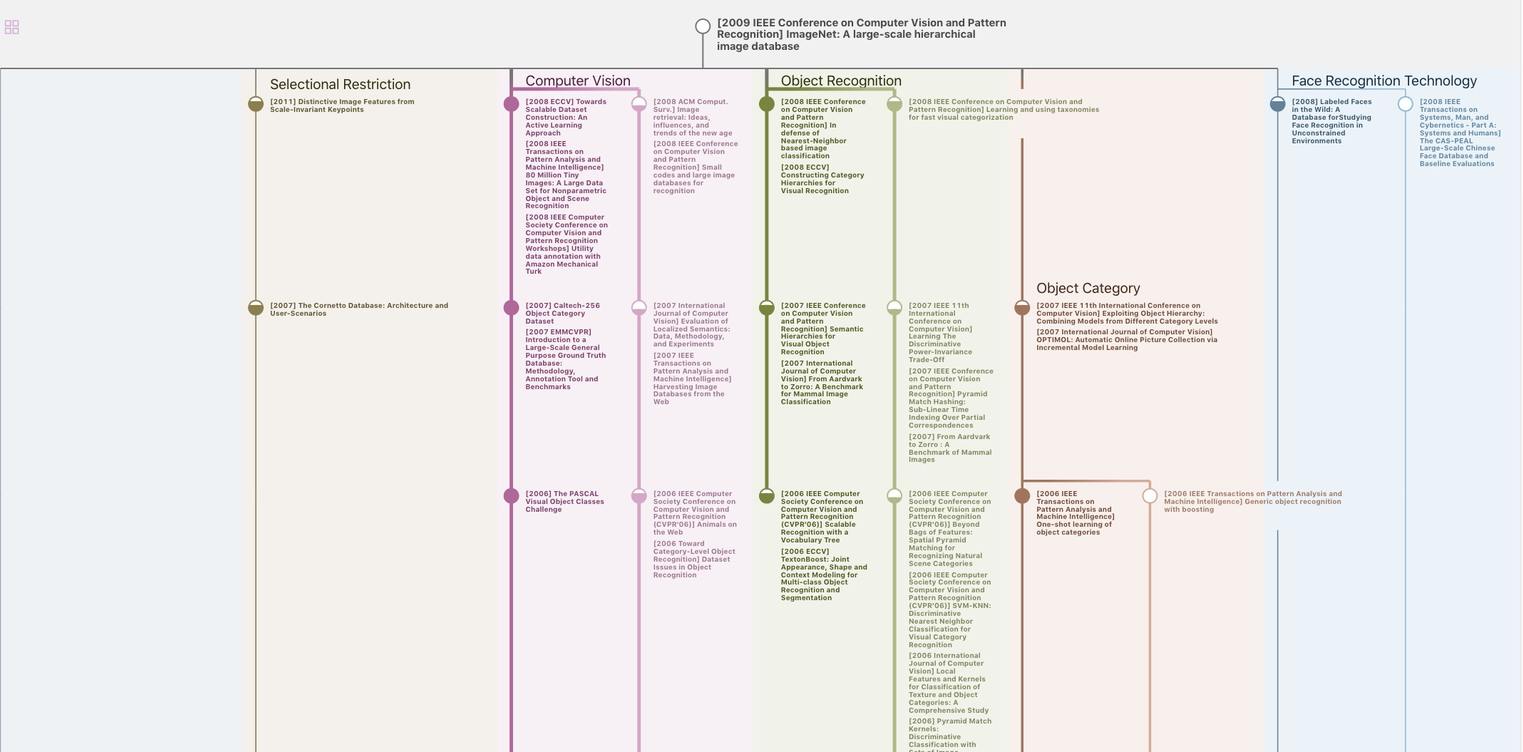
生成溯源树,研究论文发展脉络
Chat Paper
正在生成论文摘要