Improving text relatedness by incorporating phrase relatedness with word relatedness: Text Relatedness using Google Tri-grams
COMPUTATIONAL INTELLIGENCE(2018)
摘要
Text is composed of words and phrases. In the bag-of-words model, phrases in text are split into words. This may discard the semantics of phrases, which, in turn, may give an inconsistent relatedness score between 2 texts. Our objective is to apply phrase relatedness in conjunction with word relatedness on the text relatedness task to improve text relatedness performance. We adopt 2 existing word relatedness measures based on Google n-gram and Global Vectors for Word Representation, respectively, and incorporate them differently with an existing Google n-gram-based phrase relatedness method to compute text relatedness. The combination of Google n-gram-based word and phrase relatedness performs better than Google n-gram-based word relatedness alone, by achieving the higher weighted mean of Pearson's r, ie, 0.639 and 0.619, respectively, on the 14 data sets from the series of Semantic Evaluation workshops SemEval-2012, SemEval-2013, and SemEval-2015. Similarly, the combination of GloVe-based word relatedness and Google n-gram-based phrase relatedness performs better than GloVe-based word relatedness alone, by achieving the higher weighted mean of Pearson's r, ie, 0.619 and 0.605, respectively, on the same 14 data sets. On the SemEval-2012, SemEval-2013, and SemEval-2015 data sets, the text relatedness results obtained from the combination of Google n-gram-based word and phrase relatedness ranked 24, 3, and 31 out of 89, 90, and 73 text relatedness systems, respectively.
更多查看译文
关键词
semantic relatedness,semantic similarity,text mining,text relatedness,text similarity
AI 理解论文
溯源树
样例
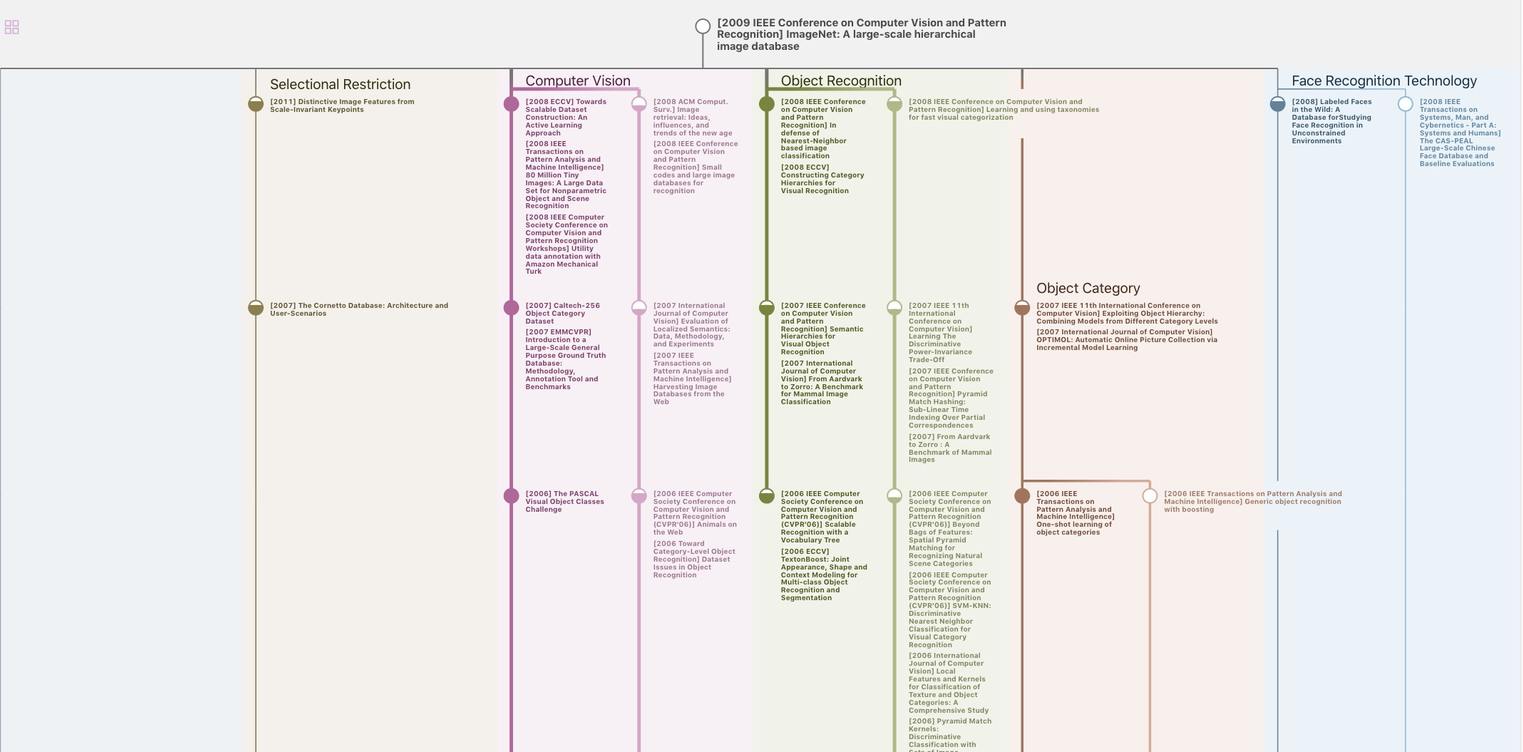
生成溯源树,研究论文发展脉络
Chat Paper
正在生成论文摘要