Context-specific interaction networks from vector representation of words
arXiv: Molecular Networks(2019)
摘要
The number of biomedical publications has grown steadily in recent years. However, most biomedical facts are not readily available, but buried in the form of unstructured text. Here we present INtERAcT, an unsupervised method to extract interactions from a corpus of biomedical articles. INtERAcT exploits a vector representation of words, computed on a corpus of domain-specific knowledge, and implements a new metric that estimates an interaction score between two molecules in the space where the corresponding words are embedded. We use INtERAcT to reconstruct the molecular pathways of 10 different cancer types using corpora of disease-specific articles, considering the STRING database as a benchmark. Our metric outperforms currently adopted approaches and it is highly robust to parameter choices, leading to the identification of known molecular interactions in all studied cancer types. Furthermore, our approach does not require text annotation, manual curation or the definition of semantic rules based on expert knowledge, and can therefore be efficiently applied to different scientific domains.
更多查看译文
关键词
Literature mining,Machine learning,Network topology,Software,Engineering,general
AI 理解论文
溯源树
样例
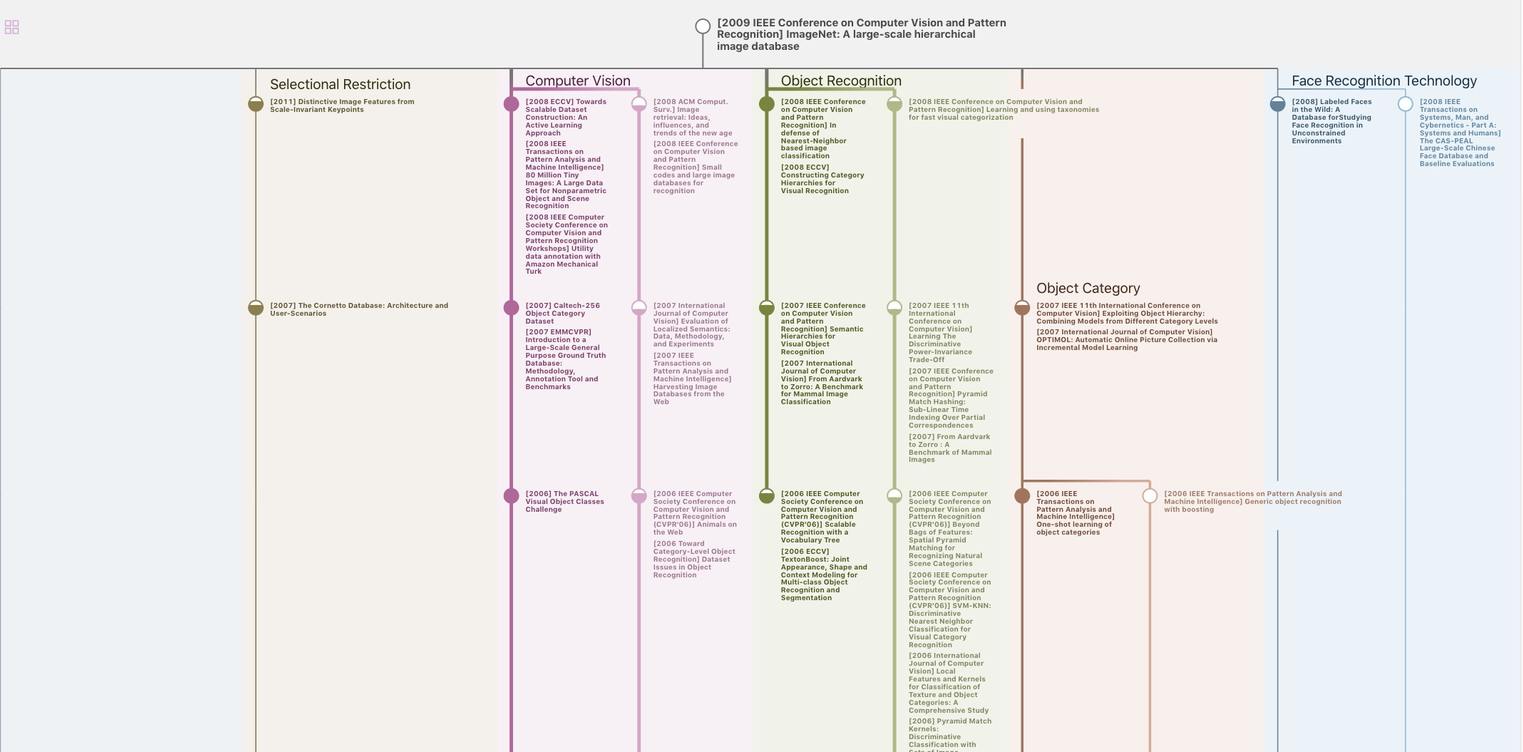
生成溯源树,研究论文发展脉络
Chat Paper
正在生成论文摘要