Local Stretch Similarity Measure And Auto Query Expansion For Re-Ranking Of Image Retrieval
INTERNATIONAL CONFERENCE ON COMPUTER SCIENCE AND APPLICATION ENGINEERING (CSAE)(2017)
摘要
Using local neighbors (contextual information) to measure similarities between images is an effective re-ranking method in image retrieval, which has many advantages (e.g., low time complexity, easy promotion for new images). However, traditional methods usually cannot take neighbors into account comprehensively for the influence of noises (the more neighbors chosen, the more noises involved). To solve this problem, we propose Local Stretch Similarity Measure algorithm (LSSM). LSSM chooses multiple layers of neighbors to measure similarities, through which more contextual information can be considered and less noises will be introduced. Furthermore, we propose Auto Query Expansion (AQE) re-ranking method to transform the original single-query problem to a multi-query problem. By means of AQE, the robustness of LSSM can be enhanced. Extensive experiments are conducted on Corel-1K, Corel-10K and UK-bench datasets. Experimental results validate that our methods outperform other state-of-the-art methods.
更多查看译文
AI 理解论文
溯源树
样例
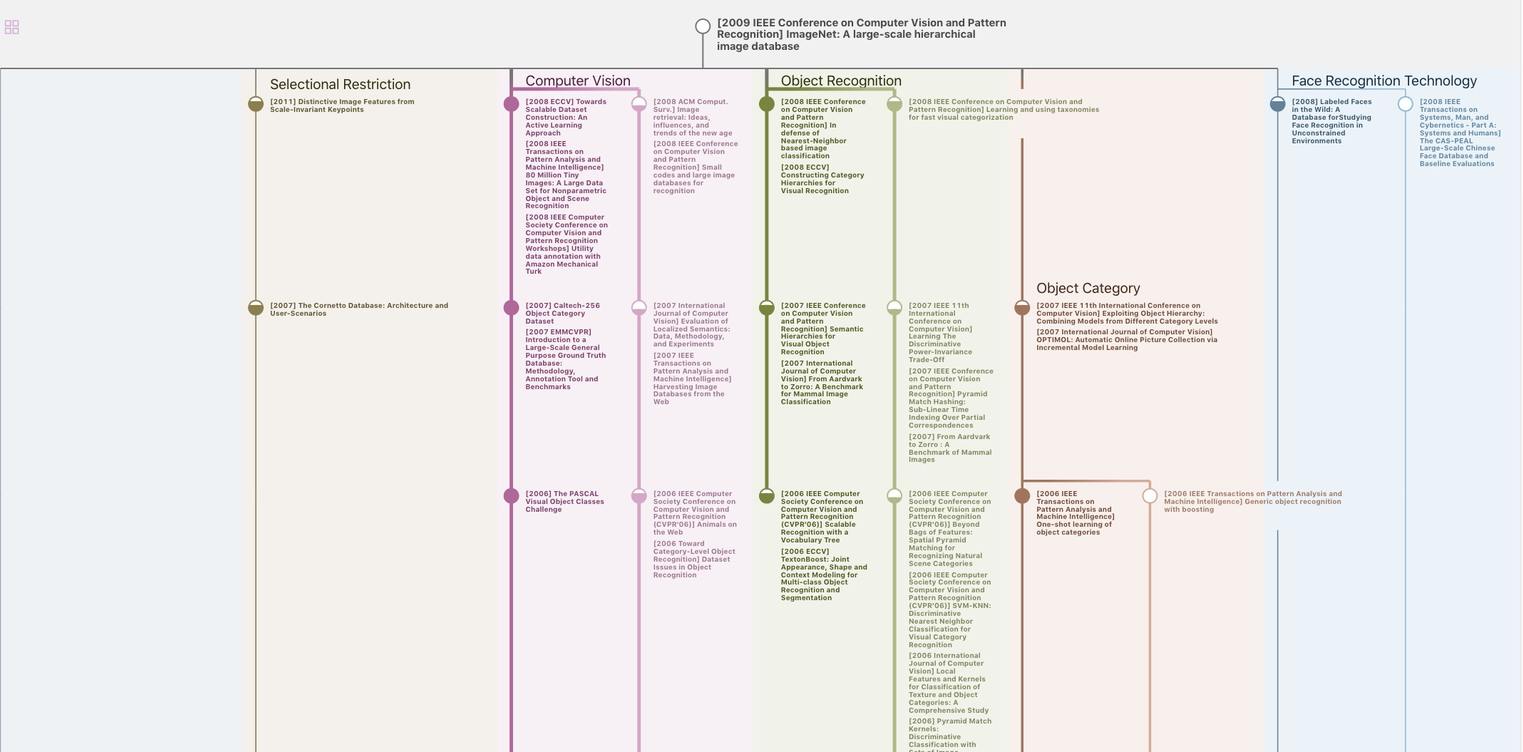
生成溯源树,研究论文发展脉络
Chat Paper
正在生成论文摘要