Performance Evaluation of Filter-based Feature Selection Techniques in Classifying Portable Executable Files
Procedia Computer Science(2018)
摘要
The dimensionality of the feature space exhibits a significant effect on the processing time and predictive performance of the Malware Detection Systems (MDS). Therefore, the selection of relevant features is crucial for the classification process. Feature Selection Technique (FST) is a prominent solution that effectively reduces the dimensionality of the feature space by identifying and neglecting noisy or irrelevant features from the original feature space. The significant features recommended by FST uplift the malware detection rate. This paper provides the performance analysis of four chosen filter-based FSTs and their impact on the classifier decision. FSTs such as Distinguishing Feature Selector (DFS), Mutual Information (MI), Categorical Proportional Difference (CPD), and Darmstadt Indexing Approach (DIA) have been used in this work and their efficiency has been evaluated using different datasets, various feature-length, classifiers, and success measures. The experimental results explicitly indicate that DFS and MI offer a competitive performance in terms of better detection accuracy and that the efficiency of the classifiers does not decline on both the balanced and unbalanced datasets.
更多查看译文
关键词
Feature Selection Technique,Malware,Malware Detection System,Machine Learning,Portable Executable Files
AI 理解论文
溯源树
样例
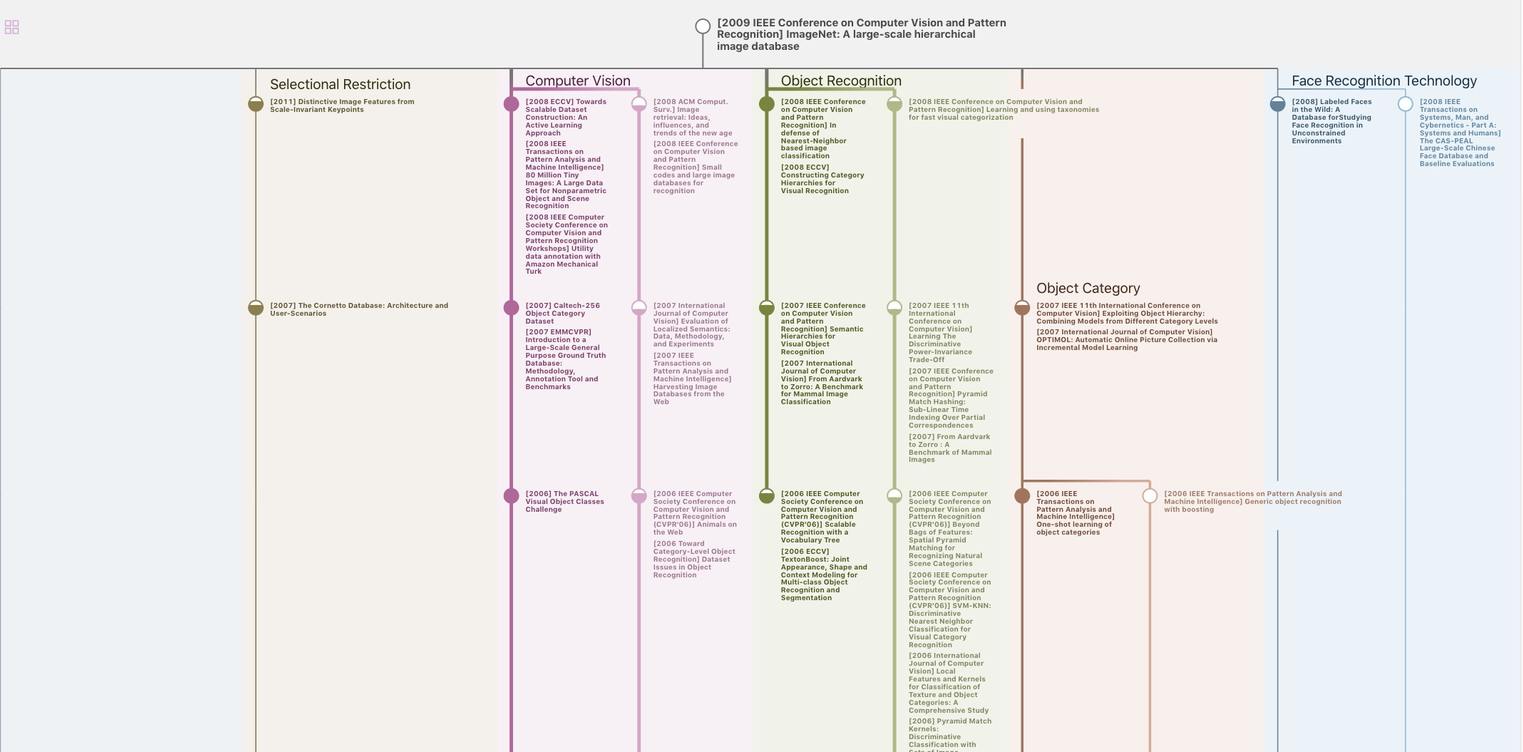
生成溯源树,研究论文发展脉络
Chat Paper
正在生成论文摘要