Computational Analysis of Genomic Abnormalities from a Phase 3 Trial of Rigosertib in Higher-Risk MDS: Simulation of a Predictive Signature for Clinical Response
Blood(2016)
摘要
Background: MDS is a marrow stem cell disorder with limited treatments. Due to outcome heterogeneity of MDS, it is imperative to identify prognostic tools for patients in clinical trials. Rigosertib (RIG) is a RAS-mimetic that inhibits cellular signaling pathways by binding to the RAS-binding Domain found in RAS effector proteins. No approved treatment options are available for Higher-risk myelodysplastic syndromes (HR-MDS) pts after Hypomethylating Agent (HMA) failure. The trial was a Phase III, randomized study of rigosertib in a heterogeneous population of HR-MDS pts who failed to respond to or progressed on HMAs. Aim: To determine the predictive value of a genomics-informed computational biology method and to design a patient selection signature in HR-MDS patients who are treated with RIG. Methods: ONTIME trial included HR-MDS pts who had progressed on (37% of total enrollment), failed to respond to (25%), or relapsed after (38%) HMA treatment. Patients were randomized 2:1 to receive RIG (199 pts) or best supportive care (BSC) (100 pts) (Garcia-Manero et al, Lancet Oncology, 2016).The primary endpoint was overall survival, analyzed on an intention-to-treat basis using the Kaplan-Meier method. Genomic DNA was isolated from single microscopic slides from 153 pts and subjected to sequence analysis of a myeloid panel comprising of 24 selected loci frequently mutated in MDS and AML. Standardized cytogenetic investigations were performed. The chromosome aberrations and clone definition followed the International System for Cytogenetic Nomenclature. Depending on the aberrations detected during karyotyping, further probes were applied. A complex karyotype was defined as ≥3 independent aberrations within 1 clone. These genomic results were input into predictive computational biology software (Cellworks Group, Fig. 1), which generates disease-specific dysregulated protein network maps using PubMed and other resources. Digital drug simulations are conducted by quantitatively measuring drug effect on a cell growth score, which is a composite of cell proliferation, viability and apoptosis. Each patient-specific protein network map was digitally screened for the extent by which RIG reduced disease growth in a dose-respondent manner. Clinical outcomes were prospectively recorded. Results: Based on input data from cell-culture studies the predicted response was blindly correlated with the clinical outcome for 54 patients with the following predictive test statistics: positive predictive value (PPV) - 85%, negative predictive value (NPV) - 94.12%, Sensitivity - 89.47%, Specificity - 91.43% and an accuracy of the predictive test at 90.74%. The accuracy of the genomics-informed computer method was significantly greater than empiric drug administration. New genomic signature rules were discovered to correlate with clinical response after RIG treatment. The predictive computational analysis identified a novel signature for selecting HR-MDS patient9s responding to RIG. We identified patients with either trisomy 8 or trisomy 21 that also do not have any of the following aberrations including: Del 5q or Del 7/7q or Del 3 or Del 14 or Del 16 or Del 20, would be responders to the drug (Trisomy 8 OR Trisomy 21) AND NOT (Del 5 q OR (Del 7/7q OR Del 3 OR Del 14 or Del 16 or Del 20). We validated this patient selection rule prospectively via statistical correlation methods and through a computer based simulation of a clinical trial generated predicted Kaplan-Meir curve with a significant p-value of 0.003 (Fig. 2). Conclusions: A predictive computational method that models multiple high-risk MDS genomic abnormalities simultaneously showed greater than 90% correlation between protein network perturbations and clinical outcomes. The computational method also helps explain reasons for lack of response to RIG and highlights resistance pathways that could be targeted to recover chemo-sensitivity. This technology also established eligibility criteria for precision enrollment in drug development trials. Disclosures Petrone: Onconova Therapeutics, Inc.: Employment. Fruchtman: Onconova: Employment. Brownstein: Onconova Therapeutics, Inc.: Consultancy. Loferer: Onconova Therapeutics, Inc.: Employment. Azarnia: Onconova Therapeutics, Inc.: Employment. Vali: Cellworks Group: Employment. Singh: Cellworks Group: Employment. Usmani: Cellworks: Employment. Grover: Cellworks Group: Employment. Abbasi: Cellworks: Employment. Silverman: Onconova Therapeutics, Inc.: Patents u0026 Royalties: Co-Patent holder for the combination of azacitidine and rigosertib, Research Funding.
更多查看译文
AI 理解论文
溯源树
样例
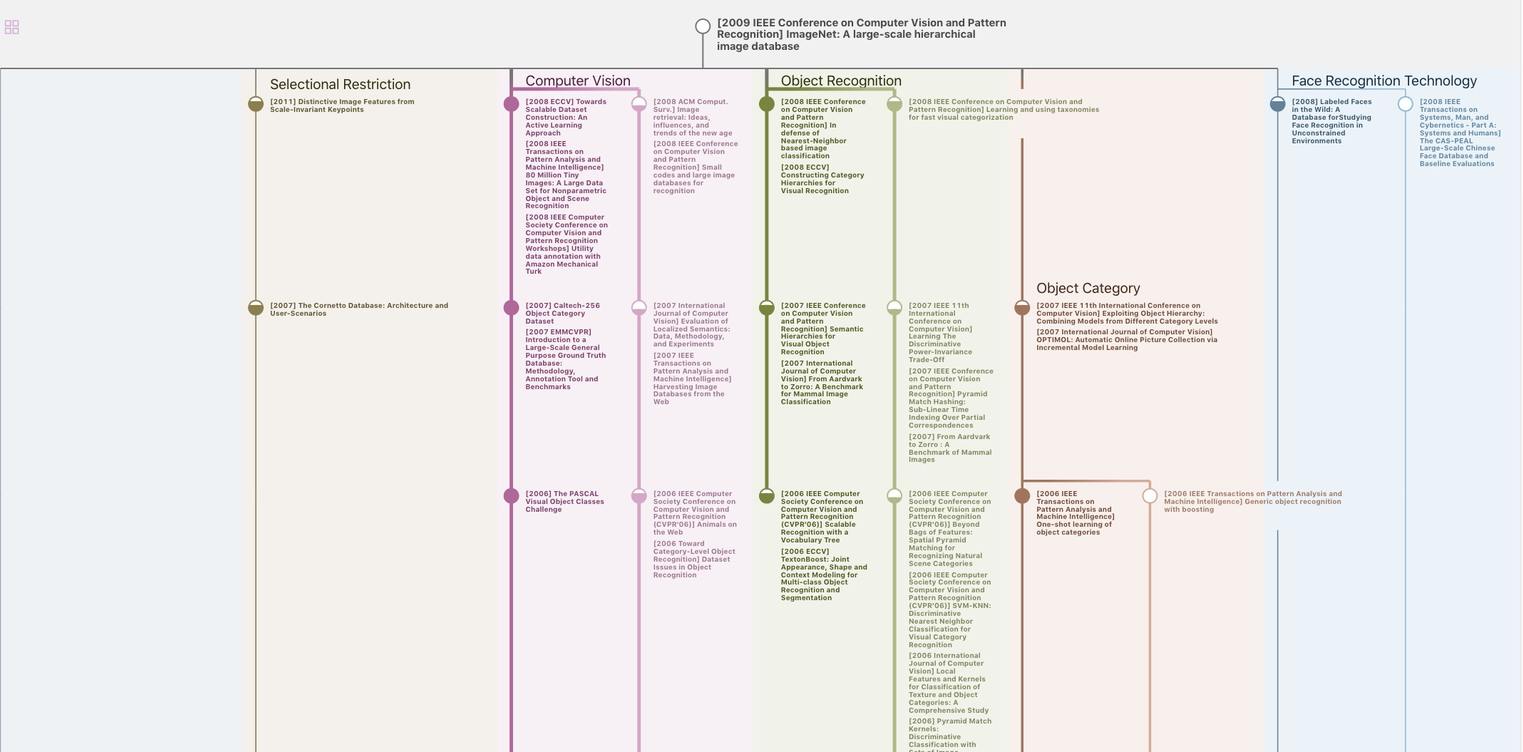
生成溯源树,研究论文发展脉络
Chat Paper
正在生成论文摘要