Challenges and opportunities toward online training acceleration using RRAM-based hardware neural network
2017 IEEE International Electron Devices Meeting (IEDM)(2017)
摘要
This paper highlights the feasible routes of using resistive memory (RRAM) for accelerating online training of deep neural networks (DNNs). A high degree of asymmetric nonlinearity in analog RRAMs could be tolerated when weight update algorithms are optimized with reduced training noise. Hybrid-weight Net (HW-Net), a modified multilayer perceptron (MLP) algorithm that utilizes hybrid internal analog and external binary weights is also proposed. Highly accurate online training could be realized using simple binary RRAMs that have already been widely developed as digital memory.
更多查看译文
关键词
online training acceleration,hardware neural network,resistive memory,deep neural networks,asymmetric nonlinearity,weight update algorithms,reduced training noise,hybrid-weight Net,HW-Net,modified multilayer perceptron algorithm,external binary weights,accurate online training,digital memory,DNN,analog RRAM,hybrid internal analog,simple binary RRAM
AI 理解论文
溯源树
样例
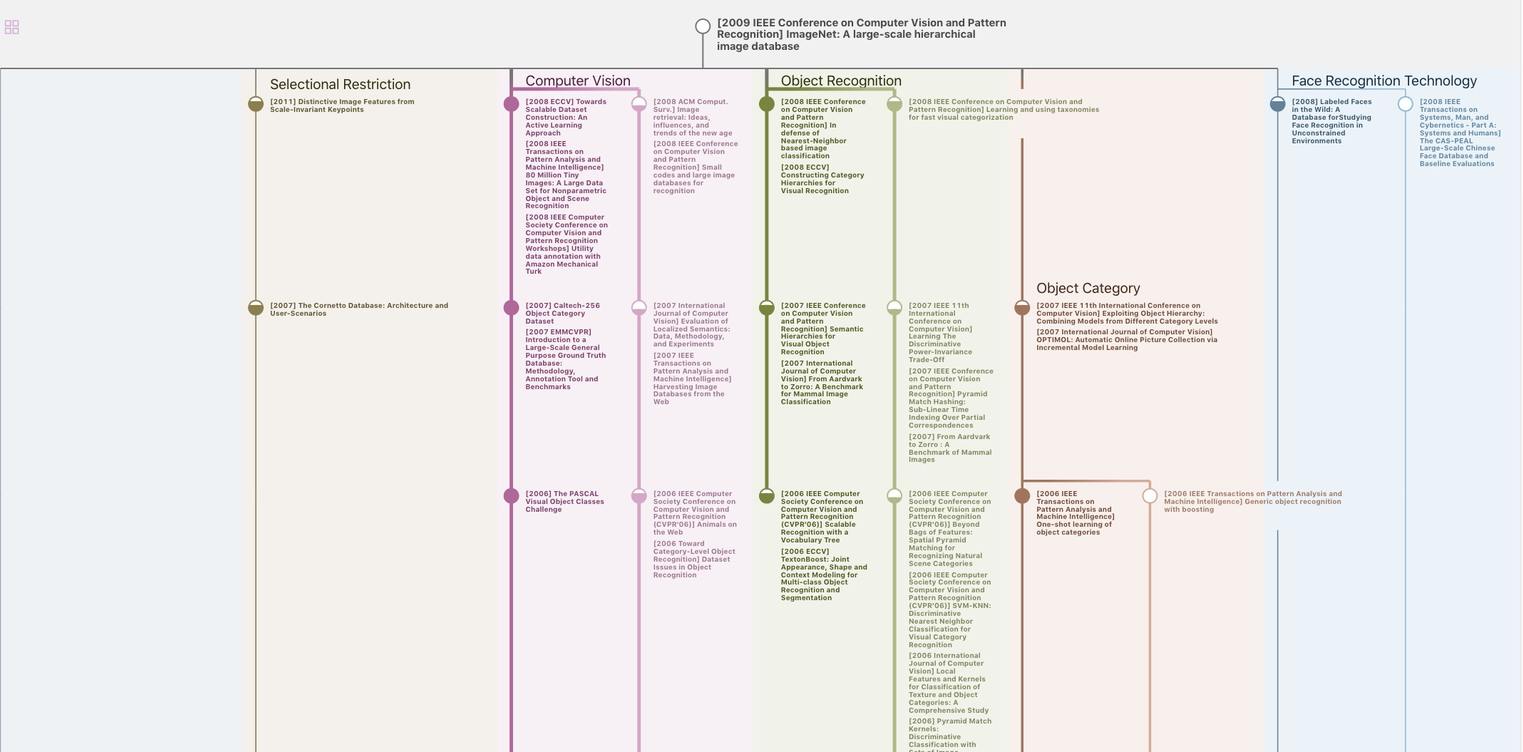
生成溯源树,研究论文发展脉络
Chat Paper
正在生成论文摘要