Deep learning mutation prediction enables early stage lung cancer detection in liquid biopsy
international conference on learning representations(2018)
摘要
Somatic cancer mutation detection at ultra-low variant allele frequencies (VAFs) is an unmet challenge that is intractable with current state-of-the-art mutation calling methods. Specifically, the limit of VAF detection is closely related to the depth of coverage, due to the requirement of multiple supporting reads in extant methods, precluding the detection of mutations at VAFs that are orders of magnitude lower than the depth of coverage. Nevertheless, the ability to detect cancer-associated mutations in ultra low VAFs is a fundamental requirement for low-tumor burden cancer diagnostics applications such as early detection, monitoring, and therapy nomination using liquid biopsy methods (cell-free DNA). Here we defined a spatial representation of sequencing information adapted for convolutional architecture that enables variant detection at VAFs, in a manner independent of the depth of sequencing. This method enables the detection of cancer mutations even in VAFs as low as 10x-4^, u003e2 orders of magnitude below the current state-of-the-art. We validated our method on both simulated plasma and on clinical cfDNA plasma samples from cancer patients and non-cancer controls. This method introduces a new domain within bioinformatics and personalized medicine – somatic whole genome mutation calling for liquid biopsy.
更多查看译文
AI 理解论文
溯源树
样例
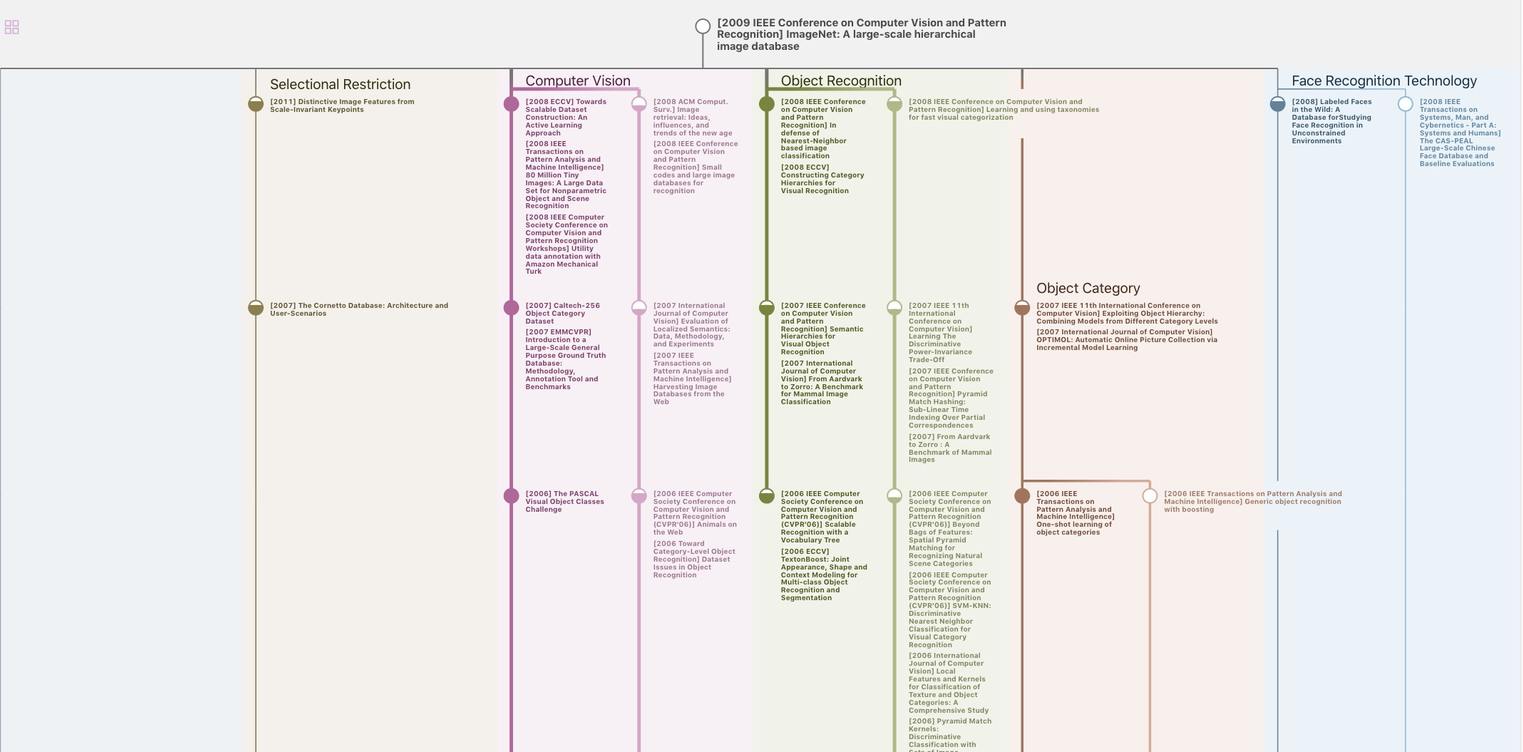
生成溯源树,研究论文发展脉络
Chat Paper
正在生成论文摘要