A scalable estimate of the extra-sample prediction error via approximate leave-one-out
arXiv: Methodology(2018)
摘要
We propose a scalable closed-form formula ($text{ALO}_{lambda}$) to estimate the extra-sample prediction error of regularized estimators. Our approach employs existing heuristic arguments to approximate the leave-one-out perturbations. We theoretically prove the accuracy of $text{ALO}_{lambda}$ in the high-dimensional setting where the number of predictors is proportional to the number of observations. We show how this approach can be applied to popular non-differentiable regularizers, such as LASSO, and compare its results with other popular risk estimation techniques, such as Steinu0027s unbiased risk estimate (SURE). Our theoretical findings are illustrated using simulations and real recordings from spatially sensitive neurons (grid cells) in the medial entorhinal cortex of a rat.
更多查看译文
AI 理解论文
溯源树
样例
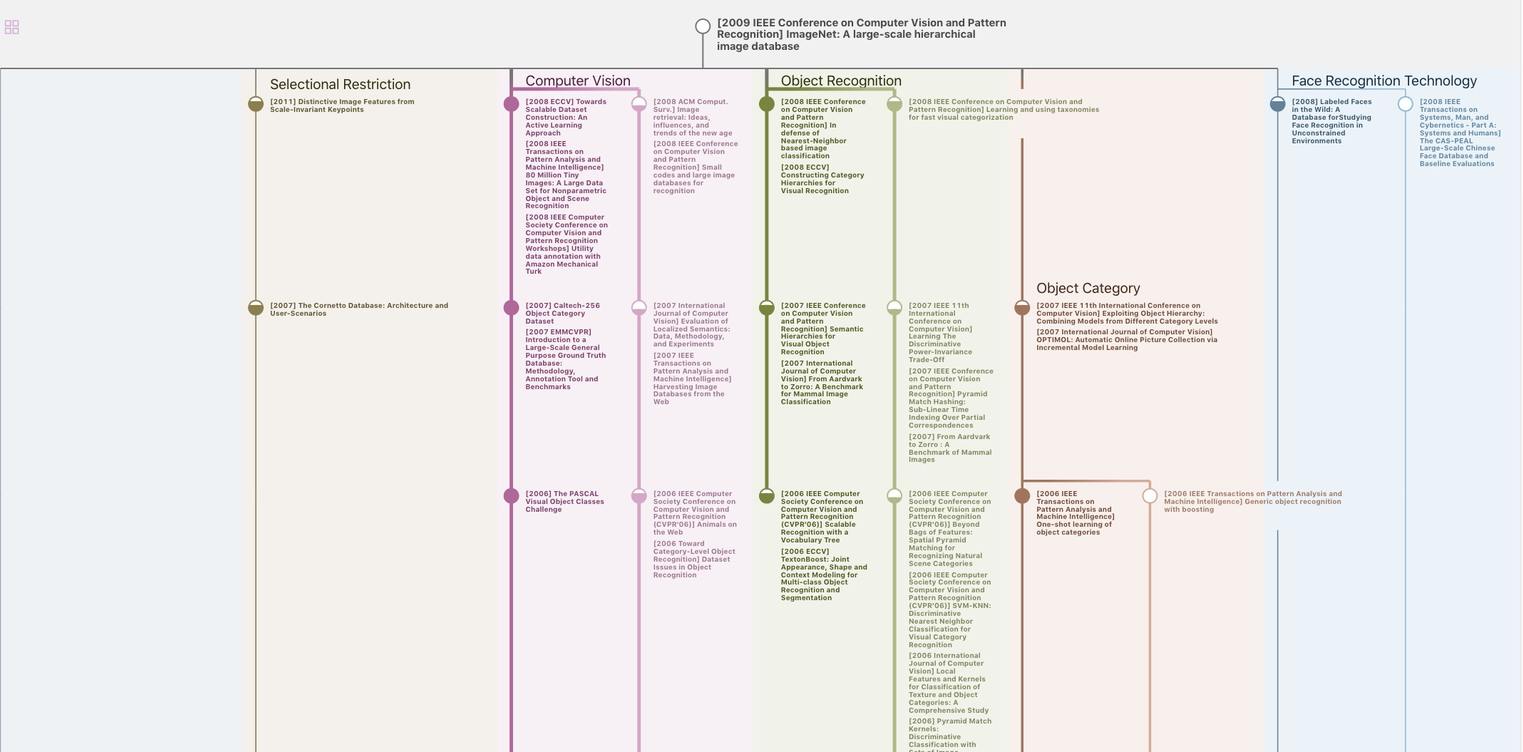
生成溯源树,研究论文发展脉络
Chat Paper
正在生成论文摘要