A FKSVM Model Based on Fisher Criterion for Text Classification
2017 10TH INTERNATIONAL SYMPOSIUM ON COMPUTATIONAL INTELLIGENCE AND DESIGN (ISCID), VOL 2(2017)
摘要
Text classification is the process of automatically assigning a given document to its previous category. It is widely used in artificial intelligence and natural language processing. In this paper, we propose a new classification model named FKSVM in order to improve the accuracy of text classification. According to the model, firstly, we use the TF-IDF algorithm to calculate the weight of features, and then the feature vectors are sorted according to the value of T calculated by Anova and T-test. Secondly, the number of features that can produce the optimal classification performance is selected according to the Fisher criterion function. Finally, the KSVM classifier is used to classify which is the combination of SVM and KNN. The algorithm calculates the distance between the sample and the optimal hyperplane of SVM. If the distance is larger than the pre given threshold, the sample will be classified by SVM. Otherwise, the KNN classifier will be used. The experiment results indicate that the average accuracy of FKSVM model is up to 88.83%, while that of KNN is 83.20%, SVM is 84.73% and KSVM is 85.56%.
更多查看译文
关键词
Text classification, Fisher criterion, KNN, SVM
AI 理解论文
溯源树
样例
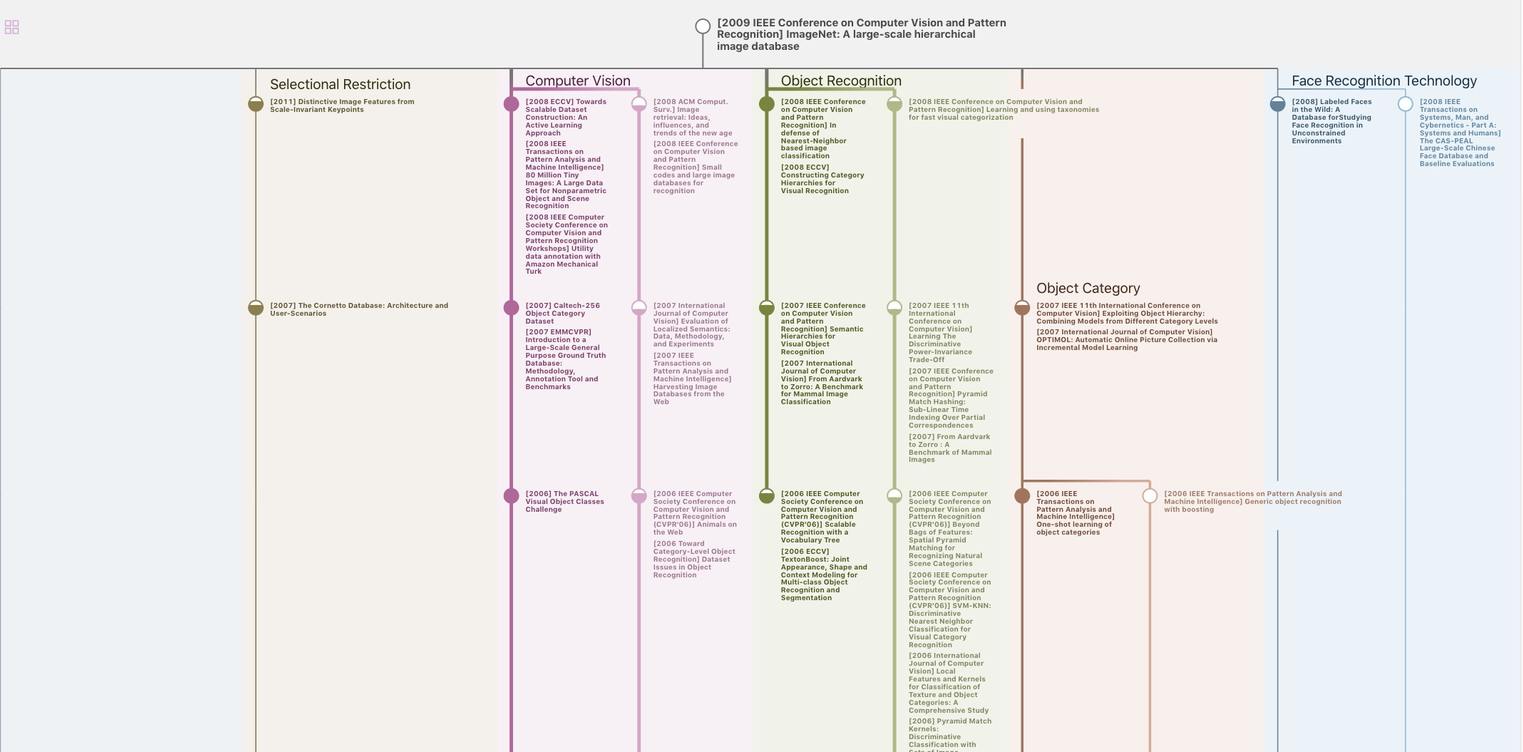
生成溯源树,研究论文发展脉络
Chat Paper
正在生成论文摘要