An efficient automatic multiple objectives optimization feature selection strategy for internet text classification
International Journal of Machine Learning and Cybernetics(2018)
摘要
Research on feature selection in text classification is usually limited to propose various techniques to select a set of features with highest scores based on different metrics. The selected features are usually determined by using a separate validation dataset with a fixed threshold. Obviously, it may not generalize well to new data as the best number for selected features is various on different datasets. In this paper, we first conduct a deep analysis, and find that simply extracting the features based on the score calculated by a metric may not always be the best strategy as it may turn many documents into zero length, which make them not suitable for training. We then model the feature selection process as a multiple objectives optimization problem to gain the best number of selected features rationally and automatically. In addition, as the optimization process costs a lot of resources, we design a parallel algorithm to improve the running time using dynamic programming. Extensive experiments are performed on several popular datasets, and the results indicate that our proposed approach is effective and feasible.
更多查看译文
关键词
Text Categorization,Separate Validation Dataset,Cost Optimization Process,Multiple Objective Optimization Problems,Negative Deviation Variables
AI 理解论文
溯源树
样例
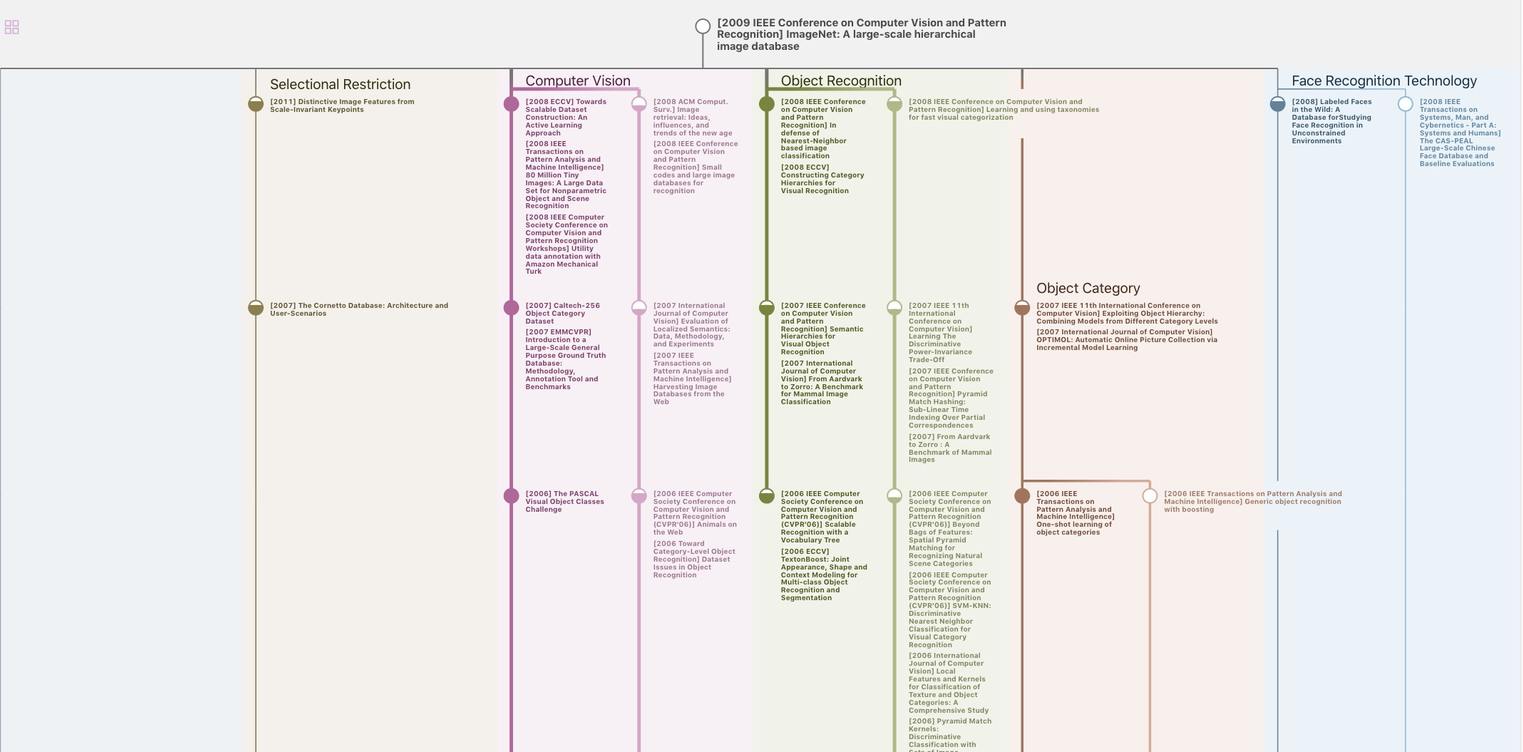
生成溯源树,研究论文发展脉络
Chat Paper
正在生成论文摘要