A New Bandwidth Selection Criterion for Analyzing Hyperspectral Data Using SVDD
arXiv: Applications(2018)
摘要
This paper presents a method for hyperspectral image classification using support vector data description (SVDD) with Gaussian kernel function. SVDD has been a popular machine-learning technique for single-class classification, but selecting the proper Gaussian kernel bandwidth to achieve the best classification performance is always a challenging problem. In this paper, we propose a new automatic, unsupervised Gaussian kernel bandwidth selection approach. A multi-class SVDD classification scheme is designed based on the proposed approach and applied to hyperspectral image data. The performance of the multi-class SVDD classification scheme is evaluated on three frequently used hyperspectral data sets and preliminary results show our proposed method can achieve better performance than published results on these data sets.
更多查看译文
AI 理解论文
溯源树
样例
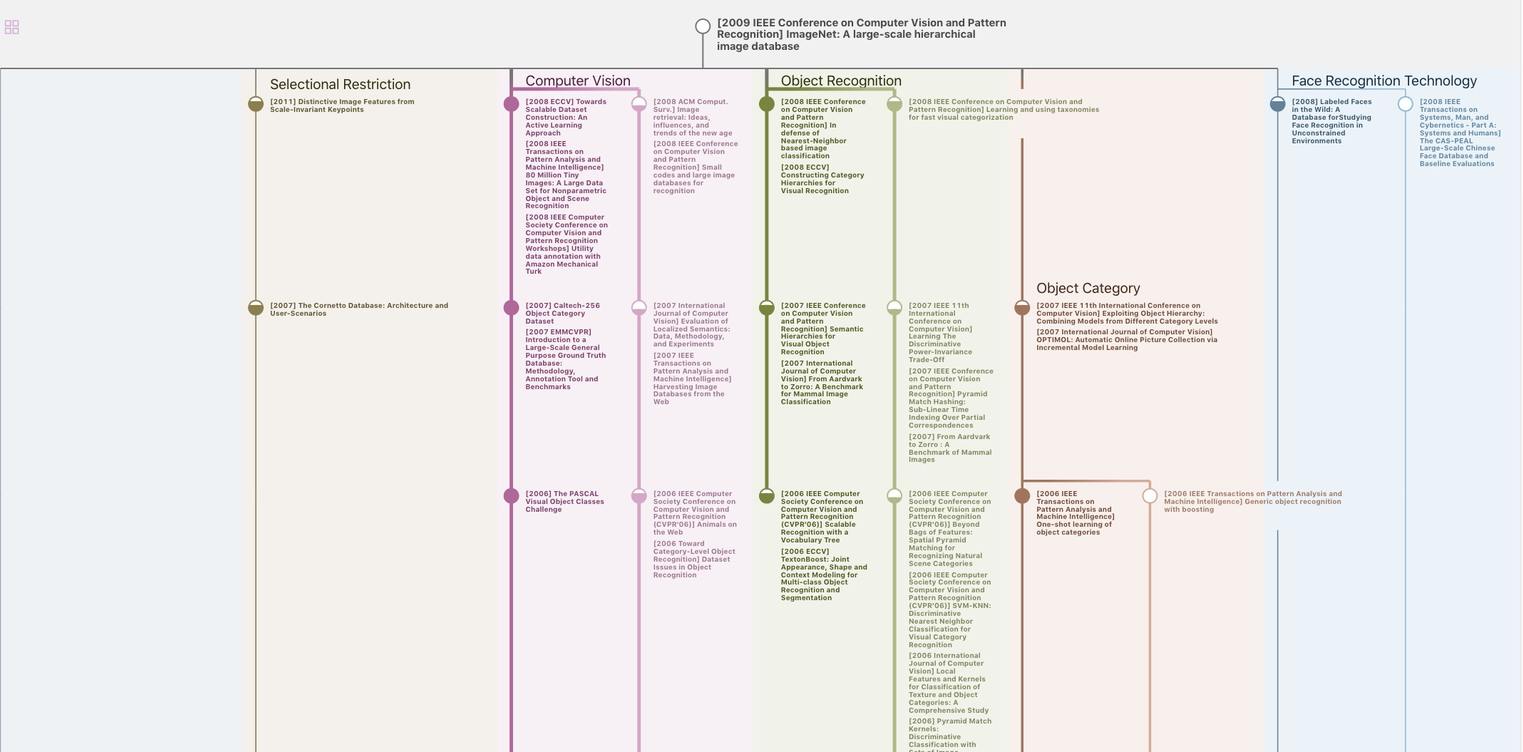
生成溯源树,研究论文发展脉络
Chat Paper
正在生成论文摘要