Imputation of posterior linkage probability relations reveals a significant influence of structural 3D constraints on linkage disequilibrium
bioRxiv(2018)
摘要
Genetic association studies have become increasingly important in unraveling the genetics of diseases or complex traits. Despite their value for modern genetics, conflicting conclusions often arise through the difficulty of confirming and replicating experimental results. We argue that this problem is largely based on the application of statistical relation measures that are not appropriate for genomic data analysis and demonstrate that the standard measures used for Genome-wide association studies or genomics linkage analysis bear a statistic bias. This may come from the violation of underlying assumptions (such as independence or stationarity) as well as from other conceptual limitations in the measures or relations, such as missing invariance with respect to coding or the inability to reflect latent factors. Attempts to introduce unbiased relation measures that avoid these limitations are usually computationally expensive and do not scale for large data sizes being typical for genomics applications. To tackle these problems, we propose a straightforwardly computable relation measure called Linkage Probability (LP). This measure provides the posterior probability of a relation between two categorical data sets and considers potential biases from latent variables. We compare several aspects of popular relation measures through an illustrative example and human genomics data. We demonstrate that the application of LP to the analysis of Single Nucleotide Polymorphisms (SNP) reveals latent 3D steric effects within 1D SNP data, that approximate to chromatin loops captured by high resolution Hi-C maps.
更多查看译文
关键词
Relation measure,Genome Wide Association Studies,Multiscale processesi,Information criteria,Numerical methods
AI 理解论文
溯源树
样例
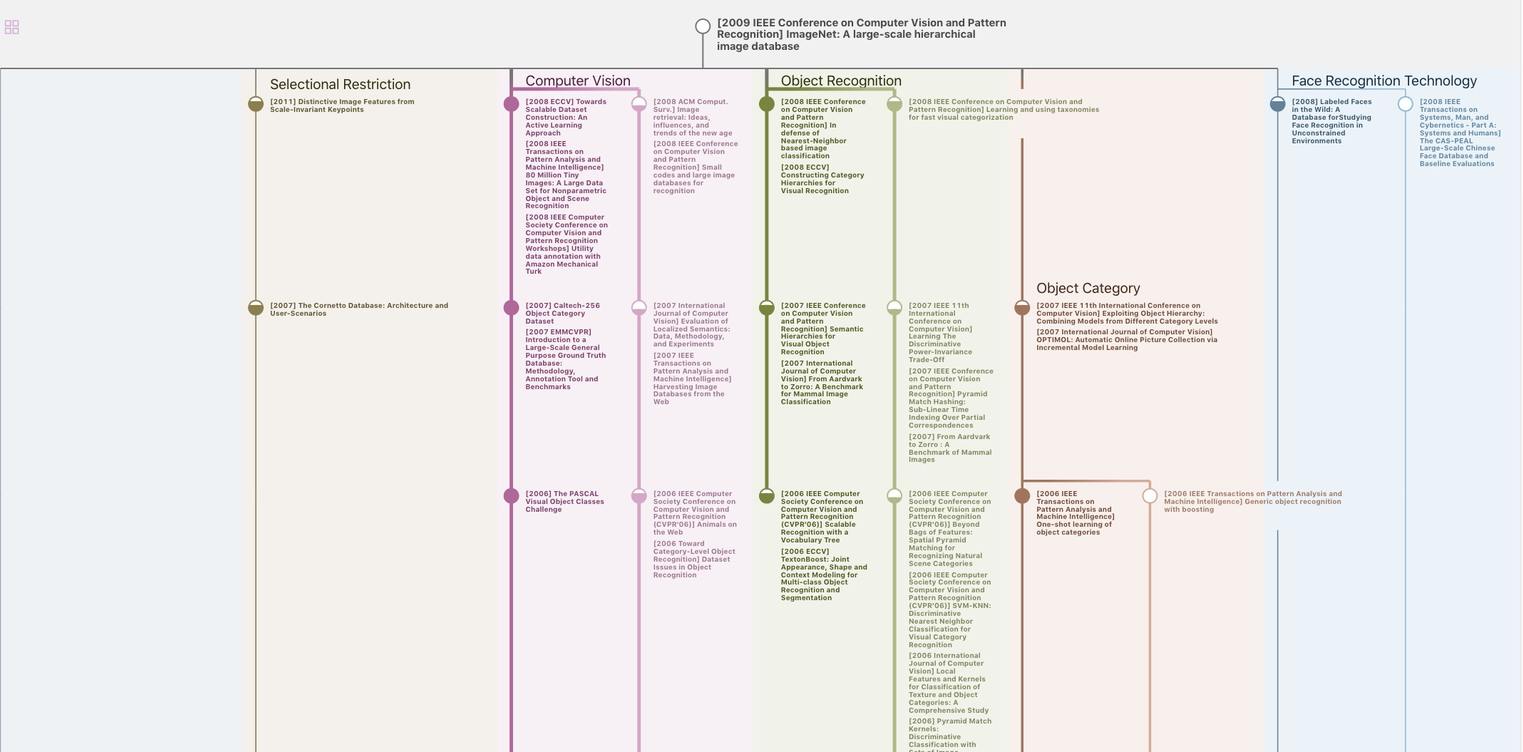
生成溯源树,研究论文发展脉络
Chat Paper
正在生成论文摘要