Low-Rank Solution Methods for Stochastic Eigenvalue Problems
SIAM JOURNAL ON SCIENTIFIC COMPUTING(2019)
摘要
We study efficient solution methods for stochastic eigenvalue problems arising from discretization of self-adjoint PDEs with random data, where the underlying operators depend linearly on the random parameters. With the stochastic Galerkin approach, the solutions are represented as generalized polynomial chaos expansions. When these solutions can be approximated well by low-rank objects, we introduce a low-rank variant of the inverse subspace iteration algorithm for computing one or several minimal eigenvalues and corresponding eigenvectors of parameter-dependent matrices. In the algorithm, the iterates are approximated by low-rank matrices, which leads to significant cost savings. The algorithm is tested on two benchmark problems: a stochastic diffusion problem with some poorly separated eigenvalues and an operator derived from a discrete stochastic Stokes problem whose minimal eigenvalue is related to the inf-sup stability constant. Numerical experiments show that the low-rank algorithm produces accurate solutions compared to the Monte Carlo method, and it uses much less computational time than the original algorithm without low-rank approximation.
更多查看译文
关键词
stochastic eigenvalue problem,inverse subspace iteration,low-rank approximation
AI 理解论文
溯源树
样例
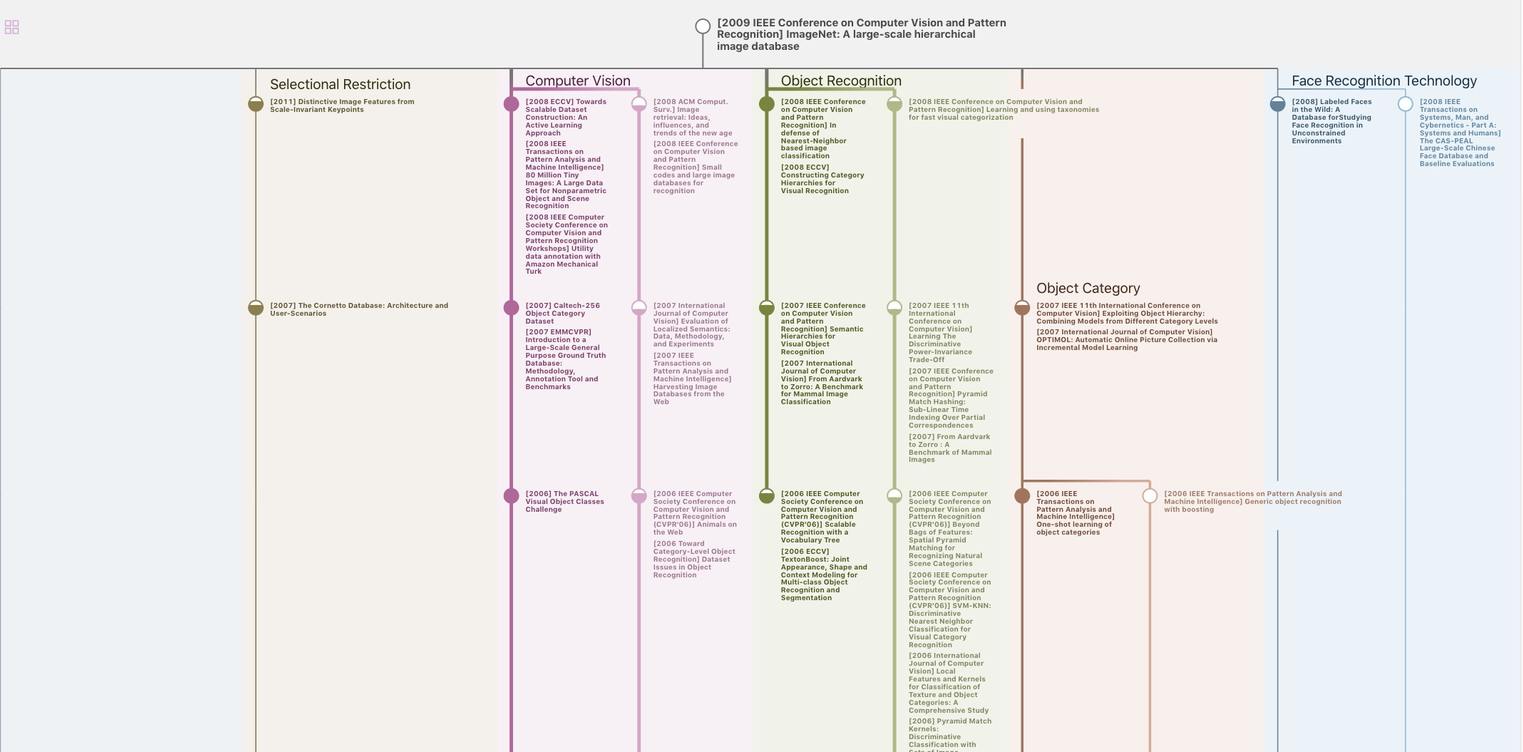
生成溯源树,研究论文发展脉络
Chat Paper
正在生成论文摘要